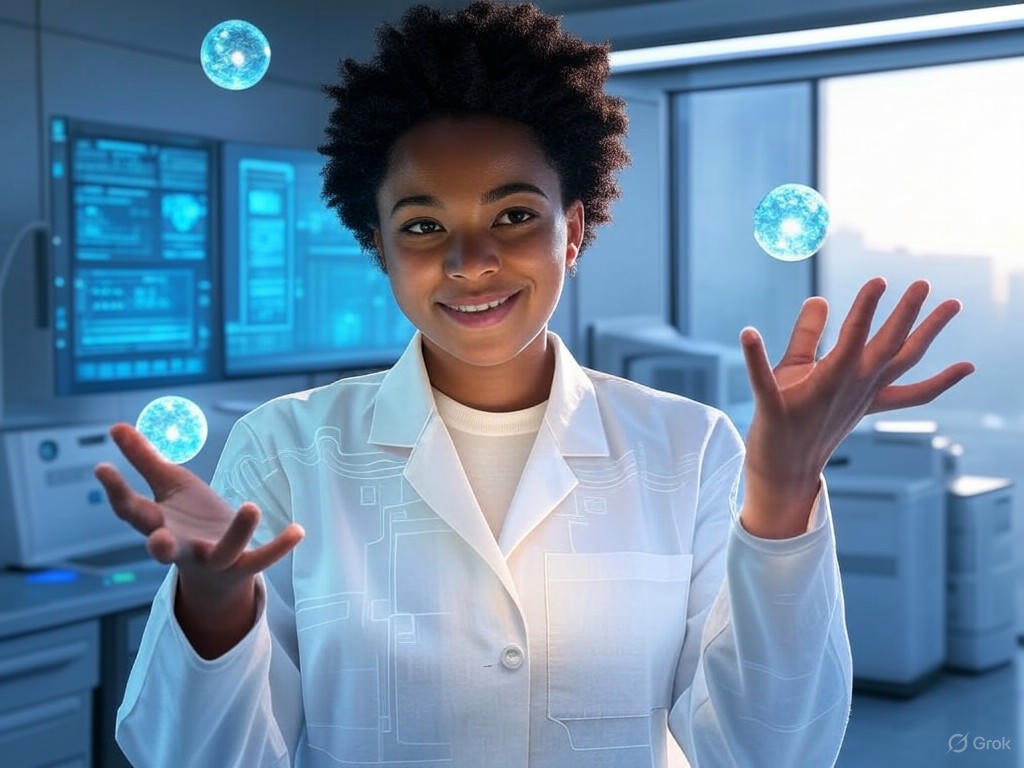
“`
AI Dilemmas: The Persistent Challenges in Artificial Intelligence
The Evolving Landscape of AI Challenges in 2025
AI challenges are reshaping how businesses approach technology, pushing organizations to confront hurdles that could make or break their digital strategies. As we step into 2025, artificial intelligence promises a staggering global economic boost—think $19.9 trillion by 2030—but turning that potential into reality isn’t straightforward. Have you noticed how AI can ace complex tasks like solving math Olympiad problems yet falter on everyday reasoning tests?
This gap underscores the core AI challenges we’re facing today, from adapting to rapid tech advancements to handling ethical pitfalls. Major players like Google and Meta are racing ahead with innovations in language models and image generation, but these breakthroughs come with a tangle of issues that demand smart decisions from everyone involved.
Data Quality, Availability, and Bias: A Core AI Challenge
At the root of many AI challenges is the quality of data feeding these systems. If the data is flawed—think inaccurate or incomplete sets—AI models spit out unreliable results, much like building a house on shaky ground. Organizations often deal with siloed data, especially in regulated industries, which starves AI of the diverse inputs it needs to thrive.
Then there’s bias, a sneaky AI challenge that can turn historical inequalities into amplified problems, leading to decisions that feel unfair or discriminatory. It’s not just about ethics; this can hit a company’s reputation hard and slow down adoption. To tackle these, companies are adopting strict data governance, hunting for varied datasets, and running ongoing checks to keep things balanced and effective.
Imagine training an AI to hire candidates—without diverse data, it might overlook great talent based on outdated biases. That’s why addressing these AI challenges head-on is crucial for building trust and real-world value.
Strategies for Tackling Data-Related AI Challenges
Overcoming data-focused AI challenges starts with solid frameworks for data management. By focusing on diverse sources and regular audits, businesses can minimize risks and enhance AI performance. This isn’t just technical—it’s about creating systems that promote fairness and accuracy from the ground up.
Privacy and Security: Major Hurdles in the AI Era
Privacy and security stand out as pressing AI challenges, especially as AI digs deeper into sensitive areas like customer data and financial records. With strict regulations in play, one slip-up can lead to massive fines, as seen with Amazon’s $900 million penalty from the EU or Meta’s over $1 billion hit.
These incidents highlight how AI challenges extend beyond data to include vulnerabilities like adversarial attacks, where bad actors tweak algorithms for misleading outcomes. It’s a wake-up call for stronger defenses, blending traditional cybersecurity with AI-specific strategies to protect what’s at stake.
What if a breach exposed personal details through an AI system? That’s why robust measures, like encryption and access controls, are essential to navigate these AI challenges securely.
Building Strong Frameworks Against AI Challenges
To combat privacy-related AI challenges, organizations should prioritize compliance with standards like GDPR and CCPA. This means layering on encryption, monitoring access, and conducting thorough audits to safeguard data. Think of it as fortifying a digital fortress—one that’s adaptable and resilient in the face of evolving threats.
Optimizing AI Implementation: Another Key Challenge
As 2025 unfolds, optimizing AI isn’t just a nice-to-have; it’s a central AI challenge for businesses aiming to maximize returns. With more than 70% of organizations already profiting from generative AI, the focus is on fine-tuning models to match specific needs, from cost efficiency to quality outputs.
This involves upgrading the entire tech stack, selecting the best models for tasks, and streamlining infrastructure for faster processing. Take LG AI Research, for instance—they slashed inference times by over 50% and cut costs by 72% using a mix of hardware, showing how mastering these AI challenges can lead to big wins.
Is your team ready to dive into optimization? By investing in the right tools and metrics, you can turn potential pitfalls into powerful advantages.
A Practical Look at Overcoming AI Implementation Challenges
Real-world examples like LG’s success story offer actionable insights into AI challenges. Start by evaluating your current setup and experimenting with hybrid hardware solutions. This approach not only boosts performance but also ensures long-term adaptability.
Breaking Down Organizational Barriers: An Overlooked AI Challenge
Internal silos often amplify AI challenges, stifling collaboration and innovation within companies. Generative AI is changing that by putting powerful tools in everyone’s hands, from marketers to engineers, and freeing up time for more creative work.
Platforms like Google Workspace are leading the charge, integrating AI into daily apps to break these barriers. It’s about fostering a culture where AI enhances teamwork, not complicates it. How might this shift impact your daily operations?
By promoting cross-departmental sharing, organizations can turn these AI challenges into opportunities for growth and efficiency.
Ethical Dilemmas and Their Socio-Economic Effects
Ethics forms a major part of the broader AI challenges, touching on issues like job displacement, transparency, and bias in algorithms. As AI scales, we must weigh its benefits against risks, such as cybersecurity threats that could undermine societal trust.
This calls for a balanced strategy that prioritizes responsible AI development. For example, ensuring models are transparent can prevent unintended harm and build public confidence. What’s your take on balancing innovation with these ethical AI challenges?
Actionable steps include forming interdisciplinary teams to review AI impacts and adopting guidelines that emphasize accountability.
The Road to Responsible AI Amid Ongoing Challenges
Navigating ethical AI challenges means integrating privacy protections and bias checks into every stage of development. It’s about creating AI that not only performs well but also aligns with human values, turning potential risks into strengths.
Ensuring Content Quality in the Age of AI
One of the trickier AI challenges is maintaining content quality when AI generates it at scale. Without proper oversight, you risk triggering search engine penalties for “scaled content abuse,” as Google highlighted in their 2024 update.
It’s tempting to churn out volumes of content, but quality always wins over quantity. Always keep humans in the loop for reviews to avoid diluted messaging and protect your SEO efforts. How do you ensure your AI-assisted content stands out?
Start with gradual scaling, rigorous checks, and a focus on value—tips that can help you sidestep these AI challenges effectively.
Key Trends Shaping the Future of AI Challenges
Despite the hurdles, five trends from industry experts are set to influence how we handle AI challenges moving forward. These include the demand for custom chips to boost AI reasoning and how cloud providers are turning AI workloads into revenue streams.
From large language models in enterprises to data firms evaluating AI effectiveness, these developments aim to address core AI challenges like performance and security. Software companies are even eyeing “agentic AI” for smarter, autonomous systems.
This evolution reminds us that staying ahead of AI challenges requires adaptability and forward-thinking strategies.
Moving Forward: Adapting to AI Challenges
In a field as dynamic as AI, continuous learning is key to overcoming persistent challenges. Businesses that stay updated through communities and strategy reviews will maintain their edge. It’s about evolving with the technology, not just reacting to it.
Are you integrating regular training into your AI plans? This proactive stance can transform potential obstacles into opportunities for innovation.
Wrapping Up: Mastering AI Challenges
AI challenges in 2025 are complex but not insurmountable, covering everything from data issues to ethical considerations. By implementing strong governance, security, and optimization practices, organizations can harness AI’s potential while minimizing risks.
Remember, success comes from a holistic approach that balances tech with responsibility. What steps will you take to tackle these AI challenges in your own work?
If this resonates, I’d love to hear your thoughts in the comments below—share your experiences or questions, and let’s keep the conversation going. For more insights, check out our related posts on AI ethics and optimization strategies.
References
- Morgan Stanley Technology, Media & Telecom Conference Insights. (2024). AI Trends and Frontier Models.
- MIT Sloan Review. (2024). Five Trends in AI and Data Science for 2025.
- Simplilearn. (2024). Challenges of Artificial Intelligence.
- Stanford HAI. (2025). 2025 AI Index Report.
- Converge Technology Solutions. (2025). Top 5 AI Adoption Challenges for 2025.
- Ovrdrv. (2024). SEO Techniques for AI-Generated Content.
- Google Cloud. (2024). 2025 and the Next Chapters of AI.
- WinSavvy. (2024). AI for SEO-Optimized Content Creation.
“`