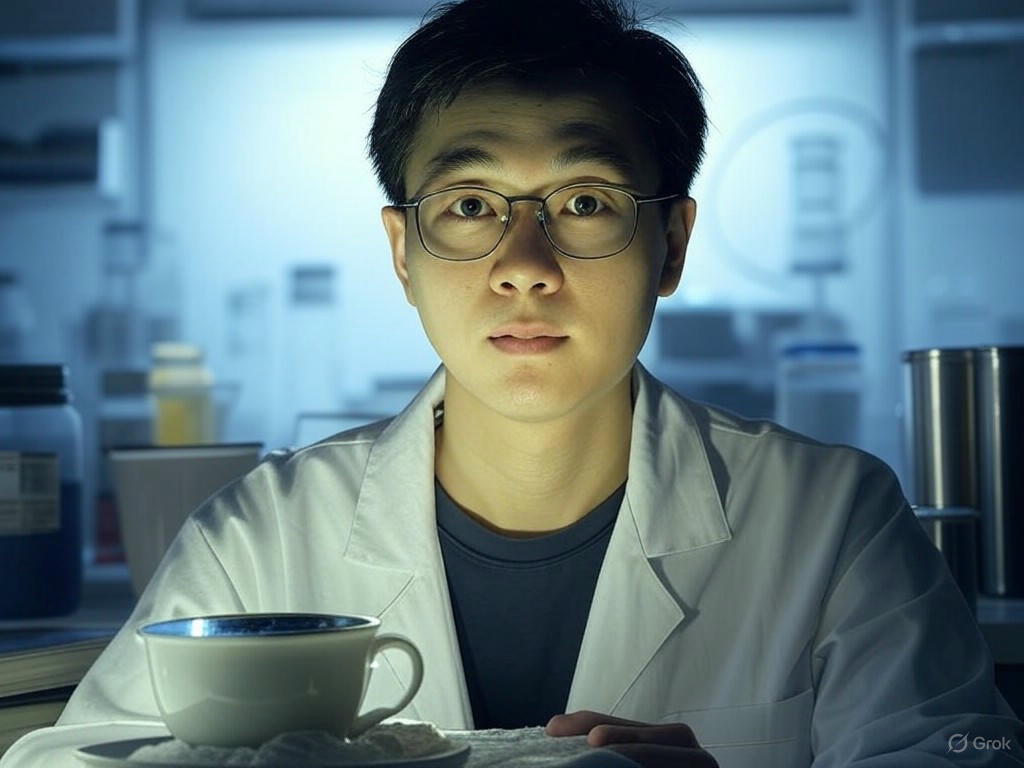
AI Hallucinations: Causes, Prevention Strategies and Solutions
What Are AI Hallucinations?
Have you ever wondered why your AI assistant sometimes spins a tale that sounds spot-on but turns out to be completely off-base? AI hallucinations are those moments when systems like large language models confidently dish out misinformation that seems credible. It’s like the AI is filling in blanks with its own creative twists, drawing from patterns it “thinks” are real, even if they’re not.
At its core, this phenomenon involves AI confabulation, where the model generates plausible but incorrect outputs. Think of it as the digital equivalent of a vivid dream—harmless in fiction, but risky in real-world applications. By understanding AI hallucinations early, we can start building more reliable tech that supports better decisions without the guesswork.
The Growing Concern of AI Hallucinations
AI hallucinations aren’t just a quirky glitch; they’re becoming more common as we rely on these tools daily. Studies suggest that chatbots powered by large language models might get it wrong up to 27% of the time, with factual errors creeping into nearly half of all outputs. Imagine basing a business decision on fabricated data—could lead to costly mistakes, eroded trust, or even legal headaches.
This issue hits hard in fields like healthcare or finance, where accuracy is non-negotiable. How do we ensure AI doesn’t mislead us? It’s a question worth pondering as these systems integrate deeper into our lives, potentially amplifying risks if left unchecked.
Exploring the Causes of AI Hallucinations
Diving into why AI hallucinations happen reveals a mix of technical flaws and data pitfalls. Often, it’s tied to how models are built and trained, making prevention more achievable once we pinpoint the problems. Let’s break this down to see what really drives these errors.
Key Triggers Behind AI Hallucinations
One major culprit is incomplete or biased training data—think of feeding an AI a diet of outdated facts, and it starts serving up skewed results. For instance, if a model learns from sources riddled with stereotypes, it might perpetuate those in its responses, leading to unreliable outputs.
Poor data classification adds to the chaos, where mislabeled information confuses the AI’s learning process. Overfitting is another factor; the model gets so fixated on its training examples that it struggles with anything new, almost like cramming for a test and forgetting how to apply knowledge in real life.
- Underfitting: When models are too simplistic, they miss subtle details and end up fabricating connections that aren’t there.
- Prompt ambiguity: Vague queries leave room for AI to guess, turning a simple question into a web of inventions.
- Lack of real-world context: Without ways to verify information, AI hallucinations thrive in a vacuum, spitting out confident errors.
Ever tried asking a chatbot about a niche topic without specifics? You might get a creative—but wrong—answer. Recognizing these causes of AI hallucinations is the first step toward fixing them.
Varied Forms of AI Hallucinations
AI hallucinations aren’t limited to text; they pop up across different AI types, making them a versatile problem. In textual scenarios, like with ChatGPT, you might get eloquent but fabricated stories that sound convincing.
- Visual hallucinations: Picture an AI misidentifying objects in an image, turning a harmless photo into something entirely different.
- Auditory hallucinations: Speech models could twist words or invent dialogue, potentially misleading voice assistants.
These variations show how AI hallucinations can sneak into everyday tech, from social media filters to automated customer service. Spotting them early could save a lot of trouble.
Risk Factors Amplifying AI Hallucinations
Why do some AI models hallucinate more than others? It’s often due to underlying risk factors that compound errors. Here’s a quick overview in a table to highlight the main issues:
Risk Factor | Description | Potential Impact |
---|---|---|
Data Quality | Issues like outdated or biased datasets | Reinforces misinformation, such as amplifying stereotypes in recommendations |
Model Complexity | Overly complex or simplistic designs | Leads to poor generalization, where AI hallucinations occur in unfamiliar scenarios |
Ambiguous Prompts | Unclear user inputs | Triggers speculative responses, making AI hallucinations more frequent |
No Verification | Absence of fact-checking tools | Allows erroneous outputs to spread unchecked, eroding trust |
Addressing these factors head-on can significantly cut down on AI hallucinations and boost overall system performance.
Strategies for Preventing AI Hallucinations
Thankfully, there are practical ways to tackle AI hallucinations before they escalate. From data improvements to smarter design, these approaches make AI more dependable. Let’s explore how to put them into action.
Data-Centric Prevention Tactics
Start with your data—it’s the foundation of any AI system. Using high-quality, verified sources helps eliminate the breeding ground for AI hallucinations. For example, curate datasets from reliable outlets and weed out biases to ensure balanced learning.
- Regularly audit data for gaps and inconsistencies.
- Adopt standardized templates to keep everything organized and reduce errors.
This not only prevents AI hallucinations but also makes your model more adaptable over time.
Refining Model Design and Prompts
Optimizing model complexity is key; aim for a balance that avoids overfitting while capturing essential patterns. Craft prompts with precision—think specific instructions that guide the AI without leaving room for guesswork.
Adding contextual anchoring, like providing background in queries, can steer responses away from AI hallucinations. It’s like giving your AI a clear map instead of a vague direction.
Incorporating Human Oversight
Humans bring the intuition that machines lack, so integrating them into the process is a game-changer. Regular reviews by experts can catch and correct potential AI hallucinations before they go live.
- Use cross-model comparisons to validate outputs across different systems.
This collaborative approach ensures more accurate results and builds confidence in AI tools.
Fostering Continuous Improvement
AI isn’t static; it evolves with feedback. Iterative updates using fresh, accurate data help minimize AI hallucinations over time. Set up user reporting systems so people can flag issues, turning real-world interactions into learning opportunities.
What if every error reported led to a smarter model? That’s the power of ongoing refinement.
Innovative Solutions to Combat AI Hallucinations
The fight against AI hallucinations is gaining momentum with cutting-edge innovations. Hybrid systems, for instance, pair language models with fact-checkers for instant verification, much like having a built-in editor.
- Enhance explainability so users can see the reasoning behind outputs.
- Implement transparency tools for audit trails and source links.
- Fine-tune models for specific domains with expert-curated data to reduce errors in targeted areas.
These advancements are making AI hallucinations less of a threat, paving the way for safer applications.
The Path to More Trustworthy AI
As AI weaves into more aspects of life, from business decisions to creative work, minimizing AI hallucinations is crucial for trust. While we can’t erase them entirely, strategies like robust data practices and human checks can make a real difference.
Imagine a future where AI supports us without second-guessing its facts— that’s the goal we’re working toward. By staying vigilant, we can harness AI’s potential responsibly.
Essential Insights on AI Hallucinations
To wrap up, AI hallucinations pose real challenges but are manageable with the right tactics. They stem from data flaws, prompt issues, and model limitations, yet prevention through quality controls and innovation keeps them in check.
- Key to success: Prioritize reliable data and human input for trustworthy AI outcomes.
- Emerging tools in AI hallucinations research are boosting transparency and accuracy.
By tackling these head-on, we create AI that’s not only powerful but dependable.
References
For deeper insights, here are the sources used:
- IBM. “AI Hallucinations.” IBM.com
- Wikipedia. “Hallucination (artificial intelligence).” en.wikipedia.org
- DataScientest. “Understanding AI Hallucinations: Causes and Consequences.” datascientest.com
- SAS. “What Are AI Hallucinations?” sas.com
- TechTarget. “AI Hallucination Definition.” techtarget.com
- DigitalOcean. “What is AI Hallucination?” digitalocean.com
- SEOWind. “AI Content for SEO.” seowind.io
- MIT Sloan. “Addressing AI Hallucinations and Bias.” mitsloanedtech.mit.edu
Ready to dive deeper into making AI more reliable? Share your experiences with AI hallucinations in the comments below, or check out our related posts on trustworthy AI practices. Let’s keep the conversation going!
AI hallucinations, large language models, AI confabulation, hallucination prevention, trustworthy AI, AI errors, LLM reliability, AI data bias, preventing AI mistakes, AI innovation