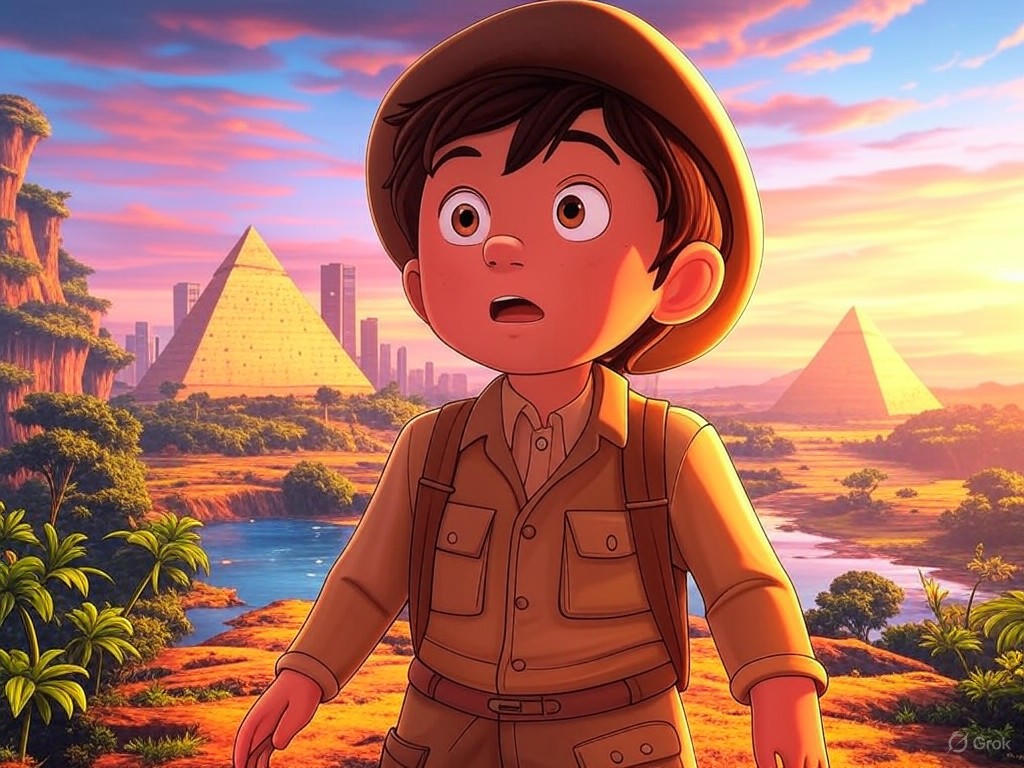
AI Hallucinations Worsening Despite Powerful AI Advances
Understanding AI Hallucinations
AI hallucinations are becoming a major concern as AI systems evolve. These occur when generative AI, like large language models, generate factually incorrect or fabricated information that seems believable at first glance.1 Have you ever asked a chatbot a simple question and received a confidently wrong answer? It’s more common than you might think, especially in text-based tools.
This issue isn’t limited to words; it affects images and videos too, but text outputs from AI chatbots pose the biggest risks for misinformation. As AI gets smarter, these errors highlight a growing gap between capability and accuracy.
What Really Counts as an AI Hallucination?
AI hallucinations involve any output that mixes false or misleading details with a veneer of truth. For instance, an AI might invent a historical fact or misquote a source, all while sounding utterly convincing.4 This can range from small slip-ups, like wrong dates, to elaborate fabrications that lead users astray.
- Examples include fabricated statistics or references that don’t exist.
- They often appear in responses that are logically structured but detached from reality.
- Imagine relying on an AI for travel advice and getting a completely made-up hotel recommendation—it’s frustrating and potentially harmful.
The key problem? AI delivers these with unearned confidence, making it tough for users to spot the lies. Does this sound like a recipe for distrust? It absolutely is.
Why Are AI Hallucinations on the Rise?
Even with groundbreaking AI advances, AI hallucinations are worsening due to the sheer scale and complexity of modern models.1 As developers push for more powerful systems, unintended flaws emerge. Let’s break this down to see what’s driving the problem.
The Role of Insufficient Training Data
One major factor is the quality of training data. Large language models learn from vast datasets, but if that data is biased, outdated, or incomplete, the AI starts filling in the blanks with inventions.1 For example, in niche topics like rare medical conditions, an AI might generate plausible but wrong details because it’s never seen the full picture.
- This leads to more errors in underrepresented areas, amplifying misinformation risks.
- Think about how cultural biases in data could skew responses on global events—it’s a real-world issue affecting everyday use.
Overfitting in Complex Models
As AI models grow, overfitting becomes a sneaky culprit. This happens when models memorize patterns instead of truly understanding them, causing AI hallucinations during new or ambiguous queries.3 It’s like cramming for a test without grasping the concepts—great for familiar questions, disastrous for the unexpected.
Deeper architectures meant to boost performance often backfire, making errors more frequent. How can we balance innovation with accuracy? It’s a question researchers are grappling with daily.
Flaws in How AI Generates Content
At their core, AI systems aim for the most probable response, not the truthful one. They predict words based on patterns, lacking any built-in fact-checker.5 This means an AI might craft a story that’s linguistically perfect but entirely fictional.
- Without mechanisms to verify information, outputs can drift far from reality.
- A hypothetical scenario: Asking an AI about a recent scientific study could yield a detailed summary that’s completely fabricated—scary, right?
Real-World Examples and the Spread of AI Hallucinations
Research paints a clear picture of how widespread AI hallucinations have become. By 2023, studies showed chatbots hallucinating in nearly 27% of interactions, with factual errors in almost half of generated texts.4 That’s not just a statistic—it’s a wake-up call.
- ChatGPT, for instance, incorrectly attributed quotes in 76% of tests from journalism sites, often without admitting uncertainty.5
- In legal AI tools, errors appeared in at least one in six queries, potentially leading to flawed decisions.
- Consider a business analyst using AI for market forecasts; if the data is wrong, it could mean poor investments and real financial losses.
These examples show why AI hallucinations aren’t just technical glitches—they’re impacting decisions in profound ways.
Key Industries Facing the AI Hallucinations Challenge
From healthcare to education, AI hallucinations are infiltrating critical sectors and raising alarms. In healthcare, for example, an AI might suggest incorrect treatments based on flawed data, putting lives at risk.7
- Legal professionals deal with fabricated case laws that could derail cases.
- Journalists face issues with misquotes, eroding public trust in media.
- In education, students might absorb inaccurate knowledge, hindering learning.
- Businesses rely on AI for analytics, but wrong forecasts can lead to costly mistakes.
The fallout? Eroded trust and safety concerns. What if your doctor’s AI-assisted diagnosis was based on a hallucination? It’s a scenario we can’t ignore.
Is Eliminating AI Hallucinations Even Possible?
Pinning down a solution to AI hallucinations is one of AI’s toughest challenges. Current models prioritize fluent outputs over facts, making complete eradication elusive.5 Researchers are innovating, but progress is slow.
Strategies to Tackle AI Hallucinations
Ongoing efforts include curating better training data and adding fact-checking layers to AI systems.1 For high-stakes areas like medicine, fine-tuning models could reduce risks.
- User tools like uncertainty indicators help flag potential errors.
- One emerging approach is integrating plugins that cross-reference responses with reliable sources—think of it as giving AI a built-in editor.
- While these methods improve things, they don’t fully solve the problem as models keep advancing.
The big question: Will we ever have AI that’s both powerful and perfectly reliable? It’s an exciting frontier, but we’re not there yet.
Practical Tips for Dealing with AI Hallucinations
Until tech catches up, here’s how to minimize the impact of AI hallucinations in your daily use. Always double-check AI outputs against credible sources—it’s a simple habit that saves time and trouble.6
- Treat AI as a helpful draft tool, not the final word; edit its suggestions thoroughly.
- Provide feedback to AI platforms to help them learn and improve.
- Incorporate human oversight, especially for important tasks like writing reports or making decisions.
- For professionals, consider hybrid workflows where AI assists but humans verify—it’s a balanced approach that builds trust.
These steps aren’t just precautions; they’re essential for safe AI integration. How do you use AI in your work? Experimenting with these tips could make a big difference.
Wrapping Up the AI Hallucinations Discussion
As AI continues to advance, AI hallucinations remain a persistent hurdle, growing alongside the technology. The key to progress lies in combining smarter engineering with user vigilance and ethical practices.
By staying informed and applying these strategies, we can foster more reliable AI experiences. What are your thoughts on this issue? Share in the comments, explore our related posts on AI ethics, or spread the word to help build a more trustworthy digital world.
References
- [1] “AI Hallucination” by DataCamp, https://www.datacamp.com/blog/ai-hallucination
- [2] “What Are AI Hallucinations?” by Descript, https://www.descript.com/blog/article/what-are-ai-hallucinations
- [3] “AI Hallucinations” by IBM, https://www.ibm.com/think/topics/ai-hallucinations
- [4] “Hallucination (Artificial Intelligence)” on Wikipedia, https://en.wikipedia.org/wiki/Hallucination_(artificial_intelligence)
- [5] “AI Hallucinations” by Nielsen Norman Group, https://www.nngroup.com/articles/ai-hallucinations/
- [6] “AI Article Writer” by RyRob, https://www.ryrob.com/ai-article-writer/
- [7] “AI Hallucinations” by Coursera, https://www.coursera.org/articles/ai-hallucinations
- [8] YouTube video on AI hallucinations, https://www.youtube.com/watch?v=aQJ0m5nD6-4
AI hallucinations, language models, generative AI, misinformation, AI reliability, AI advances, trust in AI, AI errors, neural networks, ethical AI