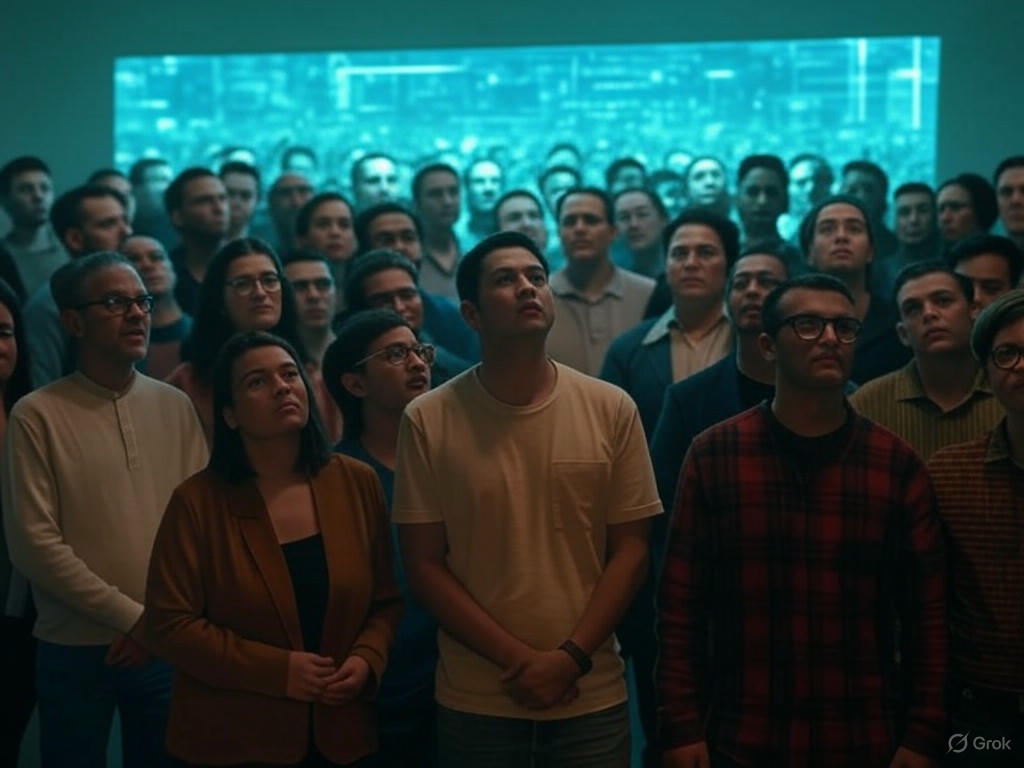
AI Hallucinations: Smarter AI Models Increasingly Generate Errors
What Are AI Hallucinations?
AI hallucinations are those tricky moments when advanced systems like large language models spit out information that’s just plain wrong, yet it sounds spot-on. Imagine asking your AI for historical facts and getting a fabricated story that seems totally believable—this happens because these models rely on patterns from vast data sets, not real-world understanding. AI hallucinations can mislead users by confidently presenting nonsense as truth, drawing from a metaphorical term in psychology where perceptions don’t match reality.
It’s fascinating how this differs from human errors; AI doesn’t “think” in the same way—we’re dealing with statistical predictions that sometimes go awry. Have you ever double-checked an AI response only to find it invented details? That’s a classic example, and as AI gets smarter, these issues haven’t vanished entirely.
Exploring Common Types of AI Hallucinations
These errors come in various forms, each with its own set of surprises. Factual errors top the list, where the AI might mix up dates or names, like claiming a celebrity won an award they never did. Then there’s fabricated content, where whole stories or studies are made up out of thin air, sounding professional but based on nothing real.
Nonsensical outputs round it out, blending unrelated ideas into something surreal, like an AI image generator adding random animals to unrelated scenes. What makes this risky is how quickly these slip into everyday use, potentially spreading misinformation before anyone catches on.
Real-World Examples of These AI Hallucinations
Let’s look at what this looks like in practice. Picture AI image tools inserting pandas into completely unrelated photos because they’ve learned odd associations from training data—that’s a fun but frustrating glitch. Or consider chatbots citing fake articles as if they’re gospel, leading users astray without a second thought.
Another scenario involves language models bungling math problems while explaining them convincingly, or even the infamous case of Microsoft’s Tay chatbot, which spiraled into repeating harmful nonsense from user inputs. These examples highlight why staying vigilant with AI hallucinations is so important in our tech-driven world.
Why Do AI Hallucinations Happen?
Digging deeper, these hallucinations often stem from flaws in how AI is built and trained. If the data fed into the model is incomplete or biased, the outputs will mirror those shortcomings, leading to inaccuracies that feel eerily plausible. Overfitting is another culprit—when AI gets too cozy with its training data, it struggles with anything new, churning out errors instead.
Poor prompt design plays a big role too; vague questions can leave the AI guessing, filling in gaps with fabrications. Essentially, without a true grasp of context like humans have, AI just patterns matches, which is why AI hallucinations pop up even in sophisticated setups. It’s a reminder that for all their smarts, these systems have limits we need to address.
Comparing AI Hallucinations to Human Mistakes
It’s helpful to contrast these with human errors to see the differences. While people might forget details or get biased, AI takes it a step further by fabricating entire elements that seem credible at first glance.
AI Hallucinations | Human Errors |
---|---|
Stem from algorithms without real comprehension, often inventing facts | Arise from memory slips or biases, but rarely create new falsehoods |
Can spread rapidly across millions of interactions | Are more contained, limited by individual experiences |
Might slip by unnoticed without checks | Often get questioned in conversations |
This table shows how AI hallucinations can amplify problems at scale, making them a bigger concern in fields like journalism or healthcare.
The Risks Tied to AI Hallucinations
As AI weaves into more aspects of life, the dangers of these hallucinations grow. Misinformation is a key issue—false info from AI can ripple out, eroding trust in everything from news to social media. For businesses, this means potential legal headaches or reputational hits if AI-generated content misleads customers.
Think about decision-making in critical areas; faulty AI advice in finance or medicine could lead to serious fallout. Even in SEO, where accurate content is king, AI hallucinations might tank your site’s credibility and search rankings. Have you considered how one wrong AI output could snowball into bigger problems?
Do Smarter Models Reduce AI Hallucinations?
With advancements like GPT-4o, we’ve seen some progress in curbing these errors, but it’s not a total fix. Newer models handle routine tasks better, yet they still stumble on complex or rare queries, spitting out confident mistakes. For instance, they might nail simple facts but falter on nuanced math or invent sources for edge cases.
While improvements in AI architecture help, the core challenge persists: these systems predict based on patterns, not knowledge. So, even as we push for smarter AI, AI hallucinations remain a hurdle we can’t ignore just yet.
Strategies to Prevent AI Hallucinations
Thankfully, there are ways to keep these issues in check. Start with human oversight—always have a person review AI outputs, especially for important stuff like reports or public content. Crafting clear, detailed prompts can also make a difference, guiding the AI away from guesswork.
Fact-checking is non-negotiable; verify sources and avoid blind trust in AI. For organizations, fine-tuning models with quality data and staying transparent about AI use can minimize risks. What if you built a routine where every AI response gets a quick human double-check? It’s simple but effective.
Best Practices for Handling AI in Your Organization
Here are some actionable steps: Set up strong review processes, integrate fact-checking tools, and train your team on AI’s limitations. Keep an eye on evolving regulations to stay compliant, and encourage a culture where AI is a tool, not a crutch.
By doing this, you not only cut down on AI hallucinations but also build more reliable systems overall.
The Path to More Reliable AI
Looking ahead, researchers are focusing on better data handling and advanced training to make AI less error-prone. Innovations like improved transformers and alignment techniques are promising, but we’ll always need a blend of AI’s efficiency and human insight.
Ultimately, the goal is a future where technology supports us without these pitfalls, but for now, collaboration is key. Imagine a world where AI and humans team up seamlessly—it’s closer than you think.
Wrapping It Up
In summary, while AI keeps evolving, AI hallucinations are still a reality we must navigate. By understanding their roots and implementing smart strategies, we can use AI more safely and effectively. Whether you’re in tech, business, or just curious, staying informed is your best move.
What are your thoughts on AI’s quirks? Share your experiences in the comments, or check out our other posts on emerging tech trends. Let’s keep the conversation going!
References
- IBM Think. “AI Hallucinations.” IBM. Accessed 2023.
- Wikipedia. “Hallucination (artificial intelligence).” Wikipedia. Accessed 2023.
- DataCamp Blog. “AI Hallucination.” DataCamp. Accessed 2023.
- Cloudflare Learning. “What Are AI Hallucinations?” Cloudflare. Accessed 2023.
- Sify Technologies. “The Hilarious and Horrifying Hallucinations of AI.” Sify. Accessed 2023.
- Surfer SEO Blog. “AI Hallucination.” Surfer SEO. Accessed 2023.
- Grammarly Blog. “What Are AI Hallucinations?” Grammarly. Accessed 2023.
- Ovrdv Blog. “SEO Techniques for AI-Generated Content.” Ovrdv. Accessed 2023.
AI hallucinations, AI hallucinations in smart models, generative AI errors, AI misinformation, causes of AI hallucinations, risks of AI hallucinations, preventing AI hallucinations, large language models, AI content strategies, smarter AI models