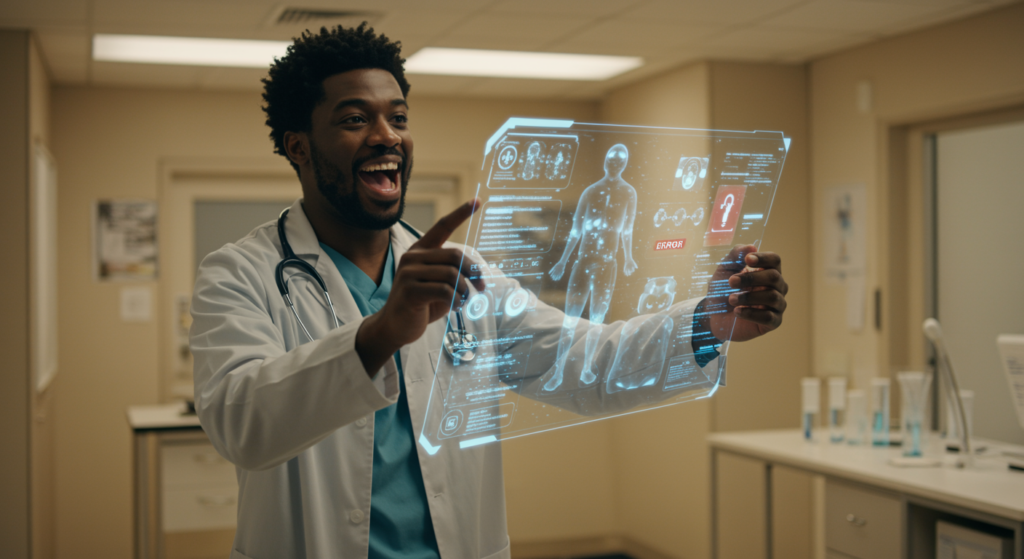
AI Medical Errors: Who Is Liable in Healthcare?
Understanding AI Medical Errors and Liability in Healthcare
Have you ever wondered what happens when AI medical errors lead to patient harm in hospitals? As artificial intelligence increasingly integrates into healthcare systems, AI medical errors raise urgent questions about liability for these failures. This complex intersection of technology and patient care involves healthcare professionals, institutions, technology developers, and patients, all navigating a shifting landscape of responsibility.
Think about it: AI tools, from diagnostic algorithms to treatment suggestions, are meant to enhance care, but when they falter, who steps up? In this section, we’ll dive into the core issues, exploring how AI medical errors can stem from design flaws, user mistakes, or systemic oversights, and why addressing them is crucial for safer healthcare.
The Rising Role of AI in Healthcare
AI is revolutionizing healthcare, stepping in for everything from spotting diseases early to managing medications, but it’s not without risks—like when AI medical errors occur in high-stakes situations. These systems promise to boost efficiency and cut down on human mistakes, potentially lowering malpractice claims by catching issues before they escalate.
For instance, imagine a busy ER where AI quickly analyzes scans to flag potential problems, freeing doctors to focus on patients. Yet, this innovation introduces new liability angles that traditional frameworks might overlook. According to a study from Stanford’s Human-Centered AI Institute (HAI, 2023), AI could reduce diagnostic errors by synthesizing vast data sets, but only if implemented thoughtfully.
Benefits of AI in Healthcare Settings
- Reducing repetitive tasks so healthcare pros can tackle more critical duties
- Minimizing errors driven by fatigue or bias, which is a game-changer in fast-paced environments
- Speeding up research via smart data analysis, leading to faster breakthroughs
- Enabling real-time patient monitoring to catch issues early and prevent AI medical errors from worsening
What’s exciting is how these benefits could transform daily routines—picture a nurse using AI to double-check dosages, avoiding costly oversights. But as we embrace this, we must weigh the potential for liability in AI medical errors, ensuring safeguards are in place.
The Complex Liability Ecosystem for AI Medical Errors
When AI medical errors result in harm, pinpointing blame isn’t straightforward—multiple parties often share the load. Factors like the error’s cause, human involvement, and context play key roles in determining accountability.
Healthcare Professionals’ Liability
Doctors and nurses might face liability if they misinterpret AI outputs or fail to explain its limitations to patients. For example, what if a physician ignores an AI warning due to overconfidence, leading to an AI medical errors-related injury? Malpractice suits could hold them responsible for not critically assessing recommendations, as highlighted in research from Milbank Quarterly (2020).
This puts practitioners in a tough spot, especially without specialized AI training. A relatable scenario: A doctor relies on an AI tool for a diagnosis but doesn’t verify it, only to face legal repercussions later—it’s a reminder that human judgment must complement tech.
Healthcare Institutions’ Responsibility
Hospitals adopting AI must set strong protocols to avoid AI medical errors, or they could be liable for lapses in oversight. If AI decisions involve human input and still go wrong, the institution might bear the brunt, as noted in a PMC article (2024).
Without clear regulations, these organizations grapple with uncertainty—think of a clinic rolling out new AI software without thorough testing, only to encounter errors. To mitigate this, they should prioritize robust guidelines and training to protect both patients and their own liability exposure.
Technology Developers and Manufacturers
AI creators could be held accountable for AI medical errors stemming from biased algorithms or poor data quality. If a system provides faulty advice due to flawed design, it might trigger widespread claims, affecting many patients at once.
Liable Party | Reason for Liability |
---|---|
Physician | Failure to understand AI information, not communicating limitations to patients, overlooking patient’s limited understanding |
Patient | Underestimating AI recommendations despite adequate information |
Developers/Producers | Erroneous AI recommendations, inadequate training, algorithmic biases |
Here’s a tip: Developers should rigorously test systems before launch to prevent such issues—after all, one error could ripple out, impacting trust in AI overall.
Legal Frameworks and Liability Challenges
The legal side of AI medical errors is still evolving, with courts struggling to apply old rules to new tech. In places like Europe, existing laws cover these under contract or product liability, but gaps remain.
Current Liability Regimes
Many jurisdictions rely on judges to define AI medical errors misuse, which is tricky when they’re not AI experts. Public unease adds to this—around 60% of Americans feel uneasy about AI in healthcare, per a Phoenix.edu survey (2023), making transparency vital.
Balancing Innovation and Safety in AI Medical Errors
How do we protect patients without stifling AI progress? New laws must weigh safety against innovation, allowing claims for harm while encouraging advancements. It’s about finding that sweet spot, where tech like AI can thrive without overwhelming liability fears.
Risk Factors and Concerns
AI brings benefits, but risks like AI medical errors from hidden flaws can escalate liability. Understanding these helps build better safeguards.
“Black Box” Problem
Some AI systems make decisions in ways even experts can’t explain, complicating accountability for AI medical errors. This opacity can lead to safety issues, as no one fully grasps why a recommendation went wrong.
Algorithmic Bias
Biases in AI training data might worsen inequalities, raising ethical and legal flags around AI medical errors. For example, if an algorithm overlooks certain demographics, it could result in unfair outcomes and lawsuits.
Training and Education Gaps
Many healthcare workers aren’t trained to handle AI outputs, increasing the chance of AI medical errors during rollout. Actionable advice: Invest in ongoing education to bridge this gap and reduce risks from the start.
Risk Management Strategies
To tackle AI medical errors, organizations can adopt smart strategies that blend tech and human elements.
Enhanced Training Programs
Offer tailored training to help staff evaluate AI recommendations and spot potential AI medical errors. This not only boosts confidence but also lowers liability through better preparedness.
Clear Documentation Protocols
Keep detailed records of AI use to create a clear trail for any disputes involving AI medical errors. It’s a simple step that can protect everyone involved.
Transparency in AI Implementation
Be upfront with patients about AI’s role, discussing both perks and pitfalls to build trust and avoid surprises related to AI medical errors.
Future Outlook
Despite challenges, AI could ultimately cut AI medical errors and improve outcomes, as experts predict. As regulations catch up, liability might become clearer, fostering safer innovations.
Regulatory Developments
Upcoming rules could clarify who handles AI medical errors, ensuring patient safety without halting progress. It’s an evolving field, but one that’s promising for healthcare’s future.
Conclusion
In the end, AI medical errors liability is a shared responsibility that demands careful navigation. By focusing on strong risk management, healthcare can reap AI’s rewards while protecting patients.
What are your thoughts on AI in healthcare—do you see it as a boon or a concern? We invite you to share your experiences in the comments, explore our related posts on medical tech innovations, or connect with us for more insights. Let’s keep the conversation going!
References
- HAI Stanford. (2023). Understanding Liability Risk in Healthcare AI. https://hai.stanford.edu/policy-brief-understanding-liability-risk-healthcare-ai
- Milbank Quarterly. (2020). Artificial Intelligence and Liability in Medicine. https://www.milbank.org/quarterly/articles/artificial-intelligence-and-liability-in-medicine-balancing-safety-and-innovation/
- PMC. (2024). Article on AI in Healthcare. https://pmc.ncbi.nlm.nih.gov/articles/PMC10800912/
- MedTech Europe. (2022). Liability Challenges in AI Medical Technologies. https://www.medtecheurope.org/wp-content/uploads/2022/10/medtech-europe_liability-challenges-in-ai-medical-technologies_document-paper_13-october-2022.pdf
- PlusWeb. (n.d.). AI in Healthcare: Risks and Benefits. https://plusweb.org/news/ai-in-healthcare-risks-and-benefits-for-medical-professional-liability/
- Phoenix.edu. (2023). Is AI Good or Bad for Society? https://www.phoenix.edu/blog/is-ai-good-or-bad-for-society.html
- HAI Stanford. (n.d.). Who’s Fault When AI Fails in Health Care? https://hai.stanford.edu/news/whos-fault-when-ai-fails-health-care
- PMC. (2024). Another Article on AI Liability. https://pmc.ncbi.nlm.nih.gov/articles/PMC11165650/
AI medical errors, healthcare liability, medical malpractice, artificial intelligence in healthcare, AI in medicine, medical liability risks, AI healthcare accountability, patient harm from AI, AI technology errors, liability in tech medicine