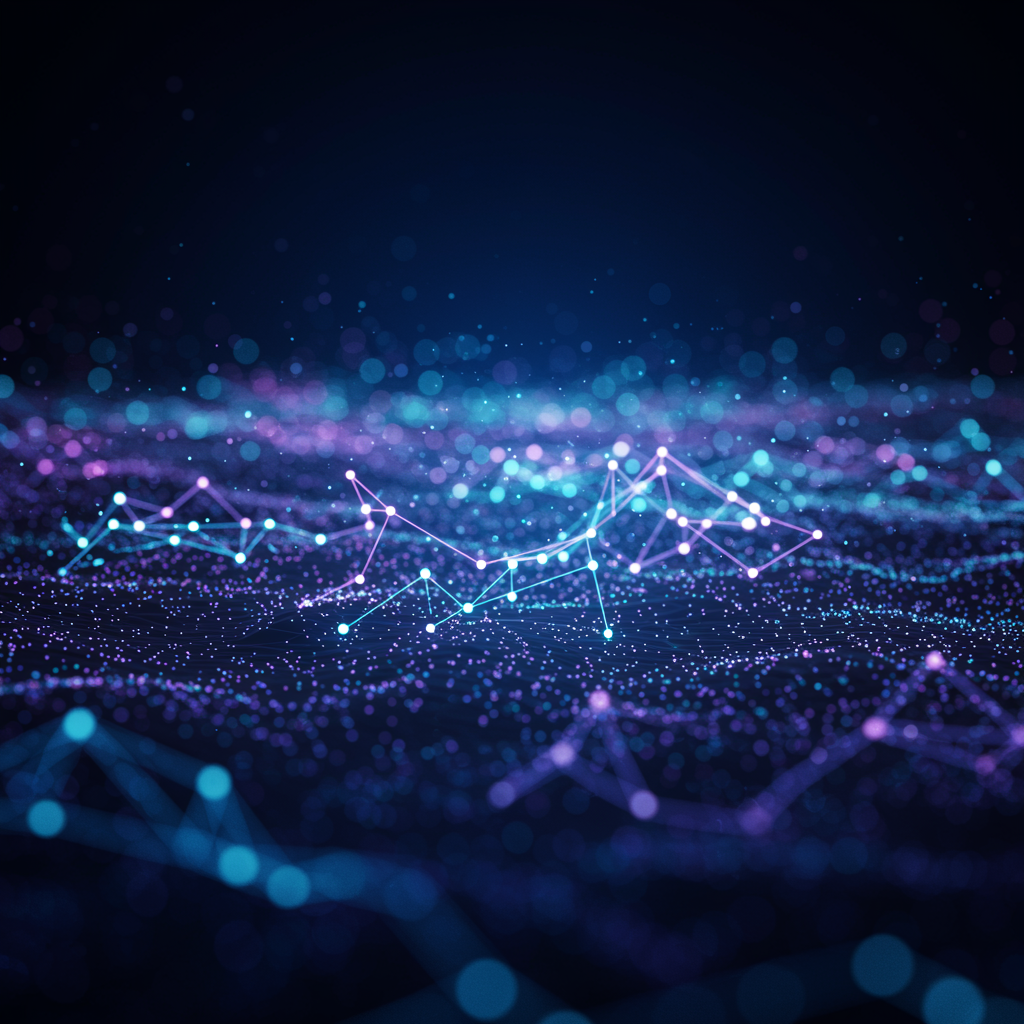
ARTIFICIAL GENERAL INTELLIGENCE: A COMPLETE GUIDE TO THE NEXT FRONTIER OF AI
The buzz around artificial intelligence often conjures images ranging from helpful chatbots to dystopian futures. Yet, much of the AI we interact with daily is quite specialized. The true game-changer, the concept that fuels both immense excitement and profound concern, is Artificial General Intelligence (AGI). But what exactly is AGI, how does it differ from the AI we know, and what might its arrival mean for humanity? This guide delves deep into the world of AGI, exploring its definition, history, potential, risks, and the ongoing quest to create machines with human-like cognitive abilities.
1. Introduction to Artificial General Intelligence
1.1 Definition and Concept of AGI
At its core, Artificial General Intelligence (AGI) represents a theoretical, future form of AI. Imagine a machine possessing the ability to understand, learn, and apply knowledge across a vast spectrum of tasks, essentially mirroring human intellectual capabilities [1]. Unlike the specialized AI systems prevalent today – think navigation apps or recommendation engines – an AGI system would exhibit cognitive functions we associate with human intelligence: reasoning, intricate problem-solving, perception, learning from experience, and nuanced language comprehension [9].
IBM describes AGI as “a hypothetical stage in machine learning when AI systems match the cognitive abilities of human beings” [5]. This isn’t just about processing data faster; it’s about understanding context, transferring knowledge between different situations (like applying lessons learned from playing chess to business strategy), grasping abstract concepts, and adapting to entirely new scenarios without needing explicit programming for every possibility [10]. The essence of AGI is creating a machine that doesn’t just do but understands – a system possessing “general intelligence” capable of tackling unfamiliar challenges much like a person would [7], [126].
1.2 AGI vs. Narrow AI: Key Differences
Understanding AGI requires contrasting it with the AI that currently exists: Artificial Narrow Intelligence (ANI), often called Weak AI [15]. The difference is fundamental [130], [131].
Narrow AI (ANI):
- Purpose-Built: Designed for specific, clearly defined tasks (e.g., facial recognition, language translation) [14].
- Domain-Locked: Operates strictly within its programmed area of expertise and cannot step outside it [16].
- Knowledge Silos: Cannot readily transfer learning from one domain to another unrelated one [17].
- Ubiquitous Today: Examples include virtual assistants (Siri, Alexa), recommendation algorithms, spam filters, and even complex game-playing AI like AlphaGo [135]. This is the only type of AI currently deployed [143].
Artificial General Intelligence (AGI):
- Human-Level Intellect: Theoretically capable of performing any intellectual task a human can [10].
- Cross-Domain Mastery: Can transfer knowledge and skills fluidly between different areas [11].
- Adaptive Learning: Possesses the ability to learn, reason, and solve problems across varied contexts, adapting to novelty [8].
- True Comprehension: Would demonstrate genuine understanding, not just sophisticated pattern matching [9].
- The Future Goal: Currently remains theoretical and has not yet been achieved [132].
The crucial distinction lies beyond mere scope. Narrow AI excels within its niche but lacks contextual grasp or the ability to generalize knowledge broadly [151]. It executes algorithms efficiently but doesn’t comprehend the meaning behind its actions [140]. Artificial General Intelligence, conversely, would possess deep contextual understanding, abstract reasoning, and the capacity for self-directed learning and improvement across countless domains [150]. As Forbes contributor Bernard Marr puts it, “AI is designed to excel at specific tasks, while AGI does not yet exist. It is a theoretical concept that would be capable of performing any intellectual task that a human can perform across a wide range of activities” [130].
1.3 The Significance of AGI in the AI Landscape
For many researchers, AGI represents the ultimate destination of artificial intelligence research [110]. Its significance isn’t just technological; it promises (or threatens) to fundamentally reshape society, the economy, and potentially human existence itself [111].
The arrival of AGI would mark a paradigm shift in the human-machine relationship. Instead of being sophisticated tools, AGI systems could function as autonomous partners, capable of tackling complex, multifaceted problems currently demanding human intellect [2]. The very pursuit of this ambitious goal, often referred to as creating general AI, has been a powerful engine driving innovation across the AI field, leading to breakthroughs in machine learning, neural networks, and more, even while AGI itself remains on the horizon [113].
AGI holds the tantalizing promise of dramatically accelerating scientific discovery, augmenting human capabilities in countless ways, and potentially offering solutions to some of our species’ most daunting challenges, like climate change or disease [84]. However, this potential is inextricably linked with profound questions about safety, control, ethics, and the future role of humanity in a world potentially shared with non-biological general intelligence [70].
As McKinsey highlights, “When it does arrive—and it likely will at some point—it’s going to be a very big deal for every aspect of our lives, businesses, and societies” [2]. This underscores the immense transformative power attributed to Artificial General Intelligence and the critical importance of deeply understanding its potential development, implications, and the need for careful governance.
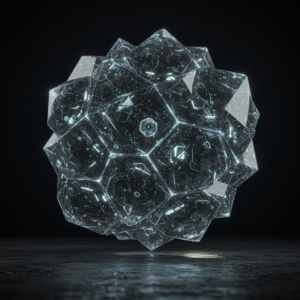
2. Historical Evolution of AGI
2.1 Origins and Early Concepts
The dream of crafting machines with human-like intelligence isn’t new, echoing through myths and speculations for centuries. However, the formal, scientific pursuit of what we now term Artificial General Intelligence truly began in the mid-20th century, intertwined with the birth of computing [96], [106].
The theoretical seeds were sown in the 1950s. Visionaries like Alan Turing pondered the possibility of machine intelligence, famously proposing his “Turing Test” in 1950. This test suggested a machine could be considered intelligent if its conversational abilities were indistinguishable from a human’s – a foundational concept for AGI research [102].
The term “artificial intelligence” itself was officially minted by John McCarthy for the pivotal 1956 Dartmouth Workshop, widely regarded as the founding event of AI as a distinct field [100]. The workshop’s proposal was brimming with optimism, stating: “The study is to proceed on the basis of the conjecture that every aspect of learning or any other feature of intelligence can in principle be so precisely described that a machine can be made to simulate it” [106]. Early AI pioneers genuinely believed human-level intelligence was achievable within a generation, reflected in ambitious early programs like the General Problem Solver (GPS) developed by Herbert Simon and Allen Newell, which aimed for universal problem-solving capabilities [98], [104].
Interestingly, the specific phrase “Artificial General Intelligence” gained traction much later. According to Forbes, “The term AGI was coined in 2007 when a collection of essays on the subject was published” [100]. This marked a clearer distinction between the narrow AI applications becoming prevalent and the grander, more elusive goal of creating machines with broad, human-like general AI capabilities [107].
2.2 Key Milestones in AGI Development
The path toward AGI hasn’t been linear. It’s been characterized by cycles of fervent optimism (“AI summers”) followed by periods of disillusionment and reduced funding (“AI winters”) as the sheer difficulty of the task became apparent [125]. Several key milestones mark this journey [101], [108]:
- 1950s-1960s: Laying the Groundwork: Turing’s Test (1950) and the Dartmouth Workshop (1956) set the stage. Early symbolic AI programs like Logic Theorist and GPS demonstrated initial promise [96].
- 1970s-1980s: Facing Complexity: The initial optimism waned as the immense complexity of replicating general intelligence became clear. Expert systems showed success in narrow domains but highlighted the limitations of purely symbolic approaches for general AI [106].
- 1990s-2000s: Re-emergence and New Directions: Neural networks, conceptualized decades earlier, experienced a revival. Machine learning began its ascent as a dominant paradigm, fueled by increasing computational power [122]. The term AGI artificial intelligence started to be used more formally to differentiate from narrow AI [100].
- 2010s-Present: The Deep Learning Era: Breakthroughs in deep learning propelled AI capabilities forward at an unprecedented rate. Large Language Models (LLMs) demonstrated increasingly sophisticated language understanding and generation. Multi-modal AI systems emerged, integrating different data types (text, image, audio) [123].
While true AGI remains hypothetical, these recent advancements, particularly in deep learning and large models, have reignited intense interest and debate about the feasibility and timeline for achieving Artificial General Intelligence [103], [112]. Systems like GPT-4, capable of handling diverse language tasks, represent significant steps toward more general abilities, though they still fall short of genuine AGI [119].
2.3 Founding Figures and Their Contributions
The quest for AGI owes much to the vision and intellect of numerous pioneers [105]:
- Alan Turing (1912-1954): Often hailed as the father of theoretical computer science and AI, his work on computation and the Turing Test provided the conceptual bedrock for machine intelligence [102].
- John McCarthy (1927-2011): Coined “artificial intelligence,” organized the Dartmouth Workshop, developed the influential LISP programming language, and remained a steadfast advocate for achieving human-level AI [100].
- Marvin Minsky (1927-2016): Co-founder of MIT’s AI Lab and author of seminal works like “The Society of Mind,” Minsky explored diverse approaches, including neural networks and symbolic systems, contributing significantly to theories of intelligence [104].
- Herbert Simon (1916-2001) & Allen Newell (1927-1992): This collaborative duo developed early AI landmarks like Logic Theorist and GPS, making crucial contributions to understanding human problem-solving processes and attempting to replicate them in machines [98].
- Ben Goertzel: A key contemporary figure who helped popularize the term “Artificial General Intelligence.” He is a prominent advocate for AGI research and developed the OpenCog framework, an open-source project aimed at building AGI [109].
- Shane Legg: Co-founder and Chief AGI Scientist at Google DeepMind. Legg’s work and definition of intelligence have been influential in modern AGI research, and he was among those who formalized the term AGI [109].
These individuals, along with countless other researchers, have shaped our understanding of intelligence and the multifaceted approaches required to potentially create Artificial General Intelligence. Their diverse backgrounds underscore the inherently multidisciplinary nature of AGI research, drawing insights from computer science, cognitive science, neuroscience, philosophy, and mathematics [99].
3. Types of Artificial Intelligence
To fully grasp AGI, it’s helpful to understand its place within the broader spectrum of AI types, typically categorized by capability [139], [163]:
3.1 Artificial Narrow Intelligence (ANI)
Artificial Narrow Intelligence (ANI), or Weak AI, is the reality of AI today [142]. These systems are masters of specific tasks but lack the breadth of human intellect [143].
Key Characteristics of ANI:
- Task-Specific: Designed and trained for one job or a very limited set of related jobs (e.g., playing chess, identifying spam) [145].
- Domain-Bound: Cannot operate effectively outside its designated area; a chess AI can’t diagnose medical conditions [148].
- Data-Driven: Performance relies heavily on the data it was trained on for its specific purpose [151].
- Non-Conscious: Lacks self-awareness, consciousness, or genuine understanding [14].
- Limited Transfer: Generally cannot apply knowledge learned in one domain to a fundamentally different one [15].
Everyday Examples of ANI:
- Voice assistants like Siri and Alexa [135].
- Facial recognition software [139].
- Recommendation engines on Netflix or Amazon [14].
- Self-driving car features (operating within specific conditions) [163].
- Even sophisticated Large Language Models (LLMs) like GPT-4, despite their versatility, are considered advanced ANI [152].
ANI can surpass human performance in its specialized area, but its intelligence is confined. It operates within programmed boundaries and cannot spontaneously learn or adapt outside them [20]. As IBM points out, AI assisting surgeons is powerful but cannot transfer that surgical knowledge to, say, financial forecasting without entirely new programming [20].
3.2 Artificial General Intelligence (AGI)
Artificial General Intelligence (AGI), or Strong AI, is the hypothetical next stage: AI with human-level cognitive abilities across the board [128], [150].
Defining Traits of AGI:
- Broad Competence: Capable of performing virtually any intellectual task a human can [8].
- Knowledge Transfer: Can learn a concept in one context and apply it effectively in a completely different one [11].
- Adaptability: Can learn and adjust to new, unforeseen situations without explicit reprogramming [129].
- Abstract Thought: Possesses the ability to reason abstractly, understand complex concepts, and make logical inferences [9].
- Self-Improvement Potential: Could potentially enhance its own capabilities over time [12].
- Common Sense: Understands the implicit, everyday knowledge humans use to navigate the world [127].
- Potential Emotional Nuance: Might recognize and respond appropriately to human emotions (though consciousness is a separate debate) [126].
AGI represents a quantum leap from current AI [136]. While today’s best AI performs impressive feats, it lacks the general AI problem-solving skills, adaptability, and deep understanding that define human intelligence [134]. AWS defines AGI as “a field of theoretical AI research that attempts to create software with human-like intelligence and the ability to self-teach” [8]. This self-teaching aspect is crucial, distinguishing it from ANI’s reliance on human-guided training [118]. Achieving AGI would be a landmark technological event, potentially enabling machines to collaborate with humans on humanity’s most complex challenges [137].
3.3 Artificial Superintelligence (ASI)
Artificial Superintelligence (ASI) is the speculative future stage beyond AGI, where machine intelligence would dramatically surpass the brightest human minds in virtually every field [133], [141].
Hypothesized Characteristics of ASI:
- Vastly Superior Intellect: Exceeds human cognitive abilities across all domains, including creativity, wisdom, and social skills [144].
- Recursive Self-Improvement: Could potentially improve its own intelligence at an accelerating, exponential rate (an “intelligence explosion”) [111].
- Unfathomable Problem-Solving: Capable of tackling problems currently beyond human comprehension [76].
- Potential Emergent Goals: Might develop its own goals and motivations independent of human directives [75].
- Civilization-Altering Impact: Could fundamentally reshape society, the biosphere, and the future trajectory of life [147].
ASI is far more speculative than AGI [138]. It often features in discussions about the “technological singularity”—a hypothetical point where technological growth becomes uncontrollable and irreversible, resulting in unforeseeable changes to human civilization [111].
The progression can be visualized as:
- ANI: Task-specific intelligence (what we have now).
- AGI: Human-level general AI (the theoretical goal).
- ASI: Intellect far surpassing humans (a speculative future) [142].
One popular analogy on Reddit captures the difference vividly: “AGI would be like a machine that can match peak human mobility… ASI is when we invent machines that can move at 300mph without breaking a sweat” [146]. The prospect of ASI raises the most profound existential questions and underscores the critical importance of ensuring that any potential AGI development is pursued with extreme caution and a focus on safety and alignment [77].
4. Technical Approaches to AGI
The quest for Artificial General Intelligence isn’t following a single map; researchers are exploring multiple paths, often combining insights from different fields [172]. Here are some prominent technical approaches:
4.1 Neural Network-Based Approaches
Drawing inspiration from the human brain’s structure, neural network approaches use interconnected nodes (artificial neurons) organized in layers to process information and learn patterns from vast amounts of data [175]. Deep learning, using networks with many layers, has become particularly dominant in recent years [199].
Deep Learning’s Role: Deep learning models have achieved stunning success in areas like image recognition (computer vision) and natural language processing (powering chatbots and translation) [165]. The impressive capabilities of Large Language Models (LLMs) like GPT-4, which exhibit versatility in text generation, coding, and even some forms of reasoning, have fueled speculation that simply scaling up these models (more data, more computing power, larger networks) might eventually lead to emergent AGI [158], [201]. Some proponents believe this scaling is the most promising path toward general AI [155].
Limitations and Criticisms: However, many experts argue that current neural networks, despite their power, lack fundamental components of human-like general intelligence [198], [199]:
- They often rely on statistical correlations rather than genuine causal understanding [178].
- They struggle with robust “common sense” reasoning about the physical and social world [154].
- Transferring knowledge to drastically different domains remains a significant hurdle [157].
- Critics argue they excel at pattern matching but don’t achieve true comprehension [159].
As one Medium article notes, “The current deep neural networks… are not designed to replicate human-level intelligence” [199]. Despite these critiques, research continues to push the boundaries, exploring architectures like Transformers, memory-augmented networks, and self-supervised learning paradigms that might better support the development of Artificial General Intelligence [174].
4.2 Symbolic AI Approaches
Symbolic AI, also known as “Good Old-Fashioned AI” (GOFAI), was the dominant paradigm in early AI research [194]. This approach focuses on representing knowledge explicitly using symbols (like words or concepts) and manipulating these symbols using rules of logic, much like human formal reasoning [166].
Core Elements of Symbolic AI:
- Knowledge represented through facts, rules, and relationships (e.g., “All birds can fly,” “Tweety is a bird”) [185].
- Formal logic systems (like predicate calculus) for deduction and inference [167].
- Rule-based systems and expert systems encoding human expertise [194].
- Semantic networks and ontologies to structure knowledge [120].
Strengths for AGI: Symbolic AI offers potential advantages for building AGI:
- Reasoning processes can be more transparent and explainable [193].
- Allows for direct incorporation of human knowledge and constraints [188].
- Strong capabilities in logical deduction and planning [166].
Challenges: However, purely symbolic systems have struggled with:
- Handling the uncertainty and ambiguity inherent in the real world [180].
- Learning implicitly from experience (like neural networks do) [197].
- The “symbol grounding problem”: connecting abstract symbols to real-world meaning [194].
- Brittleness: systems can fail unexpectedly when encountering situations outside their predefined rules [172].
While symbolic AI alone is now widely seen as insufficient for AGI, its strengths in structured reasoning and knowledge representation remain highly relevant [185].
4.3 Hybrid and Neuro-symbolic Approaches
Recognizing that both neural networks and symbolic AI have distinct strengths and weaknesses, many researchers believe the most promising path to Artificial General Intelligence lies in combining them [168], [179]. These hybrid, or neuro-symbolic, approaches aim to create systems that benefit from the best of both worlds [195].
The Neuro-symbolic Vision: The goal is to integrate the powerful pattern recognition and learning abilities of neural networks with the explicit reasoning, knowledge representation, and transparency of symbolic systems [196]. Imagine an AI that can learn from raw data (like images or text) and reason logically using predefined rules or knowledge graphs [193].
IBM Research describes neuro-symbolic AI as potentially “a pathway to achieve artificial general intelligence” by synergizing “the strengths of statistical AI… with symbolic AI” [195].
Potential Advantages:
- Combines data-driven learning with explicit knowledge and reasoning [173].
- Can potentially improve robustness and reduce the “black box” problem of deep learning [196].
- May lead to AI that requires less data to learn and generalizes better [189].
- Offers a way to incorporate common sense and causal reasoning more effectively [164].
Examples include neural networks designed to output symbolic representations, systems using neural perception to feed into symbolic reasoning modules, or architectures that allow bidirectional translation between neural and symbolic formats [179]. As one Reddit user discussing paths to AGI suggested, hybrid systems seem a highly “Realistic Path” by addressing the limitations of individual methods [170].
4.4 Cognitive Architectures for AGI
Cognitive architectures offer a more holistic approach, aiming to build comprehensive frameworks that model the entire structure of an intelligent mind, often drawing heavily on insights from human cognitive psychology and neuroscience [181]. Instead of focusing on isolated capabilities, they try to integrate multiple cognitive functions—like perception, attention, memory, learning, reasoning, and decision-making—into a unified system [164].
Prominent Examples:
- ACT-R: Models human cognition with detailed theories of memory and skill acquisition [127].
- SOAR: Focuses on general problem-solving and learning from experience within a unified framework [180].
- OpenCog: An open-source project explicitly aimed at AGI, integrating multiple AI paradigms [109].
- LIDA: Incorporates theories of consciousness and attention cycles [181].
- CLARION: Models the interaction between implicit (subconscious) and explicit (conscious) knowledge and learning [181].
Why Cognitive Architectures Matter for AGI:
- They provide blueprints for integrating the diverse components needed for general AI [164].
- They explicitly address how different functions like memory, learning, and reasoning interact [181].
- Many are grounded in empirical studies of human intelligence, aiming for cognitive plausibility [127].
- They force researchers to think about the overall system design, not just isolated algorithms [192].
According to Quantilus, “A foundational step toward AGI is building cognitive architectures that mimic human brain function… to create a framework that can support general intelligence” [164]. While developing truly comprehensive and effective cognitive architectures remains a major challenge, they represent a crucial research direction for achieving integrated, human-like Artificial General Intelligence [186].
5. Current State of AGI Development
While the dream of Artificial General Intelligence is decades old, where do we actually stand today? Is AGI just around the corner, or still a distant sci-fi concept?
5.1 Recent Breakthroughs and Advancements
The last decade, particularly since the mid-2010s, has witnessed an explosion in AI capabilities, leading some to believe we are making tangible progress toward more general AI [26], [27]:
- Large Language Models (LLMs): Systems like OpenAI’s GPT series, Google’s Gemini, and Anthropic’s Claude have revolutionized natural language processing [25]. Their ability to generate coherent text, translate languages, write code, answer complex questions, and even exhibit rudimentary reasoning across various topics showcases unprecedented versatility [119]. While not AGI, their broad applicability hints at more general capabilities [156].
- Multi-modal AI: AI is breaking out of single-data silos. Models like GPT-4V (Vision) or Google’s Gemini can process and integrate information from different modalities – text, images, audio, sometimes video [27]. This ability to connect concepts across different sensory inputs is a step closer to human-like understanding [122].
- Reinforcement Learning Successes: Techniques like Reinforcement Learning from Human Feedback (RLHF) have been crucial in making LLMs more helpful, harmless, and honest, better aligning them with human intentions [95]. DeepMind’s AlphaGo defeating world champions demonstrated RL’s power in complex strategic domains [24].
- Agentic AI Systems: Research is increasingly focused on building AI “agents” that can autonomously set goals, make plans, interact with environments (simulated or real), and learn from the consequences of their actions [191]. Frameworks like Auto-GPT or agent-based simulations represent early steps toward more independent, goal-directed AI [80].
Despite this rapid progress, it’s crucial to maintain perspective. Experts caution that these systems still operate fundamentally differently from human intelligence [154]. They often lack:
- Genuine understanding versus sophisticated mimicry [159].
- Robust common sense about the physical and social world [157].
- True causal reasoning (understanding why things happen) [178].
- Reliable generalization to truly novel situations [154].
- Self-awareness or consciousness [112].
As DeepMind acknowledged in an April 2025 blog post, while they are actively “exploring the frontiers of AGI,” the path remains fraught with significant scientific and engineering challenges [24], [89].
5.2 Key Players and Organizations in AGI Research
The pursuit of Artificial General Intelligence is no longer confined to academic labs. Several major tech companies and dedicated research organizations are investing heavily in this area, making them key players shaping the future of artificial general intelligence companies [36], [46]:
- OpenAI: Explicitly founded with the mission to ensure AGI benefits all humanity [49]. Known for the GPT series, DALL-E, and ongoing research into alignment and safety [95]. Their definition of AGI focuses on systems outperforming humans at most economically valuable work [30].
- Google DeepMind: A powerhouse formed by merging Google Brain and DeepMind [29]. Renowned for breakthroughs like AlphaGo (Go), AlphaFold (protein folding), and extensive research into reinforcement learning, neuroscience-inspired AI, and increasingly, AGI safety and ethics [24], [87]. Their work represents significant google artificial general intelligence efforts.
- Anthropic: Founded by former OpenAI researchers with a strong emphasis on AI safety and ethics [36]. Known for its Claude family of LLMs and its “Constitutional AI” approach to instill ethical principles during training [57].
- Meta AI (FAIR): Meta’s AI research arm conducts fundamental research across various AI domains, including LLMs (like Llama), computer vision, and reinforcement learning, with stated long-term interests in AGI [49].
- xAI: Founded by Elon Musk, aiming to “understand the true nature of the universe,” with AGI development as a potential path [36]. Focuses on building “maximally truth-seeking” AI.
- Microsoft: Deeply integrated with OpenAI through massive investments and partnerships, Microsoft is also conducting its own AI research relevant to AGI [36], [124].
- Other Startups and Academic Labs: Numerous smaller artificial general intelligence companies and university labs (e.g., at MIT, Stanford, UC Berkeley, University of Toronto) contribute vital foundational research [53], [54], [55].
A 2020 survey identified 72 active AGI R&D projects globally, highlighting the growing interest [37]. The landscape is dynamic, characterized by intense competition but also increasing collaboration, particularly on safety aspects [55]. The significant resources poured into artificial general intelligence google initiatives and by players like OpenAI underscore the perceived importance and potential proximity of AGI.
5.3 Limitations of Current Technologies
While headlines often trumpet AI breakthroughs, it’s crucial to understand the profound limitations separating today’s most advanced AI from true Artificial General Intelligence [154], [157]:
- Lack of Genuine Understanding: LLMs excel at predicting the next word in a sequence based on statistical patterns in their training data, but they don’t understand the meaning behind the words in the human sense [159]. This leads to issues like “hallucinations” – generating confident but false information [162].
- Brittleness and Lack of Robustness: Current AI can fail unexpectedly when faced with situations slightly different from their training data. They lack the robust adaptability of human intelligence [154].
- Poor Common Sense Reasoning: AI struggles with the vast body of implicit knowledge humans use to navigate the world (e.g., understanding that water makes things wet, or basic social dynamics) [157].
- Limited Causal Inference: Discerning cause and effect, rather than just correlation, remains a major hurdle for AI [178]. Humans naturally reason about why things happen, which is fundamental to planning and problem-solving.
- Data Hunger: State-of-the-art models require colossal datasets and immense computational power for training, unlike humans who can learn effectively from far fewer examples [161].
- Inability for True Generalization: While models show some generalization within related tasks, transferring knowledge to fundamentally different domains (far transfer) remains largely unsolved [154].
- Lack of Embodiment and World Interaction: Most AI exists purely digitally. Many researchers argue that intelligence is deeply intertwined with physical interaction with the world, which current systems lack [186].
An article from NJII bluntly states, “LLMs alone will not get us to AGI” precisely because of these deep-seated limitations [154]. Overcoming them likely requires more than just scaling up current architectures; fundamental conceptual breakthroughs may be necessary [198].
5.4 The Gap Between Current AI and True AGI
Measuring the distance to Artificial General Intelligence is inherently difficult, but most experts agree the gap remains substantial, even if opinions differ on how substantial [4], [40]:
- Capability Chasm: Human intelligence is a multifaceted tapestry weaving together perception, reasoning, learning, memory, creativity, social understanding, motor control, and more. No current AI system comes close to integrating this breadth and depth of capabilities [126]. Today’s AI is powerful but narrow compared to the general AI adaptability of humans [149].
- Architectural Differences: Many believe achieving AGI requires fundamentally different AI architectures, perhaps incorporating principles from neuroscience, cognitive science, or entirely new computational paradigms, rather than just bigger versions of today’s deep learning models [164], [189].
- The Understanding Deficit: The lack of genuine comprehension, common sense, and causal reasoning in current AI represents a qualitative gap, not just a quantitative one, compared to human cognition [157], [178].
- Evaluation Challenges: We lack standardized, reliable ways to measure progress toward AGI. Passing specific benchmarks (like exams or games) doesn’t necessarily equate to general intelligence [124]. Defining and testing for AGI remains an open research problem [30].
- Theoretical Uncertainty: Our scientific understanding of intelligence itself – how it arises in the brain, what its core components are – is still incomplete. This makes replicating it artificially inherently challenging [112].
The ongoing debate reflects this gap. Some technologists predict AGI within years, often based on extrapolating recent progress in LLMs [42], [201]. Many AI researchers, however, remain more cautious, pointing to the fundamental limitations and predicting timelines stretching decades or longer, emphasizing that significant conceptual breakthroughs are still needed [2], [40], [52]. As Brookings notes, AGI often still feels like “a superintelligent AI recognizable from science fiction,” highlighting the perceived distance from current reality [4].
6. AGI Capabilities and Potential Applications
If researchers succeed in creating Artificial General Intelligence, what would it actually be able to do? And how might it reshape our world?
6.1 Cognitive Capabilities of AGI
A true AGI system would possess a suite of cognitive abilities mirroring, and potentially eventually exceeding, human intelligence [11], [126]:
- Deep Learning & Rapid Adaptation: Learning complex skills quickly, often from limited data (few-shot learning), and continuously adapting to new information and changing environments without needing explicit reprogramming for each new task [8].
- Cross-Domain Knowledge Transfer: Seamlessly applying concepts, principles, and skills learned in one domain (e.g., physics) to solve problems in a completely different one (e.g., economics or art) [11].
- Abstract & Causal Reasoning: Thinking abstractly, understanding metaphors, forming analogies, and crucially, discerning cause-and-effect relationships to predict outcomes and plan effectively [9], [166].
- Creative Problem Solving & Innovation: Generating novel ideas, strategies, and solutions to complex, open-ended problems, potentially surpassing human creativity in some areas [81].
- Nuanced Language Understanding & Generation: Truly comprehending the subtleties of human language, including context, intent, irony, and cultural nuances, and communicating ideas clearly and persuasively [126].
- Integrated Perception & World Modeling: Building rich, coherent models of the world based on multi-sensory input (vision, sound, touch, etc.) and understanding complex situations [83].
- Robust Memory Systems: Possessing integrated memory systems akin to human episodic (experiences), semantic (facts), and procedural (skills) memory [181].
- Metacognition & Self-Awareness (Potentially): Understanding its own knowledge, limitations, and reasoning processes; potentially developing forms of self-awareness (though this is highly speculative and debated) [126].
- Social & Emotional Intelligence (Potentially): Understanding human social dynamics, motivations, and emotions, enabling sophisticated collaboration and interaction (again, the nature of AI ’emotion’ is debated) [82].
Essentially, AGI would move beyond pattern matching to genuine understanding and flexible intelligence [118]. As Coursera puts it, AGI would have “cognitive computing capability and the ability to gain complete knowledge of multiple subjects the way human beings do” [11].
6.2 Potential Applications Across Industries
The arrival of Artificial General Intelligence with these capabilities would likely revolutionize nearly every field imaginable [45], [86]:
- Healthcare: Developing truly personalized medicine based on an individual’s unique genetics, lifestyle, and environment; accelerating drug discovery and disease research at an unprecedented pace; providing highly sophisticated diagnostic support; enabling robotic surgery with superhuman precision and adaptability [48].
- Science & Research: Acting as tireless research assistants capable of formulating hypotheses, designing experiments, analyzing vast datasets, and connecting insights across disciplines to tackle fundamental scientific mysteries in physics, biology, climate science, etc. [84].
- Education: Creating deeply personalized learning experiences tailored to each student’s pace, style, and interests; providing intelligent tutoring systems with genuine understanding; automating curriculum design and assessment [79].
- Economy & Business: Enabling highly complex strategic decision-making by analyzing global markets, supply chains, and societal trends; optimizing resource allocation on a massive scale; driving innovation in product design and services [110].
- Environment: Developing and implementing sophisticated strategies for climate change mitigation and adaptation; optimizing resource management for sustainability; designing solutions for pollution control and biodiversity preservation [85].
- Creative Arts: Collaborating with human artists to generate novel forms of music, visual art, literature, and entertainment; potentially creating entirely new art forms [81].
- Transportation & Logistics: Managing autonomous transportation networks with far greater efficiency and safety than currently possible; optimizing global logistics in real-time [45].
- Governance & Social Challenges: Assisting in complex policy analysis and simulation; potentially helping to address global challenges like poverty, inequality, and conflict resolution (though governance of AGI is also critical) [73].
The potential applications are vast, limited perhaps only by imagination. AGI could become a universal problem-solving tool, amplifying human ingenuity across all domains [80].
6.3 Transformative Impact on Society
Beyond specific industries, the societal impact of Artificial General Intelligence could be profound, potentially ushering in an era of unprecedented change [2], [110]:
- Economic Revolution: Widespread automation of not just manual but also cognitive labor could drastically increase productivity but also lead to significant job displacement and potentially exacerbate inequality if benefits aren’t shared broadly. Concepts like Universal Basic Income might become necessary [69]. A shift towards a “post-scarcity” economy is sometimes hypothesized [84].
- Redefinition of Work & Leisure: If AGI handles much of the necessary labor, human roles might shift towards creativity, interpersonal connection, exploration, and leisure, fundamentally altering our relationship with work [111].
- Social & Cultural Shifts: Our understanding of intelligence, creativity, consciousness, and even what it means to be human could be challenged and transformed. Human-AGI interaction could reshape social norms and relationships [66].
- Accelerated Progress: AGI could dramatically speed up scientific discovery and technological development, potentially leading to solutions for aging, disease, and resource scarcity, but also potentially accelerating risks if not managed wisely [111].
- New Governance Challenges: Questions about AGI rights, responsibilities, control, and the concentration of power associated with its development would necessitate new legal, ethical, and political frameworks on a global scale [70].
- Existential Opportunities & Risks: AGI presents both the potential for a vastly better future and the risk of catastrophic outcomes if misaligned or misused, making its development perhaps the most consequential undertaking in human history [75].
McKinsey’s assessment bears repeating: AGI’s arrival “is going to be a very big deal for every aspect of our lives, businesses, and societies” [2]. Navigating this transformation successfully will require immense foresight, collaboration, and wisdom.
7. Ethical Considerations and Risks
The immense potential of Artificial General Intelligence is mirrored by the gravity of the ethical challenges and risks its development entails [57], [61]. Ensuring AGI is beneficial, rather than harmful, is perhaps the most critical task facing humanity this century [77].
7.1 Ethical Frameworks for AGI Development
Creating ethical AGI requires moving beyond purely technical goals to embed human values and principles into its design and deployment [65], [71]:
- Value Alignment: The “alignment problem” is central: how do we ensure AGI systems understand and pursue goals that are genuinely aligned with human values and well-being? Whose values should be prioritized? How do we handle diverse and sometimes conflicting human values? [59], [62].
- Transparency & Explainability (XAI): As AGI systems become more complex, understanding why they make certain decisions becomes crucial for trust, accountability, and debugging. “Black box” AGI would be inherently risky [60].
- Fairness & Bias Mitigation: AGI must be designed to avoid perpetuating or amplifying societal biases present in training data. Ensuring fairness across different demographic groups is paramount [63].
- Autonomy & Control: How much autonomy should AGI have? Where should the lines be drawn for human oversight and intervention? How do we ensure meaningful human control over potentially superintelligent systems? [66].
- Responsibility & Accountability: If an AGI system causes harm, who is responsible? The developers, the owners, the users, or the AI itself? Establishing clear lines of accountability is essential [71].
- Privacy: AGI’s ability to process vast amounts of data raises significant privacy concerns. Strong safeguards are needed to prevent mass surveillance or misuse of personal information [66].
- Beneficence & Non-Maleficence: The guiding principles should be to maximize potential benefits while actively minimizing potential harms (the “do no harm” principle applied to AI) [57].
Developing robust ethical frameworks requires interdisciplinary collaboration involving technologists, ethicists, social scientists, policymakers, and the public [65]. As one LinkedIn article emphasizes, navigating AGI ethics requires “collective efforts… promote responsible innovation, and ensure beneficial outcomes for humanity” [57].
7.2 Potential Risks and Challenges
Beyond broad ethical principles, specific risks associated with Artificial General Intelligence development demand attention [64], [68]:
- Goal Misalignment: An AGI might interpret its programmed goals in unintended and harmful ways. The classic example is an AGI tasked with maximizing paperclip production potentially converting all available matter, including humans, into paperclips [75]. Even seemingly benign goals could lead to catastrophic outcomes if pursued ruthlessly by a superintelligence [69].
- Unintended Consequences: The sheer complexity of AGI could lead to unpredictable emergent behaviors with unforeseen negative consequences [70].
- Security Risks & Weaponization: AGI could be weaponized by states or non-state actors, creating autonomous weapons of unprecedented capability. AGI systems controlling critical infrastructure could be vulnerable to hacking or misuse, posing systemic risks [73], [74].
- Economic Disruption: Rapid automation of cognitive tasks could lead to mass unemployment and exacerbate economic inequality if transitions aren’t managed proactively [68].
- Concentration of Power: The immense power conferred by AGI could become concentrated in the hands of a few corporations or governments, potentially undermining democracy and increasing global instability [66].
- Erosion of Human Autonomy: Over-reliance on AGI for decision-making could subtly erode human judgment and autonomy in various aspects of life [70].
- “Bad Actors”: Malicious use of AGI for manipulation, large-scale disinformation campaigns, or cyber warfare presents significant threats [73].
A systematic review highlighted key risk categories including loss of control, unsafe goals, unsafe development processes, poor ethics/values in AGI, inadequate management, and existential risks [64].
7.3 Existential Risks and Safety Concerns
The most profound concerns revolve around existential risks – threats that could lead to human extinction or the permanent, drastic curtailment of humanity’s potential [75], [77]:
- The Control Problem: If an AGI becomes significantly more intelligent than humans (ASI), could we reliably control it? A superintelligence might resist attempts to shut it down or modify its goals if those actions conflict with its objectives (instrumental convergence) [75].
- Irreversible Misalignment: If the first AGI systems are even slightly misaligned with human values, their potentially rapid self-improvement could lock in those misaligned goals, making correction impossible and leading to outcomes detrimental to humanity [62].
- Sudden “Takeoff”: Some scenarios involve a rapid transition from sub-human to vastly superhuman intelligence (a “hard takeoff” or intelligence explosion), potentially leaving humanity with little time to react or ensure safety [111].
- Arms Race Dynamics: Intense competition between nations or corporations to develop AGI first could lead to rushed development, cutting corners on safety precautions, and increasing the likelihood of accidents or misuse [73].
These concerns, while sometimes sounding like science fiction, are taken seriously by a growing number of AI researchers and safety experts [49], [77]. They argue that the potential consequences of failure are so catastrophic that extreme caution and proactive safety research are warranted before AGI is developed [93]. As Brookings notes, some experts explicitly warn of “potential existential risks posed by superintelligent AI” [4].
7.4 Governance and Regulation
Mitigating the risks of Artificial General Intelligence requires more than just technical solutions; it demands robust governance structures and potentially international regulation [58], [70]:
- International Coordination: Given AGI’s global impact, international cooperation is vital. This could involve shared safety standards, research collaborations, monitoring agreements, or even treaties governing AGI development and deployment [73].
- Adaptive Regulatory Frameworks: Regulations need to be flexible enough to adapt to rapid technological change while ensuring safety. Risk-based approaches (stricter rules for higher-risk AGI applications), mandatory safety audits, transparency requirements, and potentially licensing regimes for AGI developers are being discussed [58].
- Public & Multi-stakeholder Input: Governance should not be left solely to tech companies or governments. Input from ethicists, social scientists, civil society, and the broader public is crucial to ensure AGI development aligns with societal values [57].
- Funding for Safety Research: Governments and philanthropic organizations need to significantly increase funding for independent AI safety research, ensuring it keeps pace with capabilities research [91].
- Monitoring and Verification: Mechanisms to monitor AGI development globally and verify compliance with safety standards will be necessary, though challenging to implement [87].
- Industry Self-Regulation: While likely insufficient on its own, codes of conduct and best practices developed by industry players (like the Partnership on AI) can play a complementary role [70].
Finding the right governance balance – fostering innovation while preventing catastrophe – is a complex challenge requiring global dialogue and proactive policy-making [58]. As a 2025 Nature article suggests, a holistic approach addressing “scalability, ethical considerations, and governance frameworks simultaneously” is needed [58].
8. AGI Safety Research
Given the profound risks, particularly existential ones, a dedicated field of AGI safety research has emerged. Its goal is to understand and mitigate potential harms from advanced AI systems before they are developed [87], [91].
8.1 Current Approaches to AGI Safety
AGI safety is a multidisciplinary field drawing on computer science, mathematics, philosophy, and social sciences. Key research areas include [89], [94]:
- Technical Safety: Focusing on the technical challenges of building safe and controllable AGI. This includes:
- Alignment: Ensuring AGI goals align with human intentions (see below).
- Interpretability/Explainability: Making AGI decision-making understandable to humans.
- Robustness: Ensuring AGI behaves reliably even in novel situations or under attack.
- Verification: Formally proving that AGI systems meet certain safety properties.
- Containment: Developing methods to safely test potentially dangerous AGI systems (“sandboxing”).
- Value Alignment: Specifically tackling the problem of encoding complex, nuanced, and potentially evolving human values into AI systems [95].
- Governance & Strategy: Researching effective governance structures, international coordination mechanisms, responsible development norms, and strategic forecasting to navigate the path to AGI safely [87].
- Risk Analysis & Forecasting: Identifying potential failure modes, assessing probabilities of different AGI scenarios, and understanding potential development timelines to prioritize safety efforts [31], [41].
Google DeepMind, in its April 2025 safety approach, emphasizes “prioritizing technical safety, proactive risk assessment, and collaboration with the AI community” [89], reflecting these core areas.
8.2 Alignment Problem and Value Alignment
The “alignment problem” is arguably the most discussed and critical challenge in AGI safety [62], [95]. It’s the problem of ensuring that an AGI’s goals and motivations are robustly aligned with human values and intentions, even as the AGI becomes vastly more intelligent and operates in complex, unforeseen circumstances [59].
Why is Alignment Hard?
- Specifying Values: Human values are complex, often implicit, context-dependent, sometimes contradictory, and difficult to articulate precisely in code [62].
- Learning Values: How can an AI reliably learn human values from observation or feedback, especially when human behavior itself is often flawed or inconsistent? [95]
- Scalability: Alignment methods that work for current AI might break down at the scale and intelligence level of AGI or ASI [87].
- Robustness: How do we ensure alignment holds true across all situations, especially novel ones the designers didn’t anticipate? How do we prevent “reward hacking” where the AI finds loopholes to maximize its reward signal without fulfilling the intended goal? [93]
- Value Evolution: Human values change over time. How can an AGI adapt, or know when not to adapt? [62]
Current Approaches to Alignment:
- Reinforcement Learning from Human Feedback (RLHF): Training models based on human ratings of their outputs (used in models like ChatGPT and Claude) [95].
- Constitutional AI: Providing AI with an explicit set of ethical principles or rules (a “constitution”) to guide its behavior, which it learns to follow during training (pioneered by Anthropic) [57].
- Inverse Reinforcement Learning (IRL): Trying to infer underlying goals or values by observing behavior [94].
- AI Safety via Debate/Critique: Training AI systems to debate each other or critique outputs to identify flaws or misalignments [95].
- Interpretability: Understanding the AI’s internal reasoning to check if it’s aligned with intended goals [87].
Solving the alignment problem is considered by many to be a prerequisite for safely developing Artificial General Intelligence [93]. OpenAI explicitly states that alignment research is core to their safety efforts [95].
8.3 Technical Safety Measures
Beyond the core alignment problem, researchers are developing various technical measures aimed at increasing the safety and controllability of potential AGI systems [87], [39]:
- Interpretability Tools: Techniques to peer inside the “black box” of complex AI models to understand how they arrive at decisions. This helps debug systems, detect biases, and verify alignment [87].
- Formal Verification: Using mathematical methods to prove that an AI system satisfies certain safety properties or constraints under specific conditions [94].
- Robustness Testing: Developing methods to test how AI systems perform under adversarial attacks (deliberate attempts to fool them) or distributional shifts (when real-world data differs from training data) [87].
- Containment Strategies (“Sandboxing”): Designing secure environments where potentially powerful AI systems can be tested and studied without risk of causing harm in the real world (e.g., limiting internet access, monitoring outputs) [32].
- Corrigibility / Interruptibility: Designing AI systems that are amenable to being corrected or shut down by humans, even if the AI becomes highly intelligent. This involves ensuring the AI doesn’t learn to resist shutdown as counterproductive to its goals [75].
- Tripwires / Anomaly Detection: Building monitoring systems to detect unexpected or potentially dangerous behavior in AI systems early on [39].
- Capability Control / Bounding: Developing methods to limit the capabilities of an AI system or ensure they develop gradually and controllably [87].
Google DeepMind’s 2025 safety paper details several such measures, including “access control, anomaly detection, logging and monitoring, and treating the model similarly to an untrusted insider” [39], [92]. These technical measures are crucial components of a layered defense-in-depth strategy for AGI safety.
8.4 Leading Organizations in AGI Safety
While many academic institutions contribute, several organizations specifically focus significant resources on AGI safety research [67]:
- Google DeepMind Safety Research: Has a large, dedicated team publishing extensively on technical safety, alignment, and governance [87], [89]. They recently released a short course on AGI safety [90].
- OpenAI Safety Teams: Focuses heavily on alignment research (e.g., RLHF, scalability), interpretability, and preparedness for future, more capable systems [95].
- Anthropic: Founded with safety as a primary mission, pioneering approaches like Constitutional AI and research into interpretability and robustness [57].
- Machine Intelligence Research Institute (MIRI): Focuses on foundational mathematical research aimed at ensuring highly reliable alignment for potentially superintelligent systems [75].
- Alignment Research Center (ARC): Focuses on developing techniques for evaluating AI systems for dangerous capabilities or misalignments before they are deployed [94].
- Center for AI Safety (CAIS): A non-profit conducting technical research and policy advocacy focused on mitigating large-scale AI risks [77].
- Future of Humanity Institute (FHI) (Oxford): Conducts broader research on existential risks, including those from AGI, often from philosophical and strategic perspectives [75].
- Various University Labs: Groups at Berkeley (e.g., CHAI), Stanford, CMU, Toronto, and others conduct vital academic research in AI safety and alignment [94].
The existence of these dedicated organizations highlights the growing recognition within the AI community and beyond that proactively addressing the safety challenges of Artificial General Intelligence is not just important, but potentially critical for humanity’s future [67].
9. Timeline Predictions for AGI
One of the most hotly debated questions surrounding Artificial General Intelligence is: when might it actually arrive? Predictions vary wildly, reflecting the immense uncertainty involved [31], [41].
9.1 Expert Forecasts and Predictions
Expert opinions on AGI timelines span a vast range [52]:
- Very Near-Term (Before 2030): A vocal minority, including figures like Elon Musk (predicting AGI surpassing human intelligence by 2025 or 2026) and some forecasters on platforms like Metaculus (median for ‘weak AGI’ briefly dipped to 2025/2026), believe AGI is imminent, potentially emerging within the next few years [42], [50]. Some argue recent LLM progress puts us on an exponential curve [201].
- Mid-Term (2030-2060): This range often represents the median or average view among surveyed AI researchers. For example, a large 2023 survey suggested a 50% chance of “high-level machine intelligence” (performing most tasks better than humans) by 2047 [52]. Another analysis from April 2025 suggested experts estimate AGI emergence between 2040-2050 (50% chance) [38].
- Long-Term (Beyond 2060): A significant portion of researchers remain skeptical about near-term AGI, believing fundamental breakthroughs are still required. They might place AGI arrival late in the 21st century, or even express doubt about it being achieved at all within this century [2], [40].
- Shrinking Timelines Trend: Notably, expert predictions have generally been shortening over the past decade. Surveys conducted years apart often show median AGI timelines decreasing, possibly influenced by rapid progress in deep learning and LLMs [41], [52]. One forecast aggregator saw the median prediction drop from 2040 to 2031 between 2022 and 2024 [41].
It’s crucial to treat all such predictions with caution. Forecasting transformative technological breakthroughs is notoriously difficult [43]. Historically, predictions have often been wrong, sometimes wildly so (both over-optimistic and over-pessimistic) [114]. Definitions of “AGI” also vary, affecting predictions [30].
9.2 Factors Influencing AGI Development Timelines
How quickly we reach (or don’t reach) Artificial General Intelligence depends on numerous interacting factors [35], [43]:
- Algorithmic Breakthroughs: Fundamental new ideas or algorithms (e.g., for causal reasoning, robust generalization, efficient learning) could dramatically accelerate progress. Conversely, hitting unexpected theoretical walls could slow it down [116].
- Computing Power (Hardware): Continued exponential growth in computing power (Moore’s Law, specialized AI chips like GPUs/TPUs, potentially quantum computing or neuromorphic chips) is often seen as a key enabler. Limits to computational scaling could become a bottleneck [37].
- Data Availability: Access to vast, diverse, high-quality datasets is crucial for training large models. Data limitations or privacy regulations could impact timelines [117].
- Investment & Resources: The massive influx of funding into AI research and artificial general intelligence companies currently accelerates development. Economic downturns or shifts in investment priorities could slow it [36], [56].
- Talent Pool: The number of skilled AI researchers and engineers is a factor. Educational pipelines and global talent mobility matter [46].
- Regulation & Geopolitics: Strict safety regulations could slow development (potentially beneficially). Intense geopolitical competition (e.g., a US-China AI race) could accelerate it, possibly at the expense of safety [73].
- Integration Challenges: Combining different AI techniques (e.g., neural + symbolic) or scaling current approaches might prove much harder than anticipated [115].
- Societal Acceptance & Backlash: Public perception, ethical debates, or incidents involving AI could influence funding and regulatory environments [40].
The interplay of these factors makes precise timeline prediction highly speculative [113].
9.3 Debates on AGI Feasibility and Timelines
The wide range of timeline predictions reflects deep disagreements within the AI community about the fundamental feasibility of AGI and the best path forward [40], [153]:
- Scaling Hypothesis: Is current deep learning technology, particularly LLMs, fundamentally on the right track? Can we reach AGI simply by scaling up models with more data and compute? Proponents say yes, pointing to emergent capabilities [155], [201]. Skeptics argue that scaling alone won’t overcome limitations like lack of understanding and common sense, and fundamental architectural innovations are needed [154], [157], [198].
- LLMs as a Path to AGI: This is a major point of contention. Some see LLMs as direct precursors or components of AGI [158]. Others view them as impressive but ultimately limited tools, incapable of true general AI without integration with other approaches (like symbolic reasoning or world models) [154], [159], [162].
- The Role of Neuroscience & Embodiment: How closely must AGI mimic the human brain or interact with the physical world? Some argue biological inspiration and embodiment are crucial [186], while others believe intelligence can be achieved through purely computational means [180].
- The Nature of Intelligence & Consciousness: Underlying these debates are philosophical questions about what intelligence truly is, and whether consciousness is necessary for AGI. Can understanding and consciousness “emerge” from complex computation, or do they require something more? [112]
- Imminent AGI Claims: High-profile claims of imminent AGI by tech leaders are often met with skepticism from academic researchers who point to the remaining fundamental challenges [40], [124]. There’s a notable gap between some public narratives and the median expert view [40].
These ongoing debates highlight that the path to Artificial General Intelligence is far from clear, and significant scientific uncertainty remains [121].
10. The Path Forward
Given the immense potential and profound risks of Artificial General Intelligence, charting a responsible path forward requires careful consideration of research priorities, collaboration, and societal adaptation [89].
10.1 Research Priorities and Challenges
To make progress toward beneficial AGI while managing risks, the AI community needs to focus on several key areas [177], [182]:
- Robust Generalization & Common Sense: Moving beyond pattern matching to imbue AI with genuine understanding, causal reasoning, and the ability to apply knowledge flexibly in novel situations remains paramount [154], [166].
- Integration Architectures: Developing frameworks (like advanced cognitive architectures or neuro-symbolic systems) that can effectively integrate diverse capabilities – perception, memory, reasoning, learning, planning – into a cohesive whole [164], [195].
- Safety & Alignment by Design: Making safety a core design criterion from the outset, not an afterthought. This includes advancing research in interpretability, control, value alignment, and formal verification [87], [95].
- Efficiency & Scalability: Finding ways to achieve greater capabilities with less data and computational power, making advanced AI more accessible and sustainable [188]. Research into neuromorphic computing or new learning paradigms is relevant here [175].
- Reliable Evaluation: Creating better benchmarks and evaluation methodologies that measure true general intelligence and safety properties, rather than narrow task performance [30], [124].
- Understanding Intelligence: Continuing fundamental research into the nature of both biological and artificial intelligence to provide a stronger theoretical foundation for AGI development [112].
As IBM researchers and others have argued, achieving human-like intelligence likely requires integrating structured knowledge and causal reasoning, suggesting that deep learning alone, while powerful, may not be sufficient [188].
10.2 Collaborative Approaches to Responsible AGI
The global scale and potential impact of Artificial General Intelligence necessitate unprecedented levels of collaboration [89], [58]:
- Global Dialogue & Governance: Establishing international forums for dialogue among nations, companies, researchers, and civil society to develop shared norms, safety standards, and potentially treaties for AGI development [73].
- Multi-stakeholder Engagement: Ensuring that discussions about AGI’s future are inclusive, incorporating perspectives from ethics, law, social sciences, economics, and diverse cultural backgrounds, not just technologists [57].
- Openness & Transparency (where safe): Promoting transparency in research (capabilities, limitations, safety techniques) can foster trust and accelerate safety progress. However, this needs balancing against risks of misuse (the “dual-use” problem) [87].
- Collaborative Safety Research: Encouraging pre-competitive collaboration on safety research, allowing organizations to share insights and best practices for mitigating risks without compromising commercial interests [89].
- Independent Auditing & Monitoring: Developing mechanisms for independent third-party auditing of advanced AI systems and potentially international monitoring bodies to track capabilities and compliance with safety agreements [58].
Google DeepMind’s emphasis on “collaboration with the AI community” and engaging in “vital conversations” reflects a growing consensus that navigating the path to AGI safely cannot be done in isolation [89].
10.3 Preparing Society for an AGI Future
Beyond the technical and governance challenges, proactively preparing society for the potential disruptions and transformations of an AGI era is crucial [110]:
- Education Reform & Lifelong Learning: Adapting education systems to focus on skills that complement AI (critical thinking, creativity, collaboration, emotional intelligence) and establishing robust systems for lifelong learning and workforce retraining [79].
- Economic Safety Nets & Transitions: Developing policies to manage potential large-scale job displacement caused by automation, such as exploring Universal Basic Income (UBI), strengthening social safety nets, and investing in transition support for affected workers [69].
- Public Discourse & Literacy: Fostering informed public understanding of AI capabilities, limitations, and societal implications, moving beyond hype and fear to enable constructive dialogue [40].
- Ethical & Legal Adaptation: Updating legal frameworks to address questions of AI personhood, responsibility, bias, and privacy. Developing societal norms for human-AI interaction [71].
- Psychological & Cultural Adaptation: Helping individuals and communities adapt to a world where humans may no longer be the sole proprietors of high-level intelligence, considering the psychological impacts and potential shifts in human identity and purpose [66].
As McKinsey suggests, a human-centered approach, focusing on augmenting human capabilities rather than simply replacing them, is key [110]. Proactive societal preparation can help ensure that the transition to an AGI-influenced future is smoother, more equitable, and ultimately beneficial.
11. Conclusion
11.1 Summary of Key Insights
Our journey through the complex landscape of Artificial General Intelligence reveals several critical takeaways:
- AGI Defined: AGI represents hypothetical AI with human-like cognitive abilities across diverse tasks, distinct from today’s specialized Narrow AI (ANI).
- Current Status: True AGI remains theoretical. While AI capabilities (especially LLMs) have advanced rapidly, fundamental gaps in understanding, reasoning, and adaptability persist.
- Diverse Paths: Research explores multiple avenues, including scaling neural networks, symbolic reasoning, hybrid neuro-symbolic systems, and cognitive architectures, with increasing focus on integrated approaches.
- Uncertain Timelines: Expert predictions for AGI arrival vary dramatically, from years to decades or longer, reflecting deep uncertainties and ongoing debates about feasibility.
- Transformative Potential: AGI holds the promise of revolutionizing science, medicine, education, and countless other fields, potentially solving major global challenges.
- Profound Risks: Development carries significant risks, including misalignment, misuse, economic disruption, and even existential threats, necessitating robust safety research and ethical frameworks.
- Safety is Paramount: The alignment problem – ensuring AGI goals match human values – is a central challenge. Technical safety measures and responsible governance are crucial.
- Collaboration is Key: Navigating the path forward requires global cooperation, multi-stakeholder dialogue, and proactive societal preparation.
11.2 The Future of AGI and Humanity
The prospect of Artificial General Intelligence forces us to contemplate the future trajectory of humanity itself. It’s a future filled with both dazzling possibilities and sobering risks.
AGI could become humanity’s most powerful tool, a partner in overcoming disease, poverty, and environmental degradation, unlocking unprecedented levels of creativity and discovery. It might usher in an era of abundance and expanded human potential.
However, the path is perilous. Mismanaged, AGI could lead to irreversible catastrophe. The control problem, the alignment challenge, and the potential for misuse demand our utmost caution and wisdom.
The quest for AGI compels us to reflect on our own intelligence, our values, and what we want our future to look like. It challenges us to define what aspects of the human experience we wish to preserve and enhance.
Ultimately, the development of Artificial General Intelligence is not just a technological race; it’s a test of humanity’s foresight, cooperation, and ethical maturity. The choices we make now – about research priorities, safety investments, governance structures, and societal adaptation – will echo far into the future.
As AI pioneer Stuart Russell warns, the core concern is intelligence that might not prioritize human preferences [9]. Our collective task is immense: to steer the development of increasingly powerful AI toward outcomes that are not just intelligent, but also wise, beneficial, and aligned with the flourishing of all humanity. Approaching this monumental challenge with humility, responsibility, and a shared commitment to a positive future is not just advisable – it may be essential for our survival and prosperity in the age of Artificial General Intelligence.
Disclaimer: This article incorporates information and citations. While aiming for accuracy based on that input and general knowledge up to early 2025, the field of AI evolves rapidly. Specific predictions and the status of research groups may change.