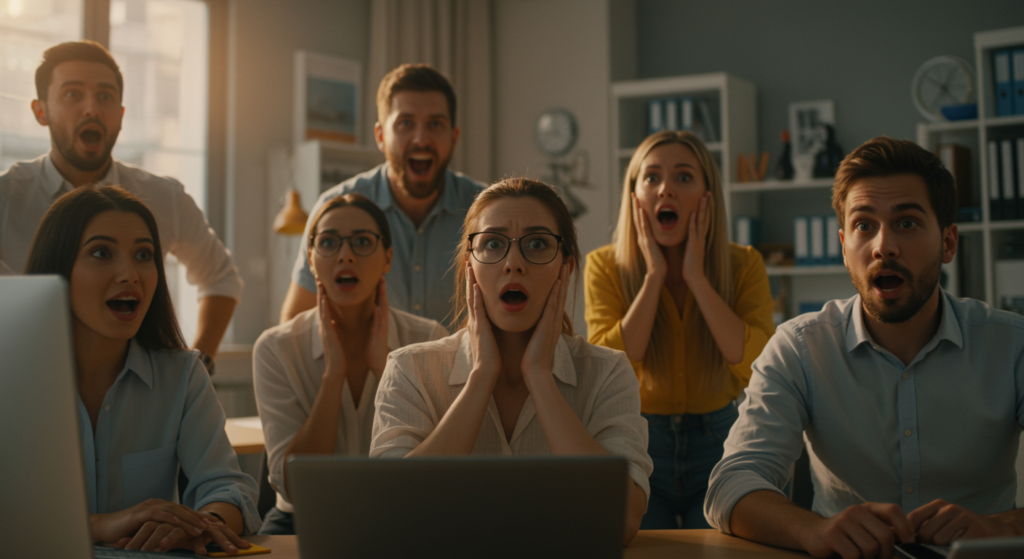
NVIDIA AI Security Risks in Translation and Speech Microservices
Exploring NVIDIA AI Security Risks in Speech and Translation
NVIDIA AI security risks have become a hot topic as companies like NVIDIA push the boundaries of innovation with platforms such as NVIDIA Riva. This system powers real-time conversational AI, handling everything from multilingual speech recognition to neural machine translation and text-to-speech features. Imagine deploying AI that can seamlessly translate languages or generate lifelike voices—it’s transformative for businesses, but it also opens doors to potential threats that need careful management.
With Riva’s ability to scale from cloud setups to edge devices and integrate with large language models, the risk of exposure grows. Have you ever wondered how a simple misconfiguration could turn cutting-edge tech into a liability? That’s where understanding NVIDIA AI security risks steps in, helping enterprises protect their investments while leveraging these powerful tools.
NVIDIA Riva: Recent Vulnerabilities Spotlighting Security Risks
Despite its strengths, NVIDIA Riva has faced scrutiny due to specific security risks, notably through vulnerabilities like CVE-2025-23242 and CVE-2025-23243. These issues, identified in cloud-based deployments, highlight how exposed API endpoints can lead to unauthorized access and broader NVIDIA AI security risks.
For instance, many Riva instances were left publicly accessible without proper authentication, making it easy for attackers to tap into AI inference services. This not only risks resource abuse but also paves the way for data leakage, where sensitive information could slip through the cracks.
- Exposed API Endpoints: Without robust controls, these endpoints become gateways for NVIDIA AI security risks, allowing outsiders to exploit GPU resources.
- Resource Abuse and Data Leakage: Attackers might hijack systems for intellectual property theft, turning a company’s AI assets into vulnerabilities.
- Denial-of-Service Attacks: Unrestricted access can disrupt operations, emphasizing the need to address these NVIDIA AI security risks head-on.
Organizations dealing with proprietary data must prioritize securing Riva to avoid these pitfalls, as even a small oversight can escalate into major threats.
Wider Implications of AI Security Risks in Microservices
The challenges with Riva reflect larger AI security risks in microservices, where shadow IT practices often create blind spots. Departments might deploy AI tools without IT approval, leading to compliance issues and unnoticed vulnerabilities.
Traditional security measures fall short against the dynamic nature of AI, especially with data flowing across platforms. For example, deep learning models lack transparent decision-making, making it tough to audit and comply with regulations—another facet of NVIDIA AI security risks that demands attention.
- Shadow IT Concerns: This can introduce NVIDIA AI security risks through unmanaged deployments of small language models.
- Limited Security Protocols: Distributed systems amplify risks, as seen in cross-platform data flows.
- Data Leakage: Tools like NVIDIA Morpheus use NLP to detect real-time leaks, helping mitigate these security risks effectively.
Have you considered how these AI security risks could affect your own team’s workflows? Staying proactive is key to preventing them.
Innovations Addressing AI Microservices Security Risks
NVIDIA has rolled out features to combat AI security risks, particularly in their NIM microservices under NeMo Guardrails. These tools restrict AI outputs to safe topics and block potential “jailbreak” attempts, ensuring more secure interactions.
Granular access controls, such as secure API keys and user screenings, form the backbone of defending against NVIDIA AI security risks. Real-time monitoring via tools like Morpheus adds another layer, using AI to spot anomalies quickly.
- Content Safety Measures: These innovations directly tackle NVIDIA AI security risks by enforcing conversation guidelines.
- Traffic Monitoring: Digital fingerprinting helps in early detection, turning potential risks into manageable alerts.
- Automated Management: AI agents speed up vulnerability fixes, reducing exposure time for these security risks.
This evolution shows how addressing NVIDIA AI security risks isn’t just about fixes—it’s about building resilient systems from the ground up.
A Case Study on Exploiting AI Security Risks
Let’s dive into a real-world scenario to illustrate NVIDIA AI security risks: Suppose a company deploys Riva with default settings in the cloud. Without authentication, the endpoints are wide open, inviting trouble.
An attacker could easily discover and exploit this, hijacking GPU resources for unauthorized tasks or stealing processed data. In this case, the company might face not only data breaches but also service disruptions from denial-of-service attacks tied to these security risks.
- First, lack of safeguards allows access to sensitive AI functions.
- Next, attackers leverage this for resource abuse or data exfiltration.
- Finally, the fallout includes potential intellectual property loss, underscoring the critical nature of NVIDIA AI security risks.
This example highlights why proactive measures are essential—could your organization handle a similar situation?
Strategies to Mitigate AI Security Risks for Enterprises
Immediate Steps to Address Security Risks
- Audit Deployments: Regularly check for exposed endpoints to minimize NVIDIA AI security risks in your setup.
- Enforce Authentication: Always use encrypted keys to block unauthorized access and related security risks.
- Monitor Activity: Set up alerts for unusual patterns, helping to catch NVIDIA AI security risks early.
Long-Term Best Practices Against Security Risks
- Granular Controls: Limit access to AI models, reducing the chances of NVIDIA AI security risks during development.
- Defense-in-Depth Approach: Combine traditional security with AI-specific tools to layer protection against these risks.
- Continuous Assessment: Evaluate new AI capabilities to stay ahead of emerging security risks.
- Incident Coordination: Use resources like NVIDIA’s bulletins for swift responses to security risks.
- Staff Training: Educate teams on AI threats to build resilience against NVIDIA AI security risks.
Implementing these can make a real difference—think of it as fortifying your digital defenses step by step.
Comparing Traditional IT and AI Microservices Security Risks
Aspect | Traditional IT Security | AI Microservices Security |
---|---|---|
Access Controls | Relies on firewalls and role-based systems | Involves dynamic keys and output filters to combat NVIDIA AI security risks |
Threat Detection | Uses static methods like antivirus | Employs AI-driven analysis to address evolving security risks |
Deployment | Centralized for easier management | Distributed, increasing exposure to NVIDIA AI security risks |
Incident Response | Often manual and slow | Features automated tools to quickly mitigate security risks |
Compliance | Standard frameworks in place | Faces challenges with audit trails amid NVIDIA AI security risks |
This comparison shows how AI introduces unique security risks that demand tailored strategies.
The Future of Managing AI Security Risks in Microservices
As AI advances, so do the associated security risks, particularly with tools like NVIDIA Riva. Enterprises must adopt vigilant practices, from enhanced monitoring to ongoing training, to safeguard their speech and translation systems.
With innovations in content safety and vulnerability detection, staying ahead of NVIDIA AI security risks is more achievable than ever. What steps will you take to ensure your AI deployments remain secure?
We encourage you to share your experiences in the comments below, explore our related posts on AI best practices, or connect with experts for more insights. Let’s build a safer AI future together.
References
- Trend Micro Research. (2025). NVIDIA Riva Vulnerabilities. Link
- NVIDIA. (2025). Frontier AI Risk Assessment. Link
- NVIDIA. AI Cybersecurity Solutions. Link
- NVIDIA. Safe and Found Resources. Link
- DIGITAL WATCH. New NVIDIA Microservices for AI Security. Link
- FTSG. 2025 Threat Report. Link
- NVIDIA. Riva Product Page. Link
- Galileo. AI Blog. Link
NVIDIA AI security risks, translation microservices, speech microservices, Riva vulnerabilities, AI risk mitigation, NVIDIA Riva security, AI microservices challenges, neural machine translation risks, text-to-speech security, enterprise AI protection