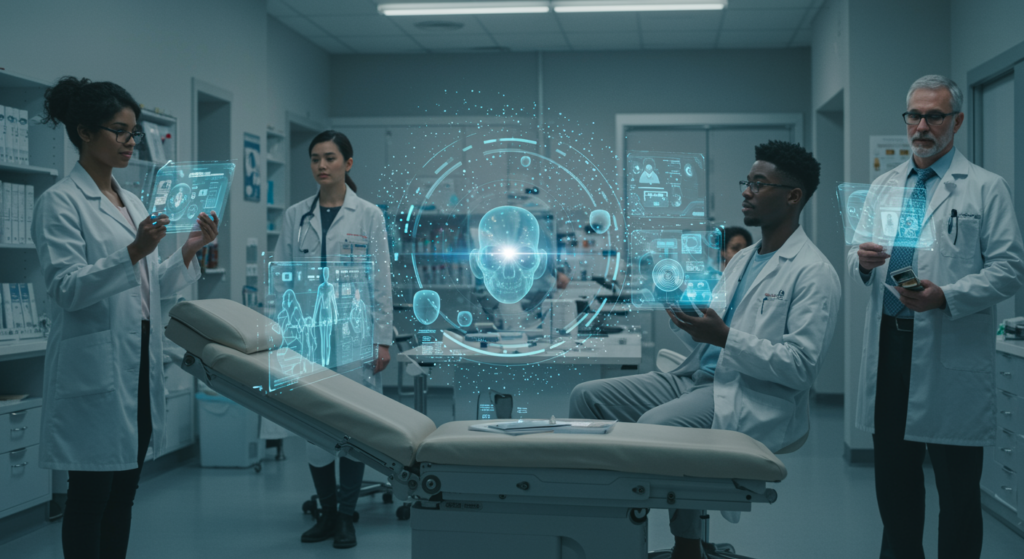
AI Fairness in Medicine: Is It Truly Equitable?
The Promise and Peril of AI in Modern Medicine
Imagine a world where AI tools catch diseases early, personalize treatments, and streamline hospital operations—it sounds revolutionary, doesn’t it? But as AI fairness in healthcare becomes a hot topic, we must ask: are these advancements really helping everyone equally? Artificial intelligence is reshaping healthcare by enhancing diagnostics, treatment planning, and efficiency, yet it carries risks of amplifying inequalities.
This dual edge of innovation raises critical questions about equity. While AI promises better outcomes, without careful oversight, it could deepen divides based on race, income, or location. Let’s dive into how we can navigate this landscape to ensure AI serves all patients fairly.
Understanding Fairness in Healthcare AI
At the heart of AI fairness in healthcare lies the goal of equitable benefits for every patient, regardless of background. The National Academy of Medicine describes it as AI applications backed by evidence of unbiased development processes. This isn’t just about avoiding harm; it’s about proving that technology delivers justice in medical settings.
Key aspects include ensuring equal diagnostic accuracy across demographics, providing fair access to AI-driven services, and using balanced data sets. Have you ever wondered if your doctor’s AI tool treats your data the same as someone from a different community? Health equity demands that AI bridges gaps, not widens them, by offering high-quality care to all, irrespective of socioeconomic status or ethnicity.
How Algorithmic Bias Fuels Healthcare Inequities
AI fairness in healthcare faces a major hurdle: algorithmic bias, which can entrench existing disparities. Research shows that AI systems often favor dominant groups, leading to misdiagnoses or unequal care for underrepresented communities. This isn’t just a tech glitch—it’s a real issue affecting lives every day.
Sources of Bias in AI Systems
One common source is biased training data, where algorithms learn from datasets dominated by certain groups, like middle-aged white males, and falter with others. For instance, if historical medical records reflect past inequalities, the AI inherits those flaws. But what if we redesigned these systems to spot and correct such issues early?
Other factors include flawed algorithm designs that unintentionally prioritize specific outcomes and a lack of consideration for environmental influences, such as pollution in low-income areas. These elements can result in severe consequences, like delayed treatments for vulnerable populations, underscoring why tackling AI fairness in healthcare is so urgent.
Real-World Effects on Patients
Take AI tools for detecting diabetic retinopathy; they’re often rolled out in affluent clinics, leaving high-risk, underserved communities without access. This scenario highlights how AI, meant to improve health, might instead widen gaps. It’s a wake-up call: without equity-focused strategies, AI fairness in healthcare remains out of reach for many.
Strategies for Achieving AI Fairness in Healthcare
To make AI fairness in healthcare a reality, we need targeted approaches that address bias at every stage. From diverse data collection to policy changes, these strategies can help build more equitable systems. Think of it as equipping AI to be a true ally in medicine, not just a powerful tool.
Building with Diverse and Representative Data
Start with the basics: training data must reflect a wide range of demographics to avoid skewed results. Developers should set diversity benchmarks and actively gather data from underrepresented groups. For example, reweighting techniques can balance datasets, ensuring AI performs reliably across all users— a simple yet effective step toward AI fairness in healthcare.
This method not only reduces errors but also builds trust. If you’re a clinician relying on AI, wouldn’t you want it trained on data that mirrors your patient base?
Ensuring Rigorous Validation for Equitable Outcomes
Before AI hits the clinic, thorough testing across diverse groups is essential. This means measuring not just overall accuracy but also how the system handles different demographics, like checking for disparities in false positives. By adopting equity-specific metrics, we can catch problems early and promote genuine AI fairness in healthcare.
Actionable tip: Always evaluate AI for variations in performance, such as how it impacts treatment access. This proactive validation can prevent real-world harm and foster more inclusive tech.
Strengthening Regulatory Frameworks
Governments play a key role here, with bodies like the FDA setting standards for AI fairness in healthcare. Current regulations cover some clinical uses, but we need broader rules for things like resource allocation. Imagine policies that demand transparent reporting on demographic performance—it’s a game-changer for accountability.
Fostering Stakeholder Collaboration
Bringing together doctors, researchers, and patients creates a stronger defense against bias. This teamwork throughout the AI lifecycle— from design to monitoring—ensures diverse perspectives shape the technology. Collaboration isn’t just helpful; it’s crucial for advancing AI fairness in healthcare and making sure no one gets left behind.
Education and Transparency in AI Use
Even the best AI needs users who understand its limits. Educating healthcare providers about potential biases empowers them to question recommendations and protect patients. Transparency, like making AI decision processes clear, helps everyone—from doctors to patients—stay informed and engaged.
For instance, explaining how AI influences a diagnosis can build trust and allow for better oversight. In your next doctor’s visit, wouldn’t it be reassuring to know if AI is involved and how fair it truly is?
Navigating Ethical and Legal Challenges
Protecting Patient Consent and Autonomy
Ethical AI fairness in healthcare means clear communication about AI’s role in care. Patients deserve to know if algorithms might carry biases, ensuring informed choices and respect for autonomy. This isn’t just about compliance; it’s about rebuilding trust in a tech-driven world.
Safeguarding Data Privacy and Security
With AI relying on vast data, privacy becomes a fairness issue, especially for at-risk groups. Strong protections prevent breaches that could exacerbate inequalities, balancing innovation with ethical responsibility.
Establishing Accountability for AI Decisions
When AI contributes to errors, who’s accountable? Clear frameworks are needed to address this, particularly when disparities arise. It’s about creating systems where responsibility is shared, ensuring AI fairness in healthcare translates to real-world justice.
The Dual Mandate: Tackling and Preventing Disparities
AI in medicine should do more than avoid harm—it must actively fight inequalities. This means designing systems that address historical disparities while preventing new ones from emerging. It’s a bold shift, turning AI into a tool for positive change in healthcare equity.
For example, algorithms could prioritize underserved areas, making AI fairness in healthcare a driver of inclusion rather than division.
Future Directions: Innovating for Better Equity
Looking ahead, research is key to overcoming gaps in AI fairness in healthcare. We need standardized metrics for bias detection and methods like federated learning to train models without compromising data privacy. Emerging tools, such as explainable AI, promise greater transparency, but they’re only part of the solution.
Long-term studies on AI’s impact will guide us, ensuring these technologies evolve to support health equity. What innovations do you think could make the biggest difference here?
Conclusion: A Shared Responsibility
AI fairness in healthcare isn’t automatic—it demands ongoing effort from everyone involved. By focusing on equity in development and use, we can turn AI into a force for good, reducing disparities and improving lives. This is our chance to shape a more just medical future.
So, what are your thoughts on making AI more equitable? Share your experiences in the comments, explore our related posts on ethical tech, or spread the word to spark more conversations. Let’s work together to ensure AI benefits us all.
References
1. A study from PMC highlights steps for fair AI development: Eliminating Bias in Health Care AI.
2. JAMA Network discusses equitable AI frameworks: Health Equity and AI.
3. Yale Medicine offers guidelines on reducing racial bias: Guidelines for AI Fairness.
4. Another PMC article addresses regulatory needs: AI in Public Health.
5. CDC resources on health disparities: Health Equity Insights.
6. Foreseemed blog on AI bias: AI Bias in Healthcare.
7. Techtarget on advancing fairness: Transparency in AI.
AI fairness in healthcare, health equity, algorithmic bias, ethical AI in medicine, healthcare disparities, medical AI implementation, equitable artificial intelligence, AI bias in healthcare, strategies for AI fairness, reducing medical inequalities