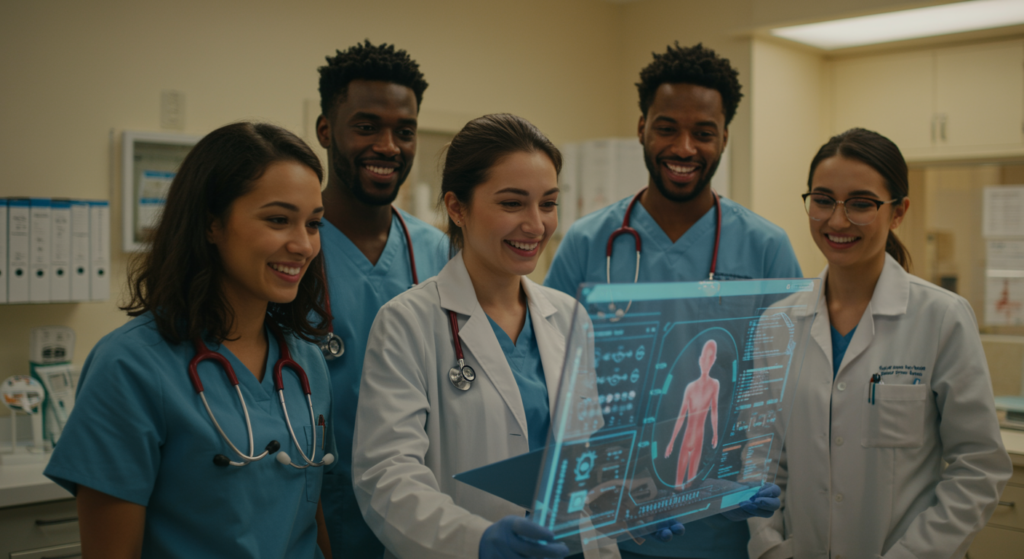
AI in Healthcare: 10 Best Practices for AI Implementation
Understanding AI’s Transformative Potential in Healthcare
AI in Healthcare is reshaping how we deliver care, from diagnostics to daily operations. As systems worldwide grapple with rising costs and the need for better outcomes, these technologies offer smart ways to boost accuracy in diagnoses, tailor treatments, and optimize workflows. Imagine a world where AI helps predict patient needs before issues arise, making value-based care more achievable and efficient.
This shift doesn’t come easy; it requires careful strategy to align with goals like improving patient experiences and cutting expenses. From predictive tools that anticipate disease progression to personalized plans that encourage adherence, AI in Healthcare is key to addressing big challenges. Let’s dive into the 10 best practices that can help organizations implement AI smoothly, focusing on trust, ethics, and real-world results.
1. Prioritizing Data Quality and Security in AI in Healthcare
At the heart of successful AI in Healthcare lies top-notch data quality and rock-solid security. Without clean, reliable data, even the smartest algorithms can falter, leading to errors that no one wants in patient care.
Think about it: Healthcare organizations need to build strong data governance to ensure information is accurate and inclusive of diverse groups. This means regular data cleaning, standardization, and checks to avoid biases that could skew results. Have you ever wondered how a small data error might affect a life-changing diagnosis?
On the security front, protecting sensitive health data is non-negotiable. Implement advanced encryption, strict access controls, and audit trails, while staying compliant with laws like HIPAA. By getting this right, you’re setting the stage for AI systems that clinicians and patients can truly trust.
2. Ensuring Ethical Development and Use of AI in Healthcare
Ethics isn’t an afterthought when it comes to AI in Healthcare; it’s the backbone of every step. Organizations should form teams with experts from various fields to guide AI development and keep things fair and transparent.
Core principles like transparency—helping users understand AI decisions—and fairness, by testing for biases across demographics, are essential. For instance, what if an AI tool overlooks certain patient groups? Accountability ensures clear ownership of decisions, while privacy keeps data safe.
Regular ethical audits can catch issues early, building trust and avoiding pitfalls. In AI in Healthcare, this approach not only protects reputations but also fosters a more equitable system for everyone involved.
3. Investing in Training for AI in Healthcare Professionals
Even the best AI tools won’t shine if healthcare teams aren’t equipped to use them. That’s why investing in comprehensive training is a game-changer in AI in Healthcare.
Start with basic AI literacy for all staff, then move to tailored sessions for specific roles, like hands-on workshops for clinicians. Picture a nurse practicing AI-driven predictions in a simulated scenario—it’s practical and empowering. Don’t forget ongoing education to keep up with AI’s rapid evolution, and appoint “AI champions” to lead the way.
This strategy speeds up adoption and turns potential skeptics into advocates, making AI in Healthcare a seamless part of the team.
4. Validating AI Systems in Real Clinical Contexts of AI in Healthcare
Before rolling out AI widely, thorough validation in actual clinical settings is crucial for AI in Healthcare. Lab tests are one thing, but real-world performance tells the full story.
This process involves pilot tests, comparisons with standard practices, and checks across diverse patient groups. Why not evaluate how an AI tool performs in a busy ER versus a controlled environment? Documenting results builds credibility and ensures the technology delivers on its promises.
By focusing on metrics like accuracy and patient outcomes, organizations can confirm that their AI in Healthcare investments are worth it.
5. Seamlessly Integrating AI into Workflows for AI in Healthcare
One common hurdle in AI in Healthcare is making new tools fit into existing routines without causing chaos. The key is integration that feels natural and adds value right away.
Map out current workflows with input from frontline staff to spot inefficiencies AI can fix. For example, imagine an AI system that delivers insights directly into an EHR at the perfect moment. Starting small with targeted use cases helps build momentum and user buy-in.
This thoughtful approach ensures AI in Healthcare enhances efficiency without overwhelming teams.
6. Implementing Ongoing Performance Monitoring in AI in Healthcare
AI isn’t a set-it-and-forget-it solution; continuous monitoring is vital in AI in Healthcare to keep things effective and adaptive. Track metrics like accuracy, user satisfaction, and cost savings regularly.
Set clear benchmarks for intervention, such as retraining algorithms if performance dips. A real-world example: A hospital notices declining predictions and adjusts based on new data trends. Involving multidisciplinary teams in reviews keeps AI aligned with evolving needs.
This practice turns AI in Healthcare into a dynamic tool that improves over time.
7. Augmenting Human Expertise with AI in Healthcare
The best AI in Healthcare setups treat technology as a partner, not a replacement for human insight. AI handles data-crunching tasks, while professionals bring empathy and judgment to the table.
For instance, AI might spot patterns in scans that doctors could miss, freeing them for deeper patient interactions. Have you considered how this collaboration could reduce burnout and elevate care quality? As AI in Healthcare evolves, it empowers clinicians to focus on what matters most.
By emphasizing augmentation, organizations create a balanced system that leverages the strengths of both tech and people.
8. Developing Governance Frameworks for AI in Healthcare
Strong governance is the glue that holds AI in Healthcare implementations together, outlining roles and processes from start to finish. This includes policies for data handling, updates, and incident responses.
A diverse committee—spanning clinicians, IT experts, and ethicists—ensures decisions are well-rounded. For example, what if an AI update affects patient privacy? Clear protocols prevent issues and promote responsibility.
In AI in Healthcare, solid governance builds a foundation for ethical, scalable innovation.
9. Embracing Predictive Analytics Within AI in Healthcare
Predictive analytics is a standout feature of AI in Healthcare, using data to foresee risks and enable proactive interventions. This tool can flag patients at risk of readmission or help manage outbreaks efficiently.
Imagine predicting medication adherence challenges and intervening early to improve outcomes. In value-based care, this means better results at lower costs. Always pair these insights with action plans to turn predictions into real benefits.
By integrating predictive analytics, AI in Healthcare shifts the focus from reactive to preventive care.
10. Planning for Long-Term Innovation in AI in Healthcare
Treating AI in Healthcare as an ongoing journey rather than a one-off project is essential for lasting success. This involves securing funding, evaluating new applications, and forming partnerships for growth.
For instance, scaling a successful pilot across facilities requires playbooks and shared knowledge networks. What steps could your organization take to stay ahead in this fast-paced field? These strategies ensure AI evolves alongside healthcare needs.
Ultimately, thoughtful planning makes AI in Healthcare a sustainable force for positive change.
Conclusion: The Road Ahead for AI in Healthcare
As we look to the future, AI in Healthcare will play a pivotal role in advancing value-based models. By adopting these practices, organizations can enhance decision-making, streamline operations, and deliver more personalized care.
Remember, success comes from viewing AI as part of a broader strategy, blending tech with human expertise. From precision imaging today to personalized medicine tomorrow, the possibilities are exciting. If you’re in healthcare, what are your thoughts on this? Share your experiences in the comments, explore our related posts, or connect with experts to dive deeper.
References
- American Journal of Managed Care. “Best Practices for Implementing AI into Value-Based Care.” Source
- PMC. “Articles on AI in Healthcare.” Source
- STRSI. “AI in Healthcare: 5 Best Practices.” Source
- Stanford Online. “How to Bring Artificial Intelligence to Healthcare Safely and Ethically.” Source
- DIME Society. “AI Implementation Playbook for Care Delivery.” Source
- Foreseemed. “Artificial Intelligence in Healthcare.” Source
- Harvard T.H. Chan School of Public Health. “Implementing Health Care AI into Clinical Practice.” Source
- Article Forge. General AI Resources. Source
AI in Healthcare, value-based care, AI implementation in healthcare, best practices for AI, artificial intelligence in healthcare, predictive analytics in healthcare, patient outcomes, operational efficiency, ethical AI in healthcare, healthcare innovation