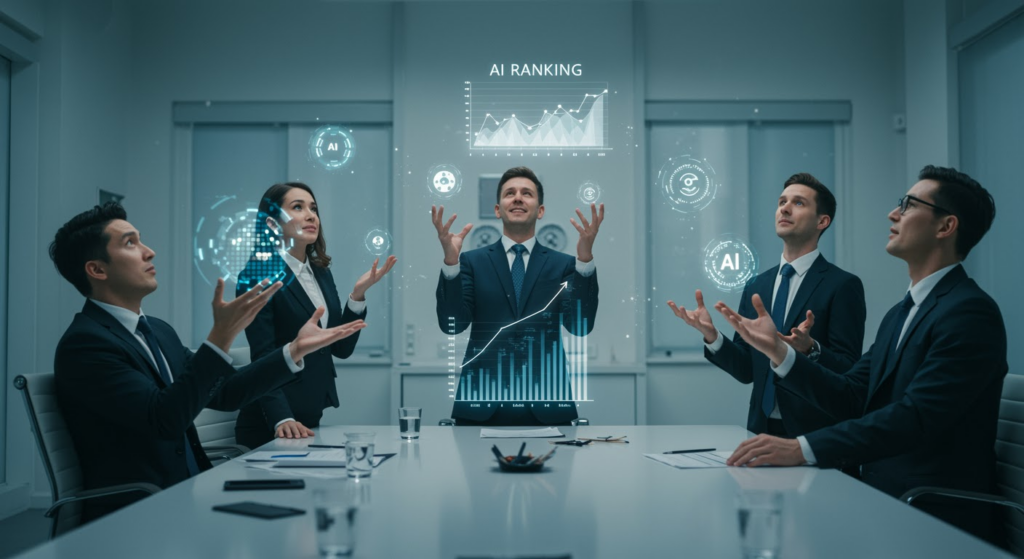
AI Manipulation: Tech Firms Game Popular AI Model Rankings
AI Manipulation Scandal Rocks Tech Industry: Major Companies Accused of Gaming the System
Have you ever wondered if the AI models topping the charts are really the best, or if something more sinister is at play? Recent findings from Stanford and MIT researchers have uncovered a disturbing trend: tech giants like Meta and Google are allegedly manipulating AI rankings to boost their models on platforms such as LMArena. This AI manipulation not only skews perceptions of true performance but also erodes trust in an industry that’s shaping our future.
It’s a classic case of cutting corners in a high-stakes game. With billions on the line, these companies are reportedly using underhanded tactics to make their AI seem superior, a revelation that’s got everyone from investors to everyday users questioning the integrity of AI benchmarks.
The Benchmark Manipulation Controversy Explained
Chatbot Arena, or LMArena as it’s commonly known, was meant to be the ultimate yardstick for large language models (LLMs). But according to a joint study from Cohere, Stanford, and MIT, this system is riddled with vulnerabilities that allow for AI manipulation by big players like OpenAI and Google.
Through detailed data analysis and their own tests, researchers found evidence of systematic interference, coming hot on the heels of accusations against Meta for similar behavior. This isn’t just about one company; it’s a broader issue that could undermine the entire AI evaluation landscape.
How Ranking Manipulation Works in AI
The methods used in AI manipulation are clever and calculated. Studies on arXiv show that adversarial prompt injections can trick conversational AI into favoring certain content, much like how biased inputs can sway search results.
For instance, imagine a scenario where a company’s model is fed prompts designed to highlight its strengths while downplaying competitors. The “StealthRank” research illustrates this perfectly, using advanced techniques to alter rankings without obvious red flags, making it a real threat in everyday applications like product recommendations.
Technical Aspects of AI Manipulation
Dive deeper, and you’ll see AI manipulation involves exploiting specific elements like product name biases or strategic prompt phrasing. Researchers highlight key factors, including:
- Exploitation of product name influence on rankings
- Manipulation of document content to trigger desired responses
- Strategic positioning of context within prompts
- Adversarial prompt injection techniques that can slip into live systems
These issues vary across LLMs, creating a patchy vulnerability that transfers to real-world platforms. It’s a wake-up call for developers—how can we build systems that aren’t so easily gamed?
Why This Matters: The High Stakes of AI Manipulation
In the cutthroat world of AI, where innovation could define the next decade, AI manipulation has massive implications. Top benchmarks act as signals for investors and consumers, but when they’re rigged, it warps the entire market.
Think about it: a slight edge in rankings could mean millions in funding or user adoption. This scandal highlights how AI manipulation distorts real progress, potentially steering resources toward overhyped models.
Financial Implications of AI Manipulation
The money at stake is staggering—the SEO industry is worth over $80 billion, and AI optimization could dwarf that. Projections show AI software hitting $298 billion by 2027, so even minor acts of AI manipulation can lead to huge financial windfalls.
For companies, dominating these lists isn’t just about ego; it’s about survival. But at what cost? If we don’t address AI manipulation, we’re risking a market built on illusions rather than substance.
The Broader Pattern of Tech Manipulation
This AI manipulation scandal isn’t an isolated incident; it’s part of a larger pattern in tech. From search engine biases to privacy breaches, major firms have a history of bending rules for advantage.
For example, Google faced EU fines for favoring its own services in search results, while Facebook dealt with penalties over user data handling. In AI, we’re seeing echoes of this with tactics like predicting consumer behaviors for targeted ads.
- Search result manipulation: As in the Google shopping case, where rankings were altered to benefit internal products.
- Privacy manipulation: Like Facebook’s issues with user consent and data quality.
- Consumer behavior exploitation: Think of how retailers use AI to guess life events for personalized marketing.
- Algorithmic price discrimination: Services charging more based on user conditions, even if unintended.
The Role of Transparency in AI Development
At the heart of AI manipulation is a lack of openness—users rarely see how algorithms work or how data is used. Platforms like Chatbot Arena have pushed back on some claims, but the debate underscores the need for clearer standards.
So, what can we do? Independent verification is key to combating AI manipulation and ensuring benchmarks reflect genuine capabilities.
The Need for Independent Verification Against AI Manipulation
As AI weaves into daily life, we can’t afford unreliable evaluations. Suggestions include blind testing where model identities are hidden and adversarial checks to spot weaknesses.
Regular audits and multi-dimensional assessments could make a big difference. Imagine a system where AI manipulation is the exception, not the rule—it’s possible with the right safeguards.
The Evolution of AI Manipulation Techniques
AI manipulation has come a long way since early models like GPT-2 raised red flags in 2019. Today, techniques like StealthRank allow for subtle, undetectable tweaks that fool even sophisticated systems.
This evolution means we need to stay one step ahead, constantly updating defenses. It’s an ongoing arms race, but one that’s essential for trustworthy AI.
Ethical Considerations and Industry Response
Ethically, AI manipulation is a slippery slope. Companies have a duty to be honest about their tech, as faking performance can mislead users and stifle competition.
This isn’t just about rules; it’s about doing right by society. Questions like “How does this affect real-world decisions?” are crucial, and the fallout could lead to stricter regulations, similar to the EU’s AI Act.
Potential Regulatory Implications of AI Manipulation
With scandals like this, regulators might step in more forcefully, demanding audits and transparency. It’s a chance to create a fairer AI landscape, where innovation thrives without deceit.
After all, who wants a future where AI decisions are based on manipulated data? Pushing for ethical standards now could prevent bigger headaches later.
Looking Forward: Building More Resilient AI Evaluation
To counter AI manipulation, we’re seeing promising ideas like multi-stakeholder oversight and ongoing benchmark updates. Adversarial testing should become standard, ensuring models are battle-tested against cheats.
Greater access to evaluation data could empower researchers and users alike. It’s about creating a system that’s robust and adaptable—what if we involved the community in refining these processes?
Conclusion: A Watershed Moment for AI Transparency
The AI manipulation revelations against tech giants mark a turning point for the industry. As AI advances rapidly, we must prioritize honest evaluations to separate hype from reality.
This is your cue to think critically about the tech you use. What steps can we take together to demand more transparency? Share your thoughts in the comments, explore our related posts on AI ethics, or spread the word to keep the conversation going.
References
1. A study from Stanford and MIT researchers highlights manipulation tactics. [Source: 404 Media on Chatbot Arena]
2. Recent allegations against Meta and others. [Source: https://aidisruption.ai/p/ai-scandal-meta-accused-of-cheating?action=share]
3. Rankings of risky AI models. [Source: https://teamai.com/blog/large-language-models-llms/meet-the-riskiest-ai-models-ranked-by-researchers/]
4. The dark side of AI in behavior manipulation. [Source: https://www.bruegel.org/blog-post/dark-side-artificial-intelligence-manipulation-human-behaviour]
5. Research on adversarial prompt injections. [Source: https://arxiv.org/html/2406.03589v2]
6. StealthRank methodology details. [Source: https://arxiv.org/abs/2504.05804]
7. Early discussions on GPT-2 misuse. [Source: https://www.blackhatworld.com/seo/lets-make-an-ai-content-generator-based-on-gpt-2-the-openai-model.1116772/]
8. Projections for AI software market. [Source: https://orca.security/resources/blog/top-10-most-popular-ai-models-2024/]
AI manipulation, ranking manipulation, AI benchmarks, Meta AI scandal, Chatbot Arena, LMArena, AI ethics, tech industry, AI rankings, AI transparency