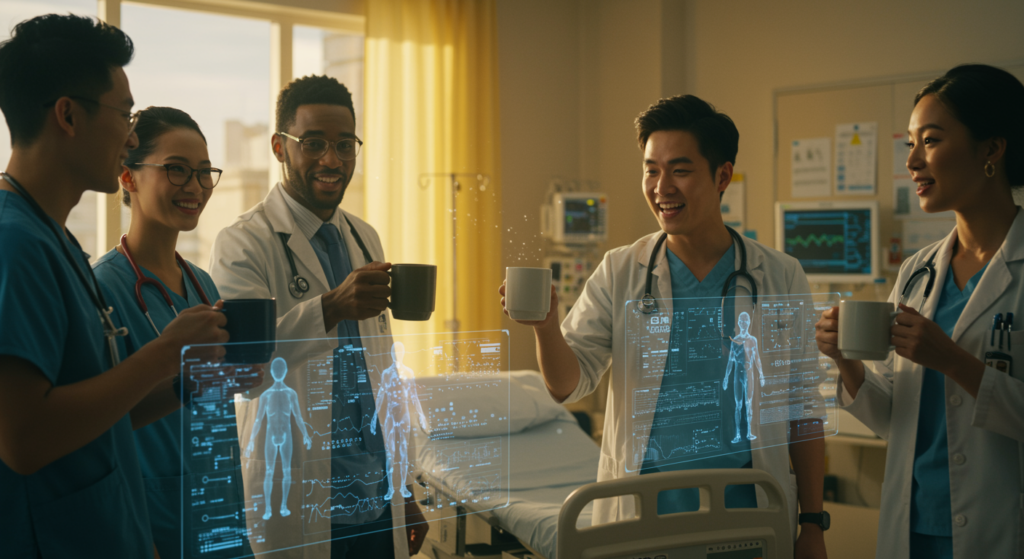
AI in Healthcare: Leaders Must Set Essential AI Usage Rules
Introduction
Imagine a world where AI in healthcare doesn’t just streamline operations but truly enhances patient outcomes without cutting corners on ethics. That’s the potential we’re unlocking today, as AI technologies transform diagnostics and care delivery. Yet, healthcare leaders must step up with clear rules to navigate risks and ensure AI in healthcare benefits everyone fairly and safely.
Why AI is Transforming Healthcare
AI in healthcare is more than a trend—it’s a game-changer, leveraging machine learning and data analytics to spot issues early and optimize resources. For instance, AI can analyze medical images faster than humans, enabling early detection of conditions like cancer. This means not only better diagnoses but also personalized treatments that save lives and cut costs.
Have you ever thought about how AI could automate routine tasks, freeing doctors to focus on what matters most? By processing vast datasets, AI promises to boost efficiency in areas like drug discovery, where it speeds up trials and identifies new therapies. But for these gains to stick, adoption must be thoughtful, ensuring tools are reliable and equitable.
The Critical Role of Leadership in AI Adoption
At the helm of this shift, healthcare leaders are the ones who can turn AI’s potential into reality. They’re not just managing tech; they’re safeguarding patient trust and preventing pitfalls like biased algorithms. Without strong guidance, AI in healthcare could lead to unequal care or privacy issues, eroding the very foundations of medicine.
Establishing Governance and Oversight
Think of governance as the backbone of AI integration—it’s what keeps everything aligned. Leaders should create policies that cover ethical and legal aspects, making sure AI tools are used responsibly. For example, regular audits can catch problems early, like unintended biases in predictive models.
- Policy creation: Develop rules that address AI’s clinical and ethical impacts, drawing from real-world examples where lapses led to errors.
- Continuous oversight: This involves monitoring AI performance and adapting as needed, perhaps through dedicated teams that review outputs weekly.
- Accountability: Pinpoint who’s responsible—clinicians, developers, or admins—to build trust and handle issues swiftly.Brookings Report
Main Challenges in AI Implementation
While AI in healthcare offers exciting possibilities, it’s not without hurdles that demand proactive solutions. Leaders must tackle these head-on to avoid setbacks that could harm patients or erode confidence in the technology.
1. Data Quality and Accessibility
High-quality data is the fuel for AI, yet many systems grapple with incomplete or biased datasets. Imagine an AI tool trained mostly on data from one demographic—it might overlook nuances in others, leading to misdiagnoses. This is why addressing data scarcity for rare diseases is crucial.
- Data gaps in minority groups can widen health disparities.
- Fragmented records across systems make integration tough, but standardization efforts can bridge these divides.PMC Study
- Poor data formats hinder AI’s effectiveness, so leaders should push for unified protocols.
2. Privacy and Security Risks
With AI handling sensitive health data, breaches are a real threat that could expose personal information. Consider a hospital system hacked—patients might lose trust forever. Regulations like HIPAA are essential, but leaders need to enforce them rigorously.
- Third-party vendors often handle data, increasing misuse risks.
- Staying compliant with laws prevents fines and protects privacy.HITRUST Insights
- What if a simple encryption update could thwart most attacks? That’s an actionable step worth prioritizing.
3. Algorithmic Bias and Fairness
Bias in AI algorithms can perpetuate inequalities, such as overlooking symptoms in certain populations. For healthcare leaders, ensuring fairness means testing models against diverse data sets from the start. This isn’t just about accuracy; it’s about delivering equitable care.
- Marginalized groups often face underdiagnosis due to flawed training.
- “Black-box” decisions frustrate clinicians, so pushing for explainable AI is key.MedPro Analysis
- By monitoring outcomes, leaders can adjust systems to reduce disparities.
4. Integration and Interoperability
Seamless AI integration is like fitting a puzzle piece—it’s essential for smooth operations. Yet, incompatible systems often create roadblocks, slowing down innovation in AI in healthcare. Leaders can solve this by promoting open standards.
- Interoperability issues between EHR systems waste time and resources.
- Harmonizing data from various sources prevents silos.Ominext Blog
- Avoid vendor lock-in by choosing flexible platforms that encourage competition.
5. Workforce Disruption and Acceptance
AI might disrupt jobs, but it can also empower staff if handled right. Many professionals worry about losing control, so training is vital to build acceptance. Picture a nurse using AI to double-check prescriptions—it’s a partnership that enhances, not replaces, human insight.
- Upskilling programs can ease fears of job loss.
- Encourage collaboration to maintain clinician autonomy.HIMSS Resource
- How can leaders foster a culture where AI is seen as an ally? Start with open dialogues and hands-on training.
Essential Rules for Responsible AI Usage in Healthcare
To make AI in healthcare work for all, leaders need rules that balance innovation with safeguards. These aren’t just guidelines—they’re strategies to build a trustworthy system. Let’s break down how to implement them effectively.
1. Data Governance and Stewardship
Strong data rules start with patient consent and privacy protocols. Regular audits ensure data integrity, preventing errors that could arise from poor quality. By promoting shared exchanges, leaders can democratize access and reduce monopolies.
- Set strict consent processes to respect patient rights.
- Audits help maintain data accuracy and fairness.
- Collaborative sharing via HIEs boosts innovation.Brookings Report
2. Algorithmic Transparency and Explainability
Transparency turns AI from a mystery into a tool clinicians can trust. Documenting training processes ensures models are reliable and unbiased. For example, using explainable AI lets doctors understand recommendations, improving decision-making.
- Require detailed model documentation.
- Implement bias checks to promote equity.
- “White-box” systems enhance trust and usability.PMC Study
3. Accountability and Liability
Clear accountability means knowing who’s answerable when things go wrong. Leaders should establish review boards and error-reporting systems to handle AI-related issues. This builds a safety net that protects patients and providers alike.
- Define roles for clinicians and developers.
- Create mechanisms for investigating outcomes.
- Transparent oversight ensures ongoing improvements.HITRUST Insights
4. Regulatory Compliance
Staying ahead of regulations like GDPR keeps AI in healthcare on solid ground. Design systems with compliance in mind, integrating security from the outset. This proactive approach minimizes risks and supports ethical practices.
- Track updates in AI laws globally.
- Build compliant frameworks by default.
5. Equity and Inclusion
Equity ensures AI serves everyone, not just a select few. Involve diverse groups in AI development to address care gaps. Monitoring performance across demographics helps catch and fix inequalities early.
- Include underrepresented voices in testing.
- Prioritize solutions for underserved areas.
- Track outcomes to maintain fairness.MedPro Analysis
6. Education and Change Management
Education turns resistance into enthusiasm, equipping staff to work with AI effectively. Invest in training programs that emphasize collaboration, ensuring AI augments human expertise. Fostering an adaptable culture makes all the difference in smooth adoption.
- Provide AI literacy courses for professionals.
- Promote teamwork between humans and tech.
- Encourage open communication for innovation.HIMSS Resource
Future Outlook: AI and the Promise of Better Healthcare
Looking ahead, AI in healthcare could lead to breakthroughs like faster disease detection and personalized medicine. With the right rules in place, we might see reduced workloads for clinicians and better access for underserved communities. But success depends on ongoing collaboration and ethical vigilance.
- AI could revolutionize diagnostics with precision.
- It promises to ease administrative burdens.
- Greater equity in care is within reach if leaders act now.
As technologies evolve, so must our approaches—always with patients at the center.
Conclusion
AI in healthcare holds immense power, but it’s up to leaders to guide it wisely. By prioritizing governance, transparency, and equity, we can minimize risks and maximize benefits. What steps will you take in your organization? Share your thoughts in the comments, explore our related posts on ethical tech, or connect with us to discuss AI strategies further.
References
- MedPro. “Challenges and Risks of Artificial Intelligence.” Link
- PMC. “Article on AI in Healthcare.” Link
- Ominext. “Challenges of AI Integration in Healthcare.” Link
- Brookings. “Generative AI in Health Care.” Link
- HITRUST. “The Pros and Cons of AI in Healthcare.” Link
- ForeseeMD. “Artificial Intelligence in Healthcare.” Link
- HIMSS. “Impact of AI on Healthcare Workforce.” Link
- PMC. “Another Article on AI Ethics.” Link
AI in Healthcare, healthcare leadership, AI regulation, ethical AI, healthcare data security, AI ethics, data privacy in healthcare, AI governance, medical AI challenges, AI innovation in medicine