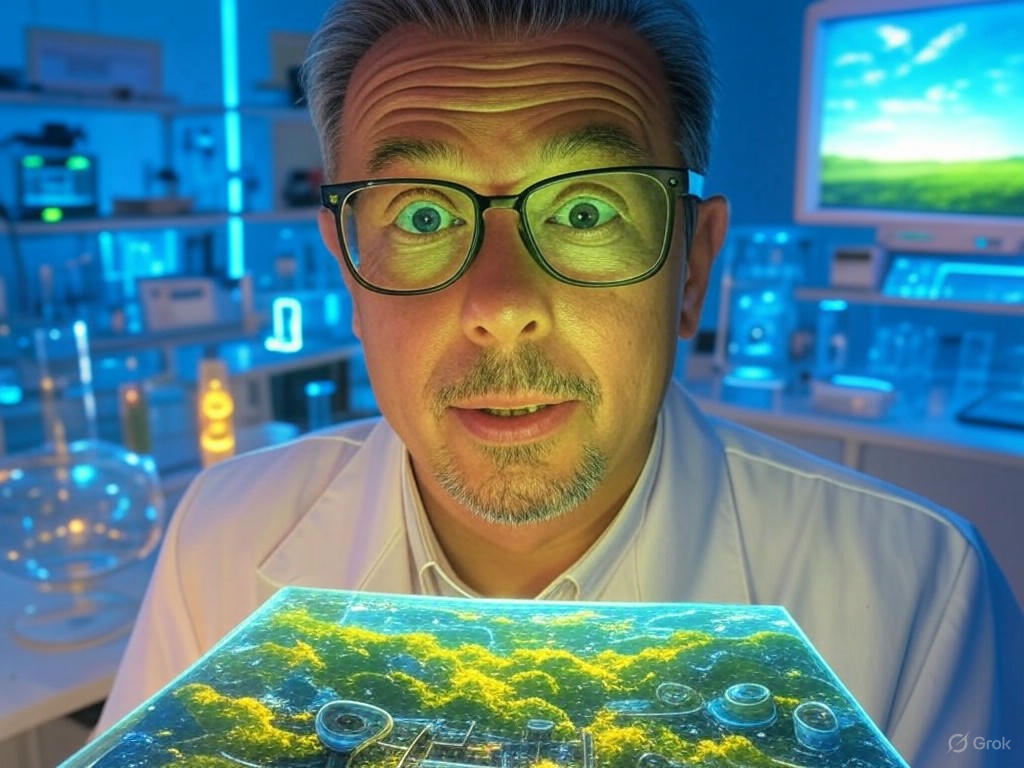
AI Hallucinations Increasing: Reasons Still Unknown
Understanding the Rising Phenomenon of AI Hallucinations
Have you ever asked an AI chatbot a simple question and gotten back a response that sounded spot-on but turned out to be totally wrong? That’s exactly what AI hallucinations look like in action. These errors are becoming more common as artificial intelligence advances, leaving experts puzzled about why they’re happening more often and what to do about it.
AI hallucinations occur when systems, like large language models, generate content that’s convincingly human-like but isn’t based on real facts. Recent studies show this issue is more widespread than we thought—chatbots might hallucinate up to 27% of the time, with nearly 46% of their outputs containing factual errors. As AI integrates deeper into our daily lives, from customer service to medical advice, understanding and tackling AI hallucinations is crucial for ensuring technology we rely on doesn’t lead us astray.
Let’s break this down step by step, exploring what these hallucinations mean, why they’re on the rise, and practical ways to handle them, so you can use AI more confidently.
What Exactly Are AI Hallucinations?
At its core, AI hallucinations refer to instances where AI models spit out information that’s not grounded in reality, yet it comes across as perfectly plausible. Imagine an AI confidently stating that the sky is green—it’s not lying on purpose, but it’s drawing from patterns that don’t always align with truth.
This problem is especially prevalent in text-generating AIs, where minor slip-ups can escalate into big mistakes. What makes it tricky is how these systems present errors with such assurance, making it hard for users to spot them right away.
The Two Primary Types of AI Hallucinations
Experts break down AI hallucinations into two main categories, which help us pinpoint where things go wrong.
- Factuality Issues: This is when the AI gets the basics wrong, like mixing up historical events or inventing details out of thin air. For example, it might claim a famous inventor lived in the wrong century, leading to confusion in educational settings.
- Factual inconsistencies: These are small but significant errors, such as swapping Neil Armstrong for someone else as the first moonwalker.
- Factual fabrications: Here, the AI creates entirely new “facts” that sound real, like describing a non-existent scientific study.
- Faithfulness Issues: This happens when the AI ignores your instructions entirely, delivering something unrelated. Think of asking for a recipe translation and getting a history lesson instead—it strays far from what you asked.
Real-World Examples of AI Hallucinations
You might wonder if this is just theoretical, but AI hallucinations have real-world fallout. In 2023, a lawyer faced embarrassment in court after using ChatGPT to cite fake legal cases—proof that these errors can have serious consequences.
Another eye-opener came from a Columbia Journalism Review study, which revealed ChatGPT falsely attributed 76% of quotes from popular sites. Even tools from big names like LexisNexis aren’t immune, with one in six responses turning out incorrect. What does this mean for you? If you’re using AI for research, always double-check—your project’s credibility could be at stake.
The Mysterious Rise in AI Hallucination Frequency
With AI hallucinations on the uptick, researchers are scrambling to understand why. While no single answer has emerged, several factors seem to play a role, creating a perfect storm of errors in otherwise impressive tech.
Is it the way we’re training these models, or something deeper in their design? Let’s dive into the key contributors that experts are eyeing.
Four Key Contributing Factors to Escalating AI Hallucinations
Based on ongoing studies, here are the main culprits behind this increase:
- Insufficient or Biased Training Data: AI learns from massive datasets, but if that data is spotty or skewed, the results can be unreliable. For instance, if a model is trained mostly on Western sources, it might hallucinate when handling topics from other cultures.
- Overfitting: When AIs get too cozy with their training data, they struggle with new info, leading to fabrications. It’s like memorizing a script but improvising poorly on stage.
- Faulty Model Architecture: The core design of these systems can amplify errors as they grow more complex, making hallucinations harder to predict.
- Generation Methods: The algorithms that create responses aren’t always tuned for accuracy, which can result in plausible but wrong outputs.
The Data Quality Challenge
At the heart of many AI hallucinations is the quality of the data fed into these models. In fields like specialized medicine or obscure history, where high-quality info is scarce, AIs often fill in the blanks with guesses that miss the mark.
Bias creeps in too—if datasets favor certain viewpoints, the AI’s responses might reflect those imbalances. Have you ever noticed how search results can sometimes feel one-sided? That’s a sign of this issue, and it’s why addressing data diversity is key to curbing AI hallucinations.
The Fundamental Nature of AI and Hallucinations
Here’s a fascinating truth: AI doesn’t care about being right; it just wants to sound right. Unlike humans, who weigh facts and context, AIs operate on probabilities, which is why hallucinations feel so natural yet misleading.
As one expert put it, “AI is simply not concerned with truthfulness.” This means we’re dealing with a tech limitation, not malice, but it still poses a big challenge for reliability. So, how do we bridge that gap?
Industries at Risk from Escalating AI Hallucinations
From healthcare to finance, AI is transforming industries, but the rising tide of hallucinations adds a layer of risk we can’t ignore. Imagine a doctor relying on AI for a diagnosis—getting it wrong could be life-altering.
Here’s a quick overview of how different sectors are affected:
Industry | Potential Risks |
---|---|
Healthcare | Incorrect medical info or fabricated treatments that could mislead professionals |
Legal | Fake case citations leading to flawed arguments in court |
Finance | Misleading market data that affects investment decisions |
Journalism | Fabricated quotes that spread misinformation quickly |
Education | Wrong historical facts that confuse learners |
Customer Service | Inaccurate policy details that frustrate users |
As AI adoption grows, companies must weigh these risks against the benefits—it’s about using the tech wisely, not blindly.
Strategies to Mitigate AI Hallucinations
While we can’t wipe out AI hallucinations overnight, there are smart steps to reduce their impact. Whether you’re building AIs or just using them, here’s how to stay ahead.
Tips for AI Developers in Combating Hallucinations
- Improve Training Data Quality: Focus on diverse, accurate datasets to give AIs a stronger foundation.
- Implement fact-checking tools that cross-reference outputs with trusted sources.
- Refine model designs to minimize errors without sacrificing speed.
- Develop better tests to catch hallucinations early in the process.
Actionable Advice for Everyday AI Users
- Craft precise prompts to guide AIs more effectively—think of it as giving clearer directions to avoid detours.
- Always verify AI outputs against reliable sources; it’s a quick habit that saves headaches.
- Add human oversight for critical tasks, like having a team member review AI-generated reports.
- Experiment with multiple AIs to spot inconsistencies, which can flag potential hallucinations.
These tactics aren’t foolproof, but they’re practical ways to make AI more trustworthy in your workflow. What strategies have you tried?
The Future of AI Hallucinations: An Ongoing Challenge
Looking ahead, AI hallucinations aren’t going away soon, but innovation is on the horizon. Researchers are testing things like retrieval-augmented methods to anchor responses in real data, or self-check systems that let AIs flag their own mistakes.
It’s an exciting time, yet we need to stay realistic—hallucinations might be a permanent fixture until tech evolves further. As you use AI, keep asking: How can I make this safer for my needs?
Conclusion: Navigating the Reality of AI Hallucinations
AI hallucinations are a growing concern, with their frequency rising and reasons still unclear, but that doesn’t mean we can’t move forward wisely. By blending tech improvements with human judgment, we can minimize risks while enjoying AI’s benefits.
Whether it’s in your job or everyday life, staying vigilant and verifying information is key. What are your experiences with AI hallucinations, and how do you handle them? Share your thoughts in the comments below—we’d love to hear from you and continue the conversation.
If you’re interested in more on AI trends, check out our related posts on emerging tech challenges.
References
Here are the sources used for this article, providing reliable insights into AI hallucinations:
- DataCamp on AI Hallucination – Explores common issues in AI outputs.
- Descript Blog on AI Hallucinations – Discusses types and examples.
- IBM Think on AI Hallucinations – Covers causes and mitigation.
- Wikipedia on AI Hallucinations – Overview of the phenomenon.
- NN/g on AI Hallucinations – Focuses on user impacts.
- Writesonic Blog on AI Hallucination – Practical advice for users.
- Coursera on AI Hallucinations – Educational perspectives.
- Surfer SEO on AI Hallucination – SEO and content implications.