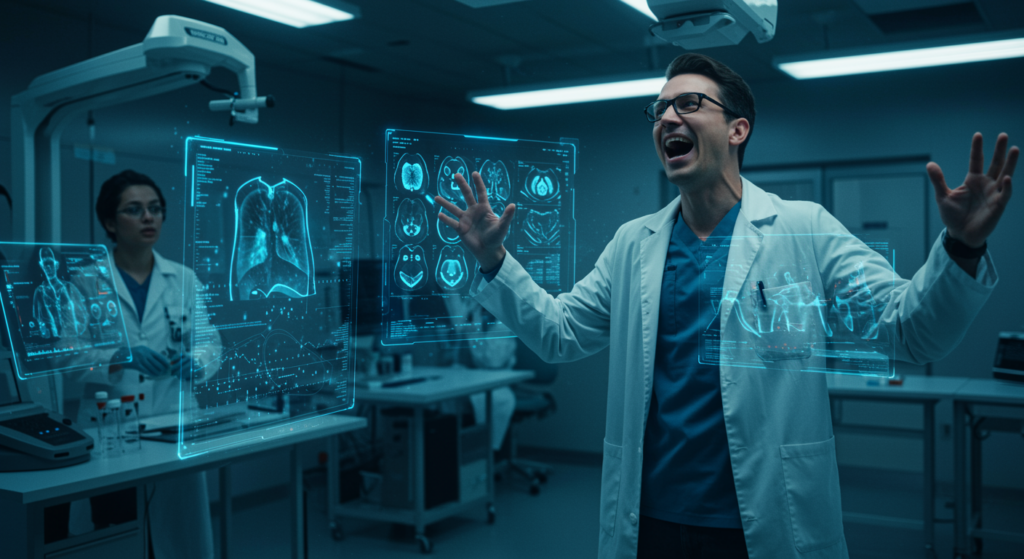
AI for Radiologist Shortage: Pros, Cons, and Realities
The Radiologist Shortage Crisis: Can AI in Radiology Step In?
The global healthcare landscape is grappling with a critical shortage of radiologists, where rising imaging demands often outpace available experts, leading to delays in diagnoses that can affect patient outcomes. AI in radiology emerges as a beacon of hope, promising to alleviate this burden by automating routine tasks and enhancing efficiency. According to a recent literature review in Health and Technology from April 2025, AI could potentially cut radiologist workloads by about 53%, freeing up time for complex cases that truly need human insight.
Imagine a busy hospital where scans pile up, but AI steps in to handle the straightforward ones—think low-risk images flagged automatically. This isn’t just theory; it’s already showing real results, helping healthcare systems adapt without compromising care quality.
Have you ever wondered how technology could bridge gaps in medical staffing? AI in radiology does exactly that, by prioritizing urgent findings and ensuring no critical issue slips through the cracks.
How AI in Radiology Is Reshaping Everyday Practice
AI in radiology isn’t about flashy gadgets; it’s about making daily workflows smarter and more sustainable. These tools are designed to work alongside radiologists, tackling specific challenges like image overload and decision fatigue.
Streamlining Workflow with Smart Triage
One standout benefit is how AI handles case prioritization, quickly sorting through scans to flag emergencies while setting aside routine ones. For instance, software that’s gained approval in Europe can auto-generate reports for normal chest X-rays, letting radiologists focus on what matters most.
This approach cuts down on burnout by reducing the mental juggling act of mixed-priority tasks. In a typical day, could AI in radiology help you, as a healthcare professional, get home on time while ensuring patients get timely care?
Boosting Image Analysis Precision
AI excels at spotting patterns and making precise measurements in images, offering consistent results that support better disease tracking. Think of it as a reliable assistant that measures tumor sizes accurately every time, aiding in monitoring conditions like cancer progression.
This capability doesn’t replace expertise; it enhances it, providing data that human eyes might overlook in a rush. For patients in remote areas, AI in radiology could mean faster preliminary insights, bridging the gap until a specialist reviews the case.
Key Advantages of Integrating AI in Radiology
Bringing AI into radiology goes beyond fixing shortages—it’s about elevating overall care. When done right, it delivers benefits that touch everyone from providers to patients.
Enhancing Accuracy Through Consistent Insights
AI in radiology acts like a vigilant second pair of eyes, catching subtle anomalies that fatigue might hide from human view. Studies show it can reduce errors by maintaining steady analysis, ensuring diagnoses are as reliable as possible across different settings.
This consistency minimizes variations between radiologists, fostering trust in results. Ever considered how a small tech boost could make such a big difference in spotting early-stage issues?
Speeding Up Diagnoses for Better Efficiency
The rapid processing power of AI means quicker turnarounds on imaging, which is a game-changer in emergencies. By automating basics, radiologists can dedicate more energy to intricate cases requiring nuanced judgment.
This efficiency ripple effect improves patient experiences and outcomes. In underserved regions, AI in radiology could expand access, offering preliminary reads that save lives in areas without on-site experts.
Expanding Care to Hard-to-Reach Places
AI in radiology holds the key to democratizing expertise, especially in rural or low-resource areas. It can provide initial interpretations, ensuring patients get guidance even when specialists are scarce.
Key Benefits | Impact on Shortage |
---|---|
Workload cut by around 53% | Lets current radiologists manage more without overload |
Auto-handling of simple cases | Redirects focus to high-stakes diagnoses |
Better remote access | Brings services to isolated communities |
Faster processing times | Reduces delays and backlogs |
These advantages aren’t pie-in-the-sky; they’re practical steps toward a more balanced healthcare system.
Challenges in Bringing AI to Radiology Workflows
While AI in radiology offers exciting potential, it’s not without hurdles that could trip up implementation. From technical glitches to ethical dilemmas, these issues demand careful navigation.
The Limits of AI’s Contextual Understanding
AI shines at data crunching but often falls short on the big picture, like factoring in a patient’s full medical history. This gap highlights why human judgment remains irreplaceable in comprehensive care.
For example, an AI might misinterpret an image without key background details. How can we ensure AI in radiology complements rather than complicates expert decisions?
Building Trust Amid Performance Variations
Research from Harvard Medical School in March 2024 showed that AI’s helpfulness depends on the radiologist using it, sometimes even hindering accuracy. This variability underscores the need for tailored approaches.
It’s a reminder that one-size-fits-all doesn’t work here. What if AI in radiology tools were customized to individual practices for optimal results?
Tackling Bias and Data Quality Issues
AI’s effectiveness hinges on diverse, unbiased training data; otherwise, it might underperform with certain patient groups. Ongoing monitoring is essential to keep these systems fair and reliable.
Implementation costs add another layer, making it tough for smaller facilities to adopt. Despite these challenges, addressing them head-on could make AI in radiology more inclusive and effective.
Ethical and Regulatory Hurdles for AI in Radiology
AI’s rise in radiology brings ethical questions to the forefront, from liability concerns to protecting patient data. These aren’t just roadblocks; they’re opportunities to build safer systems.
Navigating Liability in AI-Assisted Decisions
When AI influences diagnoses, who’s responsible if things go wrong? Radiologists worry about accountability, pushing for clearer guidelines.
This is where regulations play a key role, defining how AI in radiology fits into professional responsibilities.
Protecting Privacy in a Data-Driven World
AI needs vast datasets, raising red flags about data security and consent. Ensuring robust protections is vital for patient trust.
Strong measures can turn this into a strength, making AI in radiology a secure ally in healthcare.
Adapting to Changing Regulations
Global rules for AI are evolving, creating a complex landscape for developers and users. Staying ahead means balancing innovation with compliance.
For healthcare teams, this could mean proactive planning to integrate AI in radiology smoothly. What steps can organizations take to stay compliant while innovating?
The Road Ahead for AI in Radiology and Shortages
Looking forward, AI in radiology will likely evolve as a supportive partner, not a replacement, helping to ease shortages sustainably. The focus is on collaboration that leverages tech’s speed with human wisdom.
AI as a Support Tool, Not a Substitute
Rather than overtaking jobs, AI in radiology enhances them by handling repetitive tasks, allowing radiologists to tackle more meaningful work. This partnership plays to strengths: AI’s consistency and humans’ intuition.
Picture a future where radiologists spend more time with patients, thanks to AI managing the routine.
Shifting Roles for Radiologists
As AI takes on basics, radiologists might shift toward consulting on complex cases or integrating data with clinical insights. This evolution could make the field more engaging and impactful.
It’s an exciting shift, but one that requires ongoing adaptation. How might this change inspire the next generation of professionals?
Leveraging AI for Education and Growth
AI can serve as a teaching aid, highlighting key cases and providing feedback to trainees. This could speed up learning and help build a stronger workforce.
By incorporating AI in radiology into training, we might address shortages more effectively in the long term.
Strategies for Successfully Implementing AI in Radiology
If your organization is considering AI to combat radiologist shortages, starting smart is crucial. Focus on practical steps that deliver quick wins and build confidence.
Kicking Off with Promising Use Cases
Target areas where AI shows clear value, like screening for normal images, to see immediate benefits. This builds a foundation for broader adoption.
Actionable tip: Begin with pilot programs to test AI in radiology tools in real settings. What high-impact area could you start with?
Prioritizing Training for Radiologists
Invest in education so radiologists know how to work with AI effectively. Tailored training can maximize its potential and minimize risks.
This human-AI collaboration is key—think of it as teaming up for better results.
Ensuring Ongoing Quality Checks
Regular audits of AI performance against human reviews help maintain standards and catch any issues early. This vigilance ensures AI in radiology remains a reliable asset.
Strong monitoring systems are non-negotiable for safe, effective implementation. How can your team set up these checks efficiently?
Wrapping Up: A Thoughtful Path Forward
The radiologist shortage is a pressing issue, but AI in radiology offers a balanced way to address it by automating the mundane and amplifying human skills. With careful implementation, it can boost efficiency, accuracy, and access without overlooking ethical and practical challenges.
Ultimately, the goal is better patient care, where technology and expertise work hand in hand. As you reflect on this, consider how AI might transform your corner of healthcare—share your thoughts in the comments below.
Ready to dive deeper? Explore more on our site or connect with experts to see how AI in radiology could work for you.
References
- Rayscape AI. (n.d.). Article on AI in radiology. Link.
- Radiology Business. (2023). AI and the radiologist shortage. Link.
- PMC. (2023). Article on AI in healthcare. Link.
- Harvard Medical School. (2024). Study on AI’s impact on radiologists. Link.
- Statim Healthcare. (n.d.). Pros and cons of AI in radiology. Link.
- Microbioz Health. (n.d.). Radiology and AI overview. Link.
- Self-Made Millennials. (n.d.). AI tools discussion. Link.
- Med School Insiders. (n.d.). Can AI replace radiologists? Link.
AI in radiology, radiologist shortage, artificial intelligence healthcare, medical imaging AI, radiology workflow, AI healthcare pros, diagnostic accuracy AI, ethical AI in medicine, AI implementation challenges, future of radiology AI