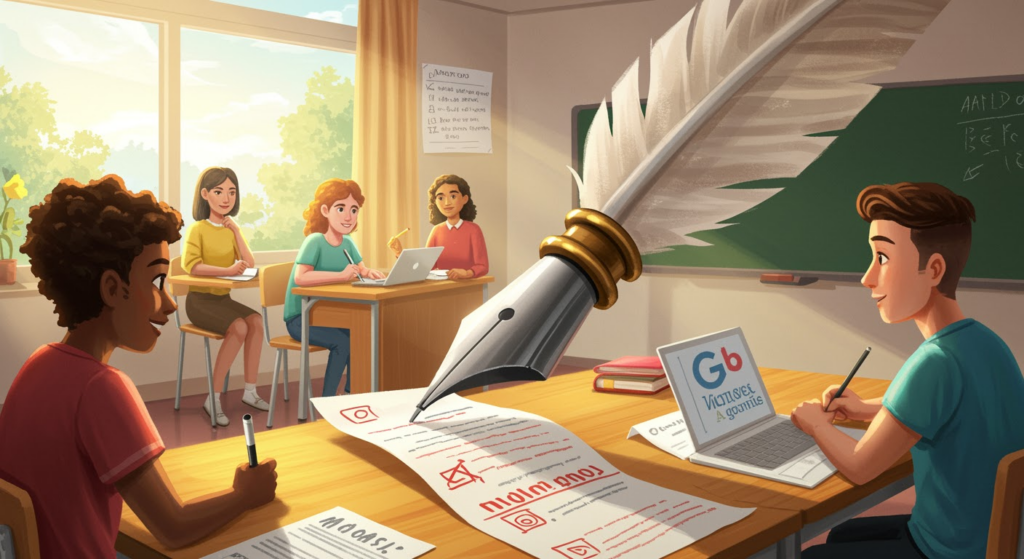
AI Bias in Grading Tools Favors Meta, Google, OpenAI
Understanding AI Bias in Grading Tools
AI bias in grading tools is emerging as a critical issue in education, where tools from companies like Meta, Google, and OpenAI are meant to streamline assessments but often fall short. Think about how these systems, powered by advanced algorithms, might unintentionally favor certain styles of writing or cultural perspectives, potentially skewing results for diverse students. As schools integrate these technologies, it’s essential to unpack how AI bias in grading tools can perpetuate inequalities and affect learning outcomes.
The Promise of AI-Assisted Marking
Generative AI tools, such as those from OpenAI, promise to make grading faster and more consistent, which is why they’re gaining traction in classrooms worldwide. For instance, imagine a teacher handling hundreds of essays with tools that apply rubrics uniformly, reducing the variability that human graders might introduce. Yet, even with benefits like improved consistency and standardization, AI bias in grading tools could undermine these advantages if not addressed properly.
Manifestations of Bias in AI Grading Systems
Inherent Limitations of Large Language Models
AI grading systems from OpenAI, Google, and Meta rely on large language models that sometimes generate inaccurate information or struggle with creative student responses. This can lead to biases that favor straightforward, conventional answers over innovative ones—what if your unique essay idea gets downgraded simply because it’s unconventional? These flaws highlight how AI bias in grading tools stems from the models’ inability to fully grasp nuanced human expression, affecting accuracy across platforms.
Ever noticed how AI might misinterpret cultural contexts? That’s a common pitfall, making it vital for educators to question these limitations before adoption.
Training Data Quality Issues
A major source of AI bias in grading tools is the quality of training data used by companies like Google and Meta, which often reflects societal inequalities. If datasets are skewed toward certain demographics, the tools might undervalue diverse perspectives, amplifying existing disparities in education. For example, a student from a underrepresented background could receive lower scores on essays that don’t align with the dominant narratives in the data.
Addressing this requires ongoing scrutiny, as biases absorbed from training sources can subtly influence grading decisions and widen educational gaps.
Comparative Analysis of Leading AI Grading Platforms
OpenAI’s Grading Capabilities
OpenAI’s models, like the o1 series, excel in complex tasks such as math and coding assessments, boasting impressive scores in evaluations. However, their focus on text-based grading means they might overlook visual elements, introducing another layer of AI bias in grading tools. What does this mean for students submitting multimedia projects? It’s a reminder that while OpenAI leads in reasoning, it has limitations that could favor certain assessment types.
Meta’s LLaMA 3.2 Approach
Meta’s LLaMA 3.2 stands out for its multimodal capabilities, handling text and images, which makes it more versatile than some competitors. Still, when it comes to specialized tasks, AI bias in grading tools from Meta might not match OpenAI’s precision in areas like advanced reasoning. Consider a scenario where a student’s visual artwork is graded fairly—Meta’s strengths could shine here, but inconsistencies in other domains persist.
This versatility is a step forward, yet it underscores the need for balanced evaluations to mitigate potential biases.
Google’s Assessment Solutions
Google’s Gemini 1.5 Pro is positioned as a top-tier option, though specific grading data is less publicized. Like other platforms, it grapples with AI bias in grading tools, particularly in how it processes varied content. If you’re an educator, you might wonder how Google’s approach compares—its integration with broader ecosystems could either enhance or complicate fairness in assessments.
Student Perceptions of AI-Graded Work
Research shows that students often view AI-graded work as fairer than human evaluations, thanks to perceived transparency. A study from Frontiers in Psychology found that students rated AI assessments higher in fairness, possibly because algorithms apply rules consistently. But does this mean AI bias in grading tools is overlooked? Not necessarily—while students appreciate objectivity, they still raise concerns about accuracy in subjective topics.
Have you ever felt more confident in a machine’s judgment? This trend suggests AI could build trust, but only if biases are minimized.
Ethical Concerns and Challenges
Replacing Human Educator Roles
AI bias in grading tools raises ethical questions about diminishing human involvement in education, as tools from Meta and Google might sideline teachers’ mentoring roles. For example, what happens to the personal feedback that inspires students when algorithms take over? Key issues include privacy and data ownership, which affect how these systems handle student information.
Educators can counteract this by staying involved, ensuring AI serves as a support rather than a substitute.
The Data Sustainability Problem
A growing challenge for AI grading tools from companies like OpenAI is the potential shortage of quality training data, which could exacerbate biases over time. Reports indicate that these models might exhaust human-generated content within a decade, limiting their ability to evolve and assess accurately. This sustainability issue could make AI bias in grading tools even more pronounced as educational needs change.
If left unaddressed, it might force a rethink of how we rely on these technologies for fair assessments.
Strategies for Mitigating AI Bias in Grading Tools
Developing Robust Safeguards
To tackle AI bias in grading tools effectively, institutions should prioritize diverse training datasets and regular audits of grading outcomes. Imagine setting up an appeals process where students can challenge unfair scores—what a game-changer that would be for equity. Tips like forming oversight committees can help ensure these tools are implemented responsibly.
Actionable advice: Start by reviewing your institution’s AI policies to incorporate bias checks early.
The Hybrid Assessment Approach
A hybrid model combining AI and human graders offers a practical way to reduce AI bias in grading tools while leveraging technology’s efficiency. For instance, AI could handle initial scoring, with teachers stepping in for nuanced reviews, creating a balanced system. This method not only maintains human insight but also allows for ongoing calibration to align standards.
By adopting this approach, educators can foster a more equitable learning environment, turning potential pitfalls into strengths.
The Future Landscape of AI in Educational Assessment
Looking ahead, the focus on transparency and ethical frameworks will shape how AI bias in grading tools is managed across companies like Google and Meta. Trends include specialized models for different subjects and rigorous bias testing before rollout. As competition drives innovation, we might see more tools that actively counteract these issues.
What innovations do you think will emerge? Staying informed is key to navigating this evolving field.
Conclusion: Balancing Innovation and Equity
AI bias in grading tools from Meta, Google, and OpenAI highlights the need for a careful balance between technological advancements and educational fairness. By implementing strategies like hybrid assessments and ongoing audits, we can harness these tools’ benefits without widening disparities. Remember, the goal is to create systems that support all students equally—something that requires collaboration and vigilance.
If you’ve experienced AI in your classroom, I’d love to hear your thoughts in the comments below. Share this post or explore more on AI ethics for deeper insights.
References
1. A study from AJET on AI in education: AJET Article.
2. NYU’s resources on generative tools: NYU FAQ.
3. OpenAI community discussion on bias: OpenAI Forum.
4. MIT’s analysis on AI grading: MIT Loaned Tech.
5. Frontiers in Psychology research: Frontiers Article.
6. Comparison of AI models: Walturn Insights.
7. YouTube discussion on AI: YouTube Video.
8. Fortune article on AI data bottlenecks: Fortune News.
AI bias in grading tools, educational assessment, OpenAI bias, Google AI grading, Meta AI tools, fairness in education, AI ethics in schools, hybrid grading systems, AI assessment challenges, reducing AI bias