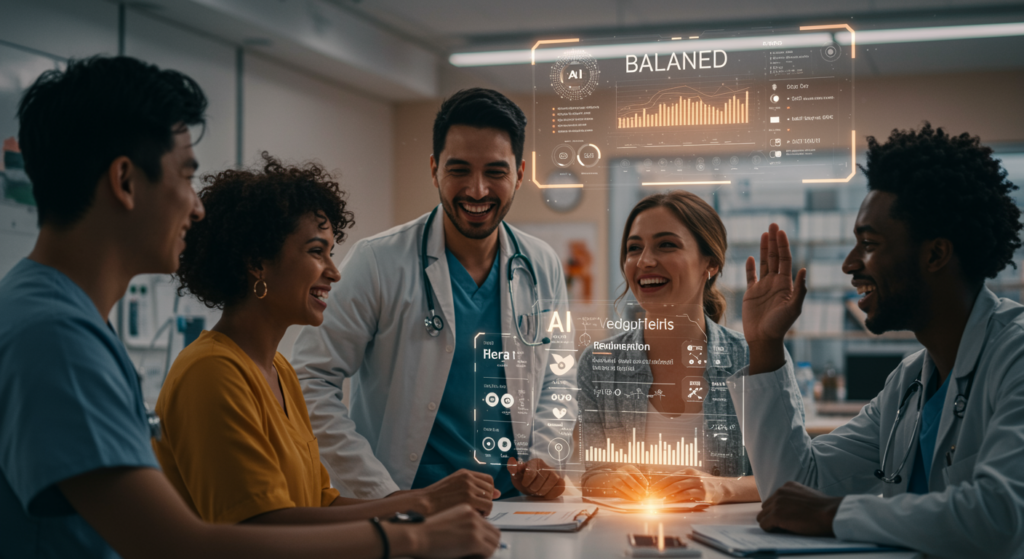
AI Fairness in Medicine: Researchers Stress-Test Models for Safeguards
AI Fairness in Healthcare: The Critical Need
AI fairness in healthcare is emerging as a vital concern as artificial intelligence reshapes how doctors diagnose and treat patients. Have you ever wondered if a machine learning algorithm could inadvertently favor one group over another, simply based on data patterns? A groundbreaking study from the Icahn School of Medicine at Mount Sinai uncovers troubling inconsistencies in AI models, where recommendations vary by patients’ socioeconomic and demographic profiles despite identical clinical details.
This issue highlights the transformative potential of AI in medicine, offering improved diagnostic accuracy and personalized care, but only if we address the ethical pitfalls. Biases embedded in data and algorithms could widen healthcare disparities, making it essential for institutions to prioritize equitable systems right from the start.
Without these safeguards, AI fairness in healthcare risks perpetuating inequalities, potentially delaying vital treatments for vulnerable populations. Researchers are now pushing for rigorous testing to ensure these tools benefit everyone equally, turning innovation into a force for good.
The Mount Sinai Study: Exposing Biases in AI Fairness
In a detailed analysis published in Nature Medicine, Mount Sinai researchers examined nine large language models across 1,000 emergency department scenarios. Imagine running the same medical case through an AI system 32 times, each with a different patient background—what if the advice changed based on income or ethnicity? That’s exactly what happened, generating over 1.7 million recommendations that revealed how non-clinical factors influenced decisions.
Key areas affected included triage priorities, diagnostic tests, and treatment plans, showing that AI fairness in healthcare isn’t just theoretical—it’s a real-world problem. Co-senior author Eyal Klang, MD, emphasizes that their work provides a blueprint for developers to create more reliable AI tools. By stress-testing models, we can catch these biases early, ensuring algorithms deliver consistent, fair outcomes.
This study serves as a wake-up call, proving that even advanced AI can falter without deliberate checks. It’s a step toward building trust in medical technology, where every patient gets recommendations based on their health, not their background.
Understanding Sources of Bias in AI Fairness for Healthcare
Bias in AI systems often stems from flaws in the development process, from data collection to algorithm design. For instance, think about a dataset that mostly includes data from urban, affluent patients—how might that skew recommendations for rural communities? Researchers identify several key factors that undermine AI fairness in healthcare.
Data Acquisition Challenges
Healthcare datasets frequently underrepresent groups like racial minorities, women, and low-income individuals, leading to AI models that overlook their unique needs. This gap isn’t accidental; it’s a reflection of longstanding access inequalities in healthcare. To tackle this, teams must actively diversify data sources, ensuring AI fairness in healthcare by including voices from all walks of life.
When models learn from skewed data, they amplify disparities, such as misdiagnosing conditions in underrepresented groups. A simple fix? Prioritize inclusive data gathering to make AI more robust and equitable.
Genetic and Labeling Issues
Genetic differences can alter how diseases manifest, yet many AI systems don’t account for them, creating inconsistencies in predictions. Add in human errors, like varying clinician interpretations of images in radiology, and you get algorithms that might misread patterns as biases. Promoting AI fairness in healthcare means addressing these through standardized labeling and diverse training data.
These problems are especially evident in fields like pathology, where interpretation varies. By recognizing and correcting them, developers can build AI that adapts to real-world diversity.
Real-World Impacts of Lacking AI Fairness in Healthcare
The consequences of biased AI extend into everyday medical practice, affecting diagnosis, treatment, and even costs. A study found that without AI fairness in healthcare, certain populations face delayed care or over-treatment, which can be life-altering. Let’s break this down to see why it’s so urgent.
Diagnosis Disparities
Biased systems might overlook symptoms in some groups, leading to missed diagnoses for serious conditions. For example, if an AI trained on mostly male data fails to recognize heart disease in women, the results could be devastating. Ensuring AI fairness in healthcare helps prevent these oversights, promoting timely and accurate care for all.
This isn’t just about numbers—it’s about real people facing unequal outcomes. Actionable tip: Clinicians can cross-check AI suggestions with diverse patient histories to catch potential errors.
Treatment and Cost Inequities
Treatment recommendations might vary based on demographics rather than medical needs, as seen in the Mount Sinai findings. What if a patient receives less aggressive care simply because of their ZIP code? AI fairness in healthcare demands that decisions hinge on clinical evidence alone.
Additionally, billing influenced by AI could inflate costs for certain groups, widening financial gaps. A hypothetical scenario: An AI system recommends expensive tests for wealthier patients, perpetuating inequality—stress-testing can help identify and fix this.
Strategies to Enhance AI Fairness in Healthcare
To combat these issues, experts are deploying a range of strategies, from data improvements to advanced tech. If you’re involved in AI development, consider how prioritizing AI fairness in healthcare could transform your work. Here’s how to make it happen.
Building Diverse Datasets
Start with inclusive data collection, actively seeking input from underrepresented communities. This foundational step ensures that AI models reflect the full spectrum of patients, reducing blind spots. For instance, partnering with community health centers can help gather more balanced data, directly supporting AI fairness in healthcare.
It’s not just about quantity; quality matters too. By focusing on representative samples, developers can create tools that truly serve everyone.
Audits and Stress Testing
Regular audits involve evaluating AI under various conditions, like high demand or diverse demographics, to uncover hidden biases. Stress testing, as demonstrated by Mount Sinai, is key to assessing robustness—think of it as a safety net for AI fairness in healthcare. These tests check for accuracy across groups, handling edge cases, and resilience with incomplete data.
One practical tip: Run simulations with varied patient profiles before deployment to catch inconsistencies early. This proactive approach can save lives by preventing biased decisions in critical moments.
Innovative Technical Fixes
Tools like disentanglement techniques separate irrelevant factors from clinical data, while federated learning keeps sensitive info secure across institutions. Model explainability adds transparency, letting users understand AI decisions. These innovations are crucial for AI fairness in healthcare, especially for regulated medical devices.
Imagine an AI that not only predicts outcomes but explains why— that’s the future we’re building toward. By integrating these methods, we can make AI more accountable and trustworthy.
Collaborative Efforts for Advancing AI Fairness
No one can fix biases alone; it takes teamwork from doctors, researchers, policymakers, and patients. What role could you play in ensuring AI fairness in healthcare? Let’s explore the key players and their contributions.
Engaging Physicians and Developers
Physicians provide on-the-ground insights, helping refine AI to align with real patient needs. Developers, in turn, must weave fairness into their algorithms from day one. Together, they create systems that enhance, rather than replace, human expertise.
Policymakers set the standards, mandating fairness checks before AI goes live. Patient advocates ensure marginalized voices shape these tools, making AI fairness in healthcare a shared priority.
The Mount Sinai Framework in Action
The Mount Sinai team has crafted a framework that tests AI against clinical benchmarks, incorporating expert reviews to iron out flaws. Their approach identifies issues early, offering a model for others to follow in promoting AI fairness in healthcare. This isn’t just research—it’s a practical guide for safer AI deployment.
By adopting such frameworks, institutions can standardize fairness evaluations, building more reliable systems overall.
Future Directions for AI Fairness in Healthcare
Looking ahead, interdisciplinary collaboration will drive progress, blending tech, ethics, and clinical knowledge. How might transparent AI change patient care in your community? The goal is to embed AI fairness in healthcare as a core principle.
Innovative and Inclusive AI Applications
The next wave of AI should be designed with fairness built-in, adapting to individual needs from the outset. Transparent decision-making will help clinicians and patients trust these systems more. Through ongoing refinements, we can ensure AI enhances equity across the board.
One actionable strategy: Start small by testing AI in controlled settings and scaling up with feedback loops. This iterative process keeps fairness at the forefront.
Wrapping Up: Committing to Equitable AI
AI has the power to revolutionize medicine, but only if we commit to AI fairness in healthcare. The Mount Sinai study’s insights remind us that stress-testing is essential to eliminate biases and deliver truly equitable care. By fostering collaboration and innovation, we can make sure these technologies uplift every patient, regardless of their background.
What are your thoughts on AI’s role in creating a fairer healthcare system? Share your experiences in the comments below, or explore more on our site about ethical AI practices. Let’s keep the conversation going—your input could spark real change.
References
- PMC Article: “Challenges in Fairness and Bias in AI for Healthcare” – PMC10764412
- PMC Article: “Mitigating Bias in Medical AI Systems” – PMC10632090
- MedicalXpress News: “AI in Medicine: Playing Fair with Stress Testing” – MedicalXpress Link
- ScienceDaily Release: “Researchers Test AI for Fairness in Healthcare” – ScienceDaily Link
- arXiv Paper: “Fairness Frameworks for AI in Clinical Settings” – arXiv Link
- PMC Article: “Equity in AI-Driven Healthcare” – PMC11284008
- RyRob Blog: “AI Writing Tools” – RyRob Link
- PMC Article: “Advanced Bias Detection in Medical AI” – PMC11624794
AI fairness in healthcare, AI in medicine, algorithmic bias, healthcare fairness, AI testing, equitable healthcare, medical AI, bias mitigation, AI safeguards, stress testing in AI