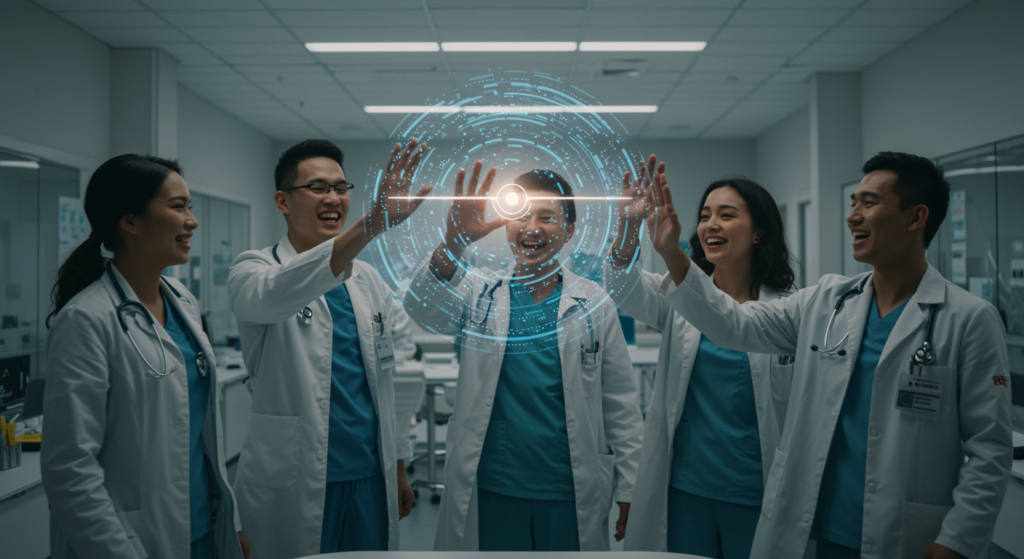
AI Fairness in Healthcare: Addressing Bias in Medical AI
Introduction
In today’s fast-evolving world, AI fairness in healthcare is emerging as a vital concern as artificial intelligence transforms how we approach patient care and medical decisions. Think about it: AI tools are now helping diagnose diseases, recommend treatments, and allocate resources, but they can unintentionally favor some groups over others. This article dives into why addressing bias in medical AI isn’t just a technical fix—it’s a step toward truly equitable health outcomes for everyone, no matter their background.
Understanding AI Fairness in Healthcare
AI fairness in healthcare means building and using systems that deliver the same high-quality results for all patients, regardless of race, gender, or economic status. Have you ever considered how an unfair algorithm might lead to misdiagnoses in underrepresented communities, like delaying critical care for women or people of color? These imbalances don’t just hurt individuals; they widen health disparities and erode trust in technology.
For instance, if AI tools rely on skewed data, they could perpetuate inequalities that have persisted for years. Ensuring AI fairness in healthcare is both an ethical must and a practical one—it’s key to improving everyone’s well-being and making sure innovations benefit society as a whole. According to a study from PMC, fair AI practices can directly boost patient trust and outcomes.
The Roots of Bias in Medical AI
Data Bias and Its Challenges
At the heart of many issues lies data bias, where AI systems learn from datasets that don’t fully represent diverse populations. Picture this: an AI designed to detect skin cancer trained mostly on images of lighter skin tones might miss signs in darker skin, putting certain groups at risk. This type of bias in AI fairness in healthcare stems from historical data gaps, often excluding minorities or low-income areas.
To combat this, developers must prioritize inclusive data collection, but it’s not always straightforward. For example, rural communities might lack the resources for comprehensive health records, amplifying the problem. As researchers from Harvard highlight, confronting these biases requires ongoing scrutiny to avoid real-world harm.
Algorithmic Bias in Practice
Even with good data, algorithmic bias can sneak in through the design process. If programmers overlook population differences or base models on outdated practices, the results could reinforce inequality. What if an algorithm assumes certain symptoms are more common in one demographic based on flawed assumptions? That’s exactly what happens in some diagnostic tools, leading to inequities.
Strategies for AI fairness in healthcare involve rethinking how algorithms are built, perhaps by incorporating checks for variability. A study from PMC emphasizes that transparent modeling can prevent these pitfalls, ensuring algorithms serve as tools for progress rather than perpetuators of division.
Human and Institutional Influences
Bias doesn’t stop at data or code; it often reflects deeper human and institutional flaws. For example, if healthcare systems have historically underserved certain groups, that gets baked into the AI through biased inputs. Here’s a relatable scenario: A clinic that provides less screening for low-income patients might train an AI that overlooks those needs, creating a vicious cycle.
Tackling this demands awareness and reform, like training programs for developers and providers. By addressing these roots, we can advance AI fairness in healthcare and build more just systems.
Real-World Impact: How Bias in AI Fairness in Healthcare Hurts Patients
The effects of unchecked bias are all too real, with studies showing higher barriers to treatment for racial minorities. Imagine a parent whose child faces delayed diagnosis because an AI algorithm sets a tougher threshold for intervention—it’s heartbreaking and avoidable. These issues not only exacerbate health disparities but also strain community resources.
In one case, AI tools in hospitals have misidentified conditions in Latino patients, leading to poorer outcomes. This underscores why AI fairness in healthcare must be a priority, as highlighted by experts at Yale, who warn that without intervention, technology could widen existing gaps.
Key Principles for Achieving AI Fairness in Healthcare
Inclusive and Representative Data Strategies
Start with the basics: Make sure your data reflects real-world diversity. This could mean partnering with community health centers to gather broader samples and actively correct imbalances. Actionable tip: Conduct regular data audits to spot and fix gaps before they affect outcomes.
What works well? Using AI fairness in healthcare frameworks to integrate voices from marginalized groups ensures no one is left out. For example, a developer might add synthetic data points to balance datasets, making algorithms more reliable across demographics.
Transparent Development and Governance Approaches
Transparency builds trust, so share your processes openly. This includes documenting decisions and inviting feedback from users. Have you thought about how open-source tools could help spot biases early? By doing this, you’re not just complying with ethics—you’re fostering innovation.
Best practice: Set up governance boards with diverse members to review AI systems. This principle of AI fairness in healthcare can prevent errors and promote accountability.
Continual Validation and Bias Audits
Don’t set it and forget it—regular testing is crucial. Run bias audits on updated datasets to maintain equity, especially as patient populations evolve. A simple step: Use metrics that track performance by demographic, flagging any disparities.
This ongoing process ensures AI fairness in healthcare adapts to new challenges, turning potential risks into strengths.
Stakeholder Engagement for Lasting Change
Bring everyone to the table: Clinicians, patients, and policymakers should collaborate on AI development. Host workshops to educate teams on bias recognition—it’s a game-changer. For instance, involving community leaders can tailor AI to local needs, making it more effective.
Through these efforts, we’re not just fixing problems; we’re creating opportunities for inclusive healthcare.
Ethical and Legal Considerations in AI Fairness in Healthcare
Beyond technical fixes, ethics play a huge role. Issues like patient consent and data privacy must be handled with care to avoid breaches. What if an AI decision can’t be explained? That’s a red flag for transparency.
Legally, regulations are evolving to hold developers accountable, ensuring AI fairness in healthcare protects rights. Policymakers are key here, as seen in reports from the HHS, pushing for safeguards against harm.
Opportunities and the Path Forward for AI Fairness in Healthcare
Despite the challenges, AI holds promise for closing care gaps. With the right safeguards, it can personalize treatments and detect diseases earlier in underrepresented groups. Here’s an idea: Use AI to analyze social determinants of health, making guidelines more adaptive.
The future looks bright if we commit to multidisciplinary teams and proactive measures. By prioritizing AI fairness in healthcare, we can turn technology into a force for good.
Frequently Asked Questions
What Causes Bias in Medical AI?
Bias often comes from non-representative data, flawed algorithms, or systemic inequities, as noted in key studies.
How Can We Reduce Bias in Healthcare Algorithms?
Focus on diverse datasets, regular audits, and stakeholder involvement to enhance AI fairness in healthcare.
What Are the Risks of Ignoring AI Bias?
It can lead to misdiagnoses and amplified disparities, undermining the very purpose of medical advancements.
Conclusion
Embracing AI fairness in healthcare is about creating a system where technology uplifts everyone equally. By implementing inclusive practices and ethical guidelines, we can minimize bias and foster better health for all. What steps can you take in your own work or community? Share your thoughts in the comments, explore more on our site, or connect with experts to keep the conversation going.
References
1. PMC Article on AI Fairness: Exploring AI Ethics in Healthcare
2. Harvard Study: Confronting Biases in AI
3. Yale Guidelines: Eliminating Racial Bias in AI
4. PMC Research: AI and Health Disparities
5. HHS Report: Algorithmic Bias in Healthcare
6. Additional PMC Study: Opportunities in Medical AI
7. Rutgers News: Perpetuating Bias Through AI
AI fairness in healthcare, medical AI bias, healthcare algorithms, health disparities, AI ethics, equitable AI in medicine, reducing bias in AI, AI in healthcare equity, ethical AI practices, AI fairness strategies