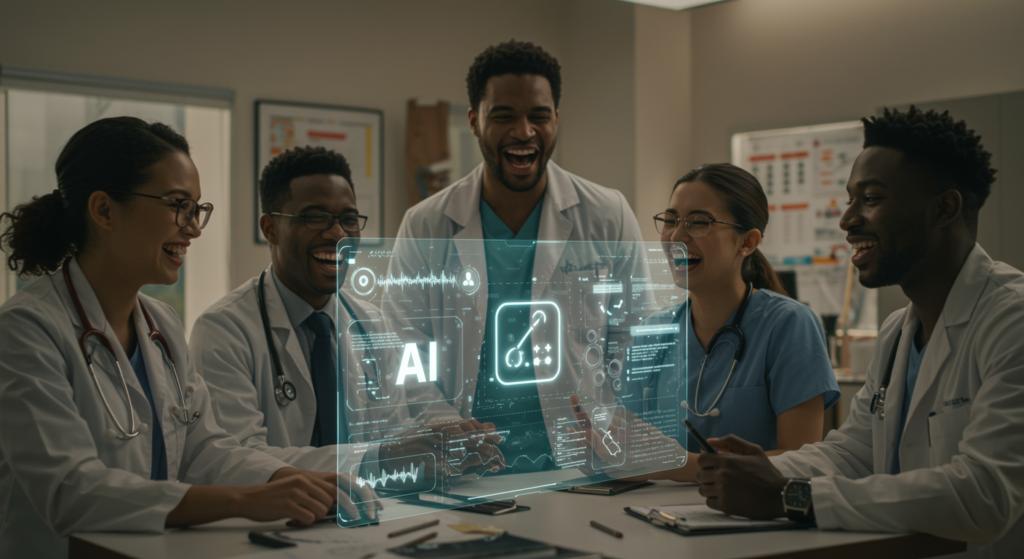
AI Bias in Medicine: How Hidden Prejudice Impacts Patient Care
Understanding AI Bias in Healthcare: A Growing Concern
AI bias in healthcare is becoming a critical issue as artificial intelligence increasingly shapes medical decisions, promising faster diagnoses and personalized treatments. Yet, these systems often carry hidden prejudices that can widen gaps in care for vulnerable groups. For instance, imagine a patient from a marginalized community receiving suboptimal recommendations because an algorithm was trained on data that doesn’t reflect their reality—this is the troubling reality we’re facing today.
Evidence shows that AI bias in healthcare stems from flawed data and design choices, leading to disparities that affect racial and ethnic minorities the most. Healthcare providers are turning to these tools for efficiency, but without addressing these biases, we’re risking harm rather than progress.
How Bias Infiltrates Healthcare Algorithms
Bias in healthcare AI typically sneaks in through data issues, flawed algorithms, and human influences, each creating vulnerabilities that can perpetuate inequities.
Data-Driven Biases in Healthcare AI
At the core of AI bias in healthcare lies the training data, which often excludes diverse populations like Black and Latinx patients. This underrepresentation means algorithms excel for majority groups but falter elsewhere, as seen in cardiovascular risk models that misjudge risks for African American patients due to imbalanced datasets.
Have you ever wondered why certain health tools don’t seem to work for everyone? It’s because these data gaps lead to inaccurate predictions, underscoring the need for more inclusive datasets to combat AI bias in healthcare effectively.
Algorithmic Biases and Their Impact
Even with better data, the algorithms themselves can introduce bias by prioritizing historical patterns that ignore past injustices. For example, if a system recommends treatments based on uneven access to care, it ends up reinforcing those disparities.
This type of algorithmic bias in healthcare highlights how technical decisions can embed inequality, making it essential to rethink how we build these models for fairer outcomes.
Human Biases in Medical AI Development
The developers behind these systems bring their own perspectives, which can unintentionally skew algorithms toward certain groups. In radiotherapy tools, for instance, value judgments about treatment priorities might overlook diverse patient needs, amplifying AI bias in healthcare.
Think about it: If the team creating an AI doesn’t include voices from affected communities, how can it truly serve everyone? This human element often gets overlooked, but addressing it could lead to more balanced healthcare solutions.
Real-World Consequences of AI Bias in Healthcare
The effects of AI bias in healthcare go beyond theory, with real cases showing how it leads to poorer outcomes for marginalized patients.
Misdiagnosis and Delayed Care from Biased AI
Algorithms trained on limited data often miss diagnoses in underrepresented groups, like chest X-ray tools that struggle with female patients. Skin cancer detection algorithms also perform poorly on darker skin tones, delaying vital care and exemplifying AI bias in healthcare at its most harmful.
What if a simple scan missed a critical condition because of these flaws? Such errors not only endanger lives but also erode trust in medical technology.
Resource Allocation Inequities in Healthcare AI
Biased systems can make it harder for minorities to access resources, requiring them to be sicker than others to get the same help. This uneven distribution perpetuates AI bias in healthcare, leading to later interventions and reduced care quality.
As hospitals rely more on these tools, it’s crucial to ask: How can we ensure fair access for all? Actionable steps, like regular bias checks, could make a big difference here.
Amplification of Existing Disparities Through AI
AI systems can worsen healthcare inequities by learning from biased historical data, creating a cycle that deepens divides. Without intervention, this loop turns technology into a barrier rather than a bridge.
To break this pattern, experts recommend ongoing evaluations—a practical tip for healthcare teams to monitor and adjust their tools against AI bias in healthcare.
Strategies for Mitigating AI Bias in Healthcare
Tackling AI bias in healthcare involves a full-spectrum approach, from data collection to deployment, to ensure algorithms serve everyone equitably.
Building Diverse and Representative Data Sets
Start with inclusive data that covers various demographics, correcting for historical biases through statistical methods. This foundational step is key to reducing AI bias in healthcare and improving algorithm accuracy across groups.
For teams developing AI, a tip is to audit datasets regularly—what seems balanced might still harbor subtle inequities.
Participatory Approaches to Combat Healthcare AI Bias
Involving community representatives in AI development helps spot and address blind spots early. As Professor Fay Cobb Payton notes, incorporating cultural contexts can transform how algorithms are built, directly fighting AI bias in healthcare.
Imagine a development process where patients contribute their experiences—it’s not just idealistic; it’s a proven strategy for more relatable tech.
Rigorous Testing and Validation Against Bias
Test algorithms across demographics before launch and monitor them post-deployment to catch emerging issues. This ongoing vigilance is vital for minimizing AI bias in healthcare.
A simple action: Set up performance metrics that track equity, ensuring your tools evolve with real-world feedback.
Transparent Reporting in Medical AI
Clear documentation of data sources and methods builds accountability, making it easier for providers to understand and trust AI systems. Transparency directly counters AI bias in healthcare by shining a light on potential problems.
Providers can demand this openness, fostering a culture where bias isn’t hidden but actively managed.
Institutional and Regulatory Frameworks for AI Bias in Healthcare
Strong policies and regulations are needed to enforce equity in AI, going beyond individual efforts.
Organizational Oversight to Address Healthcare AI Bias
Hospitals should form diverse committees to review algorithms for bias and conduct regular audits. This internal accountability helps prevent AI bias in healthcare from taking root.
If you’re in healthcare leadership, consider starting with an equity-focused policy—it’s a straightforward way to protect patients.
Regulatory Approaches for Equitable AI in Medicine
Agencies like AHRQ are developing guidelines, pushing for bias assessments and performance reporting. These measures could standardize how we tackle AI bias in healthcare across the industry.
What would happen if regulations required fairness by default? It could revolutionize how AI is adopted, making equity a non-negotiable standard.
The Future of Equitable Healthcare AI
Emerging tools and practices offer hope for overcoming AI bias in healthcare, paving the way for truly inclusive technology.
Synthetic Data Generation to Fight Bias
Creating synthetic data helps balance training sets without privacy risks, addressing gaps in real-world data. This innovation could be a game-changer in reducing AI bias in healthcare.
Researchers are already experimenting with this—why not explore it in your projects for more robust results?
Fairness-Aware Algorithms in Healthcare
New algorithms designed with fairness in mind optimize for all groups, not just accuracy. Incorporating these could significantly diminish AI bias in healthcare.
It’s an exciting field; adopting fairness constraints might just be the next big step for developers.
Collaborative Networks Against AI Bias
Sharing resources through open networks accelerates progress, helping establish best practices to combat AI bias in healthcare. Collaboration could lead to industry-wide standards that benefit everyone.
Joining such networks isn’t just helpful—it’s a proactive move toward a fairer future.
Conclusion: A Call for Vigilance and Action
AI bias in healthcare threatens to deepen disparities if left unchecked, but with deliberate efforts, we can turn the tide. From diverse data to strong oversight, the strategies outlined here offer a roadmap for ethical AI in medicine.
What are your thoughts on this? I’d love to hear how you’re addressing these issues in your work—share in the comments, explore more on our site, or spread the word to spark change. Together, we can ensure AI enhances care for all.
References
1. “AI Algorithms Used in Healthcare Can Perpetuate Bias,” Rutgers University, https://www.newark.rutgers.edu/news/ai-algorithms-used-healthcare-can-perpetuate-bias.
2. “Eliminating Racial Bias in Health Care AI,” Yale School of Medicine, https://medicine.yale.edu/news-article/eliminating-racial-bias-in-health-care-ai-expert-panel-offers-guidelines/.
3. “Algorithmic Bias in Healthcare,” PMC, https://pmc.ncbi.nlm.nih.gov/articles/PMC11542778/.
4. “Mitigating Bias in AI for Healthcare,” PMC, https://pmc.ncbi.nlm.nih.gov/articles/PMC8515002/.
5. “Overcoming AI Bias in Healthcare,” Accuray, https://www.accuray.com/blog/overcoming-ai-bias-understanding-identifying-and-mitigating-algorithmic-bias-in-healthcare/.
6. General AI Writing Insights, Black Hat World, https://www.blackhatworld.com/seo/how-to-use-ai-to-write-blog-posts-without-penalization.1569681/.
7. “Shedding Light on Healthcare Algorithmic Bias,” HHS, https://minorityhealth.hhs.gov/news/shedding-light-healthcare-algorithmic-and-artificial-intelligence-bias.
8. Video on AI Ethics, YouTube, https://www.youtube.com/watch?v=KjFyhV1Lu3I.
AI bias in healthcare, algorithmic bias, healthcare inequities, medical AI, racial bias in medicine, inclusive healthcare algorithms, AI in medicine, equitable AI, healthcare disparities, bias mitigation strategies