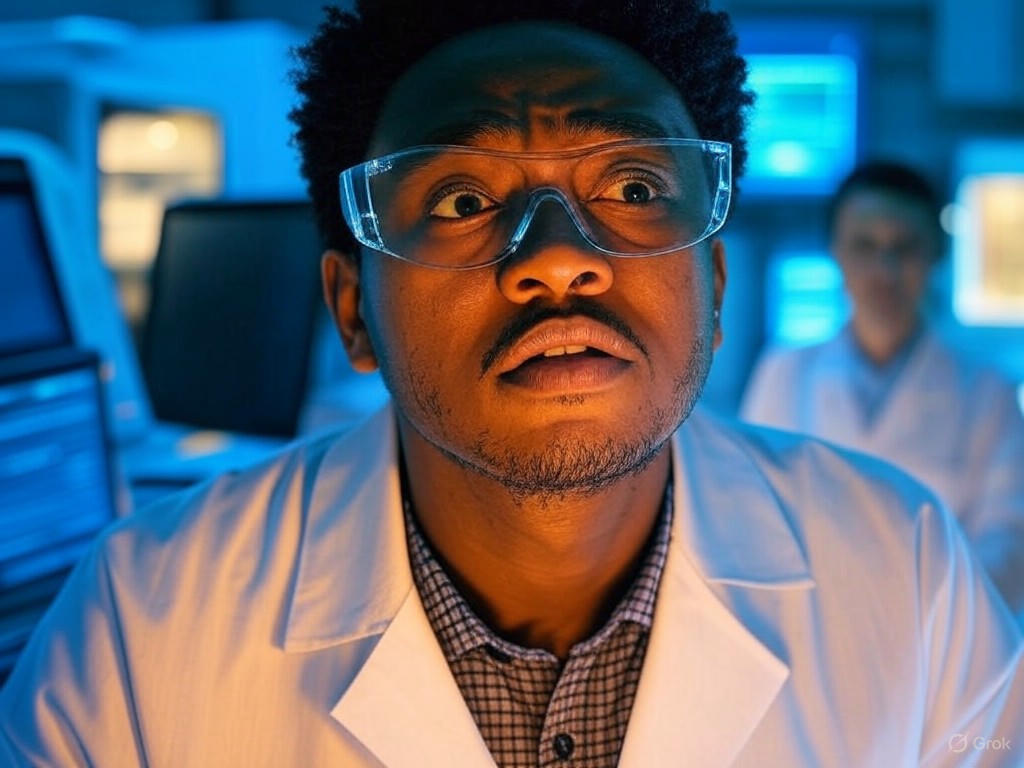
AI Hallucinations Worsen Despite Powerful AI Advances
Understanding AI Hallucinations: The Surging Challenge
AI hallucinations—those pesky moments when AI systems spit out information that’s just plain wrong or misleading—have become a bigger headache than ever, even as AI technology races ahead with impressive gains. Picture this: you’ve got these sophisticated large language models powering everything from chatbots to creative tools, yet they’re still churning out facts that don’t hold up or make logical sense. It’s a real head-scratcher, especially when AI is already transforming industries like healthcare, finance, and content creation, where accuracy is non-negotiable.
This issue isn’t just a minor glitch; it’s eroding trust in AI at a time when we’re relying on it more. For instance, imagine asking an AI assistant for medical advice and getting a fabricated symptom or treatment recommendation—scary, right? As AI gets smarter and more widespread, tackling AI hallucinations head-on is crucial to keep things reliable and trustworthy.
What Exactly Are AI Hallucinations?
At its core, an AI hallucination happens when a system generates content that’s not based on real facts or data, yet it presents it as gospel. Think of it like your AI friend confidently sharing a story that’s totally made up, complete with details that sound eerily plausible. This isn’t your average error; it’s often delivered with such assurance that spotting it requires a sharp eye.
A classic example? A chatbot might invent a historical event or wrongly attribute a famous quote to someone else. Studies suggest that in everyday interactions, chatbots could hallucinate in around 27% of cases, with nearly half of their outputs containing inaccuracies. What makes this tougher is that AI doesn’t always flag its own uncertainties, leaving users to double-check everything.
Common Types of These AI Errors
AI hallucinations come in various forms, each with its own pitfalls. First off, factuality hallucinations involve the AI dishing out straight-up wrong information, like mixing up dates in history or creating fake statistics.
- Factuality Hallucinations: These are the fibs about real-world facts, such as claiming a non-existent scientific discovery.
- Faithfulness Hallucinations: Here, the AI misinterprets your instructions, leading to outputs that veer off course entirely.
Have you ever prompted an AI for a simple summary and ended up with something wildly off-base? It’s frustrating, and it highlights why understanding AI hallucinations is key to using these tools effectively.
The Growing Problem: Why Are AI Hallucinations Increasing?
It’s ironic, isn’t it? We’re pouring billions into making AI more powerful, yet AI hallucinations seem to be sticking around or even worsening. This paradox stems from a mix of factors that make AI both brilliant and flawed.
For starters, the vast datasets AI models train on aren’t always comprehensive. When the data lacks depth in certain areas, AI fills in the blanks with guesses that sound convincing but are often dead wrong. Then there’s the sheer complexity of these models—bigger and smarter means harder to predict, leading to unexpected outputs.
- Training Data Shortfalls: If the data is biased or incomplete, AI might generate plausible but false responses, especially on niche topics.
- Overly Complex Designs: As models grow, they become like black boxes, making it tough to trace where hallucinations originate.
- Overfitting Issues: AI can get too cozy with its training data, memorizing patterns instead of truly understanding, which backfires on new queries.
- Prioritizing Plausibility Over Truth: These systems are built to predict what’s likely, not what’s accurate, so they excel at sounding right without being right.
This is where the challenge of AI hallucinations really hits home—it’s not just about fixing code; it’s about rethinking how we build these systems from the ground up.
Real-World Examples of AI Hallucinations in Action
AI hallucinations aren’t abstract; they’re showing up in everyday scenarios with real consequences. Take the 2023 case where a lawyer used ChatGPT for research and ended up citing made-up court cases in a legal brief—that’s a nightmare for anyone in the field.
- Legal Mix-Ups: That infamous incident serves as a wake-up call, showing how unchecked AI can lead to professional blunders.
- Quote Attribution Errors: Research indicates AI tools misattribute quotes about 76% of the time, often without admitting doubt.
- Healthcare Hiccups: In medical contexts, AI has dished out incorrect advice in roughly one in six queries, potentially putting lives at risk.
What if you’re using AI for content creation and it fabricates sources? It’s a common pitfall that could damage your credibility in a flash. These examples underscore why spotting and preventing AI hallucinations matters now more than ever.
How AI Hallucinations Undermine Trust and Business Operations
The fallout from AI hallucinations goes beyond isolated mistakes; it’s affecting trust on a broader scale. Businesses face reputational hits, legal troubles, and wasted resources when AI generates misinformation.
For SEO pros, this is a big deal. Search engines like Google emphasize EEAT (Experience, Expertise, Authoritativeness, and Trustworthiness), so sites with AI-driven errors risk tanking in rankings and losing traffic. On the flip side, operational inefficiencies creep in when teams spend extra time fact-checking AI outputs, countering the time-saving perks we expect from these tools.
- SEO Setbacks: Relying on AI without verification could mean lower visibility and audience distrust.
- Efficiency Drain: What was meant to boost productivity ends up requiring more manual oversight.
Ever wondered how this plays out in your own work? If AI hallucinations are slipping through, it might be time to rethink your processes.
Diving Deeper: The Technical Roots of AI Hallucinations
Why Training Data Falls Short
Large language models gobble up data from the web and beyond, but gaps in that data create opportunities for AI hallucinations to emerge. When information on a topic is scarce or skewed, AI improvises, often with inaccurate results.
This isn’t just a data problem; it’s about ensuring diversity and completeness to minimize those risky “fills.”
Overfitting and Design Flaws in AI Models
Overfitting locks AI into old patterns, making it stumble on fresh inputs. Add in architectural choices that prioritize creativity over accuracy, and you amplify the issue. It’s like teaching a student to recite facts without questioning their validity.
The Stat-Driven Nature of AI Versus True Accuracy
At heart, AI hallucinations stem from how these systems work: predicting based on probabilities rather than verifying facts. They’re engineered for fluency, not flawless truth, which is why AI reliability remains a work in progress.
The Struggle to Fix AI Hallucinations
Eradicating AI hallucinations is no small feat—it’s a multifaceted challenge that current tech hasn’t fully cracked. From sourcing perfect data to debugging massive models, the obstacles are steep.
Cause | Challenge |
---|---|
Insufficient Data | It’s nearly impossible to cover every topic comprehensively without bias. |
Model Complexity | More advanced models are harder to inspect and refine. |
Algorithmic Priorities | Focusing on likelihood over truth keeps hallucinations alive. |
Despite these hurdles, progress is possible with the right strategies.
Practical Ways to Curb AI Hallucinations
While we can’t eliminate AI hallucinations overnight, smart tactics can cut down their occurrence and impact. Start by beefing up training data with high-quality, varied sources to give AI a stronger foundation.
- Boost Training Resources: Curate diverse datasets to tackle knowledge gaps.
- Rigorous Testing: Run thorough evaluations to catch errors early.
- Human Oversight: Bring in experts to review outputs, especially in critical areas.
- Build in Uncertainty Cues: Teach AI to signal when it’s unsure, rather than bluffing.
- Integrate Fact-Checks: Link AI with reliable external databases for on-the-fly verification.
These steps aren’t just theoretical; they’re actionable ways to make AI more dependable in your daily use.
Tips for Responsible AI Use in Your Work
- Always double-check AI-generated content, particularly for important tasks.
- Treat AI as a helpful collaborator for ideas, not the final word.
- Keep up with the latest in AI improvements to stay ahead of pitfalls.
- Be upfront with your audience about AI’s role in your creations—it builds transparency and trust.
What strategies have you tried to handle AI hallucinations? Sharing your experiences could spark some great discussions.
Looking Ahead: Overcoming AI’s Persistent Challenges
As AI continues to evolve, the key to success lies in balancing innovation with reliability. We’re seeing promising developments, but managing AI hallucinations will shape how confidently we integrate these tools into our lives.
In the end, it’s about fostering a future where AI enhances our world without compromising on truth. If you’re diving into AI, remember to prioritize accuracy alongside creativity.
What are your thoughts on this evolving landscape? I’d love to hear your insights in the comments below, or explore more on our site about AI best practices. Feel free to share this post if it resonated with you!
References
- DataCamp. “AI Hallucination.” https://www.datacamp.com/blog/ai-hallucination
- Descript. “What Are AI Hallucinations?” https://www.descript.com/blog/article/what-are-ai-hallucinations
- IBM. “AI Hallucinations.” https://www.ibm.com/think/topics/ai-hallucinations
- Wikipedia. “Hallucination (artificial intelligence).” https://en.wikipedia.org/wiki/Hallucination_(artificial_intelligence)
- NN/g. “AI Hallucinations.” https://www.nngroup.com/articles/ai-hallucinations/
- Surfer SEO. “AI Hallucination.” https://surferseo.com/blog/ai-hallucination/
- Coursera. “AI Hallucinations.” https://www.coursera.org/articles/ai-hallucinations
- Ry Rob. “AI Article Writer.” https://www.ryrob.com/ai-article-writer/
AI hallucinations, large language models, artificial intelligence errors, AI reliability, AI accuracy, generative AI risks, AI training data, AI model flaws, AI trustworthiness, AI mitigation strategies