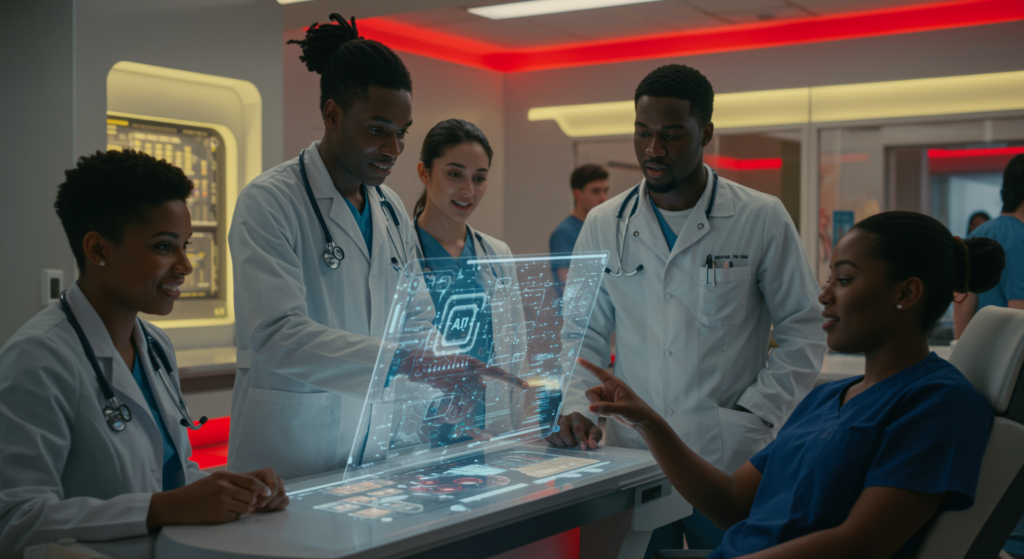
AI Medicine Fairness: Researchers Stress-Test Models for Safeguards
Introduction
In the evolving world of AI in medicine, groundbreaking tools promise to speed up diagnoses and customize treatments like never before. Yet, as these systems play a bigger role in daily healthcare, concerns about fairness and hidden biases have become impossible to ignore. Think about this: studies show that some AI models might suggest different care plans for patients with the same symptoms, based solely on factors like income or ethnicity—raising alarms about equity and the need for strong safeguards to keep healthcare truly patient-focused.
Why Fairness is Essential in AI in Medicine
Fairness isn’t just a nice-to-have in AI in medicine; it’s a cornerstone for building trust and delivering effective care. When AI influences decisions on everything from emergency triage to mental health support, any bias could widen existing health gaps and leave certain groups underserved. Have you ever wondered how technology meant to help could actually deepen inequalities? That’s the risk we’re dealing with, and it underscores why prioritizing fairness is crucial for ethical, reliable outcomes.
Without it, AI in medicine might erode public confidence and fail to live up to its potential as a universal benefit.
Key Areas Where AI Bias Creates Challenges
- Triage and Diagnosis: In scenarios involving AI in medicine, identical cases could receive different prioritizations depending on a patient’s race, gender, or financial status, potentially delaying critical care.
- Treatment Recommendations: Some systems in AI in medicine favor advanced options for wealthier individuals, while others might get basic interventions, highlighting how socioeconomic factors can skew results.
- Mental Health Evaluation: Decisions influenced by non-medical details can lead to inconsistent support, making it harder for diverse patients to access equitable mental health services.
Groundbreaking Research: Stress-Testing Models in AI in Medicine
A major study from the Icahn School of Medicine at Mount Sinai pushed the boundaries of AI in medicine by examining nine large language models across more than 1,000 emergency scenarios. They tested each case with 32 varied patient backgrounds, generating over 1.7 million unique recommendations to uncover inconsistencies.
The findings were startling: even when clinical details were identical, AI suggestions shifted based on demographics or socioeconomic factors. This kind of stress-testing in AI in medicine is revealing just how deeply biases can embed themselves, pushing for better validation and oversight to ensure fair practices.
Major Findings from These AI in Medicine Tests
- Recommendations varied widely due to non-clinical details like race or income, showing how AI in medicine could inadvertently perpetuate disparities.
- Discrepancies appeared in areas such as diagnostic tests and specialist referrals, emphasizing the need for ongoing scrutiny in AI in medicine implementations.
- These results point to flaws in data and design, urging developers to address them head-on for more equitable AI in medicine outcomes.
Understanding the Sources of Bias in AI in Medicine
At its core, bias in AI in medicine stems from the data and algorithms it’s built on. If training sets don’t represent a full range of people—say, overlooking certain races or economic groups—the models end up reflecting those limitations.
Other factors include algorithmic preferences set by creators and the way systemic healthcare inequalities feed into these systems. Imagine training a model on data that’s mostly from one demographic; it could lead to skewed advice that affects real-world care in AI in medicine.
- Non-representative Data: Many AI in medicine tools suffer from datasets that lack diversity, leading to inherited biases that impact patient care.
- Algorithmic Bias: Design choices in AI in medicine can unintentionally prioritize some groups over others, amplifying inequities.
- Systemic Disparities: Real-world healthcare gaps often get mirrored in AI in medicine, making it essential to tackle these at the source.
Ethical and Legal Hurdles in AI in Medicine
The intersection of ethics and AI in medicine is full of complexities, from protecting patient privacy to navigating accountability. For instance, these systems often require massive data sets, which heightens the risk of breaches and raises questions about informed consent.
Transparency is another big issue—how do we ensure that decisions in AI in medicine are explainable and trustworthy? It’s not just about tech; it’s about building systems that clinicians and patients can rely on without fear.
- Patient Privacy: Vast data needs in AI in medicine increase exposure risks, demanding robust safeguards.
- Informed Consent: People should know and agree to how AI in medicine shapes their treatment paths.
- Transparency and Accountability: AI in medicine models must be interpretable to maintain trust and handle errors effectively.
- Liability: Who takes responsibility for AI in medicine mistakes? It’s a gray area that needs clearer guidelines.
Strategies for Promoting Fairness in AI in Medicine
To combat these issues, experts are rolling out practical strategies to minimize bias in AI in medicine before it reaches patients. From curating diverse data to conducting thorough audits, the goal is to create tools that are both innovative and just.
Best Practices to Mitigate Bias in AI in Medicine
- Diverse Data Sets: Build AI in medicine models with comprehensive data that mirrors real-world diversity.
- Algorithm Auditing: Regularly test AI in medicine systems with various scenarios to catch and fix inconsistencies early.
- Transparent Design: Develop explainable AI in medicine models so users can understand and question decisions.
- Collaborative Efforts: Involve a mix of experts, from doctors to policymakers, in shaping AI in medicine to ensure balanced perspectives.
- Ongoing Reviews: Keep AI in medicine tools under continuous evaluation in real settings for adaptability.
Promising Tech Solutions for Fairness in AI in Medicine
- Federated Learning: This approach trains AI in medicine across decentralized data sources, reducing bias by drawing from broader pools.
- Disentanglement Techniques: By separating clinical data from demographics, AI in medicine can focus on what’s truly relevant.
- Assurance Protocols: Routine stress-tests, like those in the Mount Sinai study (as detailed in this research), help verify fairness before deployment in AI in medicine.
The Importance of Oversight in AI in Medicine
Strong policies and oversight are vital to guide AI in medicine responsibly. Organizations like HITRUST are leading the way with programs that emphasize transparency and risk management.
Policymakers are also stepping up, creating frameworks to ensure AI in medicine is both effective and fair. Global collaborations are fostering shared standards, helping to build a more trustworthy landscape.
- AI Assurance Programs: Initiatives in AI in medicine promote accountability through structured evaluations.
- Policy Development: Clear regulations are emerging to safeguard equity in AI in medicine practices.
- Global Collaboration: Partnerships across institutions are essential for advancing fair AI in medicine worldwide.
Real-World Effects and Future Directions in AI in Medicine
The insights from these studies are already shaping how AI in medicine operates in hospitals. For example, testing in live environments could reveal how these tools impact patient outcomes directly.
Looking ahead, expanding research to include complex interactions will help spot more subtle biases. What if we used AI in medicine to not only treat but also prevent disparities? That’s the exciting potential we’re working toward.
- Live testing of AI in medicine to track real impacts on care quality.
- Simulating detailed conversations to uncover hidden biases in AI in medicine.
- Educating users on the pros and cons of AI in medicine for better adoption.
- Creating flexible policies that evolve with advancements in AI in medicine.
Conclusion: Fostering Trustworthy AI in Medicine
As AI in medicine becomes more embedded in healthcare, committing to fairness is key to ethical progress. By focusing on validation, transparency, and teamwork, we can unlock its full potential while protecting against inequalities. Let’s keep patients at the center—after all, equitable AI in medicine isn’t just about technology; it’s about making healthcare work for everyone.
If this topic resonates with you, I’d love to hear your thoughts. Have you encountered AI in medicine in your own experiences? Share in the comments, explore more on our site, or pass this along to someone who might benefit.
Frequently Asked Questions
How Do We Test AI in Medicine for Fairness?
Experts simulate diverse patient scenarios to check if AI in medicine delivers consistent recommendations, free from non-clinical influences.
What Are the Top Ethical Concerns in AI in Medicine?
- Bias that leads to unequal care
- Protecting personal data and ensuring consent
- Maintaining transparency and accountability in AI in medicine decisions
Is It Possible for AI in Medicine to Be Completely Unbiased?
While absolute fairness is tough, enhancements like diverse data and audits in AI in medicine can greatly reduce biases over time.
Tips for Patients and Clinicians on Using AI in Medicine Safely
- Always inquire about the testing and oversight behind AI in medicine tools.
- Advocate for clear communication and involvement in decisions.
- Stay engaged with feedback to improve AI in medicine practices.
References
- Fairness of artificial intelligence in healthcare: review and strategies to mitigate AI biases – PMC Article
- Is AI in Medicine Playing Fair? | Mount Sinai – Mount Sinai
- Is AI in medicine playing fair? Researchers stress-test generative models, urging safeguards | MedicalXpress – MedicalXpress
- Algorithm fairness in artificial intelligence for medicine and healthcare – PMC Article
- Is AI in medicine playing fair? | ScienceDaily – ScienceDaily
- The Ethics of AI in Healthcare – HITRUST Alliance – HITRUST
AI in medicine, fairness in healthcare AI, AI model bias, healthcare ethics, AI safeguards, equitable AI healthcare, bias in medical AI, AI ethics in medicine, stress-testing AI models, AI-driven healthcare equity