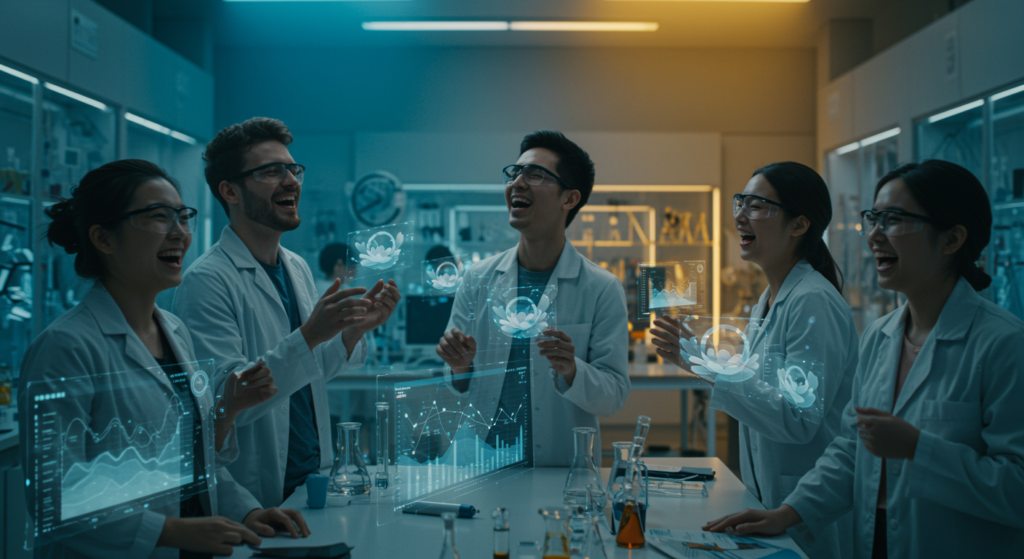
AI Exponential Growth: Doubling AI Task Complexity Implications
Introduction
Have you ever wondered how quickly technology can outpace our expectations? AI task complexity is doubling roughly every seven months, transforming everything from daily routines to global industries. This rapid evolution isn’t just about faster computers; it’s about AI systems handling more intricate problems, boosting efficiency, and sparking new opportunities for innovation. As we dive into this trend, let’s explore what it means for businesses, researchers, and everyday users alike.
Drivers Behind the Exponential Growth in AI Task Complexity
The surge in AI task complexity stems from a perfect storm of advancements. Affordable energy and state-of-the-art hardware, like powerful GPUs, are making it easier to process massive datasets in the cloud. For instance, companies are building sprawling data centers that crunch numbers at speeds unimaginable just a decade ago.
Breakthroughs in algorithms are another key factor, with researchers continually refining models to adapt and learn more effectively. Picture this: a startup using these smarter algorithms to predict market trends with pinpoint accuracy, giving them an edge over competitors. Capital investment from tech giants fuels this fire, drawing in top talent and accelerating development cycles.
Meanwhile, the flood of data from smartphones, sensors, and online interactions provides endless fuel for AI training. Accelerated data processing through cloud infrastructure means complex models can be trained in days rather than months. All these elements create a feedback loop, where each leap in AI task complexity paves the way for the next.
Current AI Capabilities and the Barriers of AI Task Complexity
Understanding Limits in Task Duration and AI Task Complexity
Even with these gains, AI task complexity hits roadblocks in real-world applications. Top AI agents can handle short tasks flawlessly, but when projects stretch beyond a few minutes—say, mimicking a human expert’s four-hour effort—success rates plummet to under 10%. This limitation often stems from AI’s struggle with prolonged sequences, where even simple steps add up to overwhelming challenges.
Imagine an AI trying to plan a full marketing campaign; it might excel at individual elements like data analysis but falter when adapting to unexpected changes. Performance dips not from memory constraints, but from issues in logical reasoning and mid-process adjustments. As AI task complexity grows, overcoming these barriers will be crucial for broader adoption.
Navigating Human Collaboration Amid Rising AI Task Complexity
As tasks become more demanding, the partnership between humans and AI evolves in fascinating ways. Higher AI task complexity means people rely more on these tools for handling information overload, like sifting through thousands of emails to spot trends. Yet, this dependence can erode trust if users overlook subtle errors in complex outputs.
For example, in healthcare, doctors might use AI to analyze patient data, but they need clear explanations to verify recommendations. Building that trust requires transparent AI systems, especially in high-stakes fields. So, how can we ensure humans and AI work together without one overshadowing the other?
The Rise of Agentic AI in Tackling AI Task Complexity
One of the most exciting developments is agentic AI, which empowers systems to act autonomously, planning and reasoning like never before. These agents can dissect AI task complexity by breaking down massive projects into manageable sub-tasks, such as reviewing documents or refining strategies on the fly.
Think of an agentic AI as your personal research assistant: it could summarize a mountain of reports in hours, highlighting contradictions that might take weeks for a human team. In practice, this means AI agents functioning as junior developers, accelerating innovation by compressing timelines from months to days. As AI task complexity continues to escalate, agentic systems could redefine productivity across sectors.
Contrasting Traditional AI with Agentic Approaches
To appreciate the shift, let’s compare the two. Traditional AI excels in straightforward, repetitive tasks, but it lacks the flexibility to handle evolving AI task complexity on its own.
Aspect | Traditional AI | Agentic AI |
---|---|---|
Task Scope | Narrow, focused applications | Broad, self-managing for complex scenarios |
Adaptability | Limited to predefined rules | Highly flexible, with real-time error fixes |
Human Oversight | Needs constant guidance | Requires only occasional checks |
Impact | Small efficiency boosts | Major transformations in workflows |
This evolution isn’t just technical; it’s about empowering AI to tackle AI task complexity with minimal human input, opening doors to more autonomous operations.
Industry-Wide Implications of Doubling AI Task Complexity
The ripple effects of increasing AI task complexity are profound, touching everything from business operations to creative fields. In corporate settings, AI can slash analysis time from months to days, helping companies forecast trends and outmaneuver rivals—but it demands new skills from managers to oversee these tools effectively.
In healthcare, AI agents might integrate patient records with research papers to suggest personalized treatments, potentially saving lives through faster diagnostics. Research and development teams benefit too, as AI dynamically pulls from vast knowledge bases, cutting error rates in complex projects. Even in content creation, AI streamlines SEO tasks, like generating optimized articles based on user behavior data.
However, this growth isn’t without costs; industries like energy face skyrocketing demands for computational power. A hypothetical scenario: a data center’s electricity use doubles annually, pushing companies toward sustainable solutions. What strategies can businesses adopt to balance innovation with environmental responsibility?
Sustainability Challenges from Escalating AI Task Complexity
As AI task complexity rises, so does its energy footprint, with training models consuming vast amounts of electricity. This exponential demand is straining resources, from increased operational costs to higher carbon emissions. For instance, a single AI model’s training can rival the energy use of a small town over a year.
To counter this, developers are focusing on energy-efficient architectures and optimized processes that reduce waste without sacrificing performance. Investing in renewable energy for data centers is another smart move, ensuring that the push for greater AI task complexity doesn’t harm the planet. Ultimately, sustainable AI isn’t just an option—it’s essential for long-term viability.
Balancing Trust and Human Roles in the Face of AI Task Complexity
With AI handling more intricate tasks, maintaining trust becomes paramount. Users might second-guess decisions when AI task complexity outstrips their ability to verify outcomes, leading to over-reliance or skepticism. Simple, clear explanations from AI systems can bridge this gap, helping people understand and trust the results.
Organizations should prioritize AI literacy training, equipping teams to collaborate effectively. Here’s a tip: start with small pilots, like using AI for routine data analysis, to build confidence gradually. By fostering this balance, we can enhance human-AI partnerships rather than replace them.
The Future Landscape of AI Task Complexity
Looking ahead, AI task complexity is set to expand even further, blurring lines between human and machine capabilities. In creative domains, AI is already generating art or code that rivals experts, raising questions about collaboration and ethics. This trajectory promises exciting opportunities, like automating mundane tasks to free up time for innovative work, but it also brings risks, such as job displacement or ethical dilemmas.
To thrive, businesses must assess how AI can integrate into their processes—perhaps by adopting agentic tools for project management. Building AI-fluent teams and monitoring sustainability will be key. What steps are you taking to prepare for this shift?
Wrapping Up and Next Steps
The doubling of AI task complexity is reshaping our world, offering tools for greater efficiency and creativity while demanding careful management of trust and resources. By embracing these changes thoughtfully, we can unlock a future where AI amplifies human potential. If this topic sparks your interest, I’d love to hear your thoughts in the comments below—share your experiences or ideas on how AI is impacting your work. For more insights, check out our related posts on AI innovations.
References
- Perplexity AI. “AI Task Capacity Doubles Every Seven Months.” Link
- ML Science. “Exponential Growth in AI.” Link
- Dornanoco Substack. “The Great AI Discontinuity.” Link
- RAND Corporation. “Research Report on AI Implications.” Link
- Sidecar AI. “Moore’s Law Accelerated.” Link
- ACM Digital Library. “Human-AI Collaboration Study.” Link
- SEO Vendor. “AI for Content Excellence.” Link
- The Science Dev. “Exponential Growth of AI Computation.” Link
AI task complexity, AI exponential growth, agentic AI, computational power, AI implications, AI capabilities, sustainable AI, human-AI collaboration, AI task barriers, AI innovation