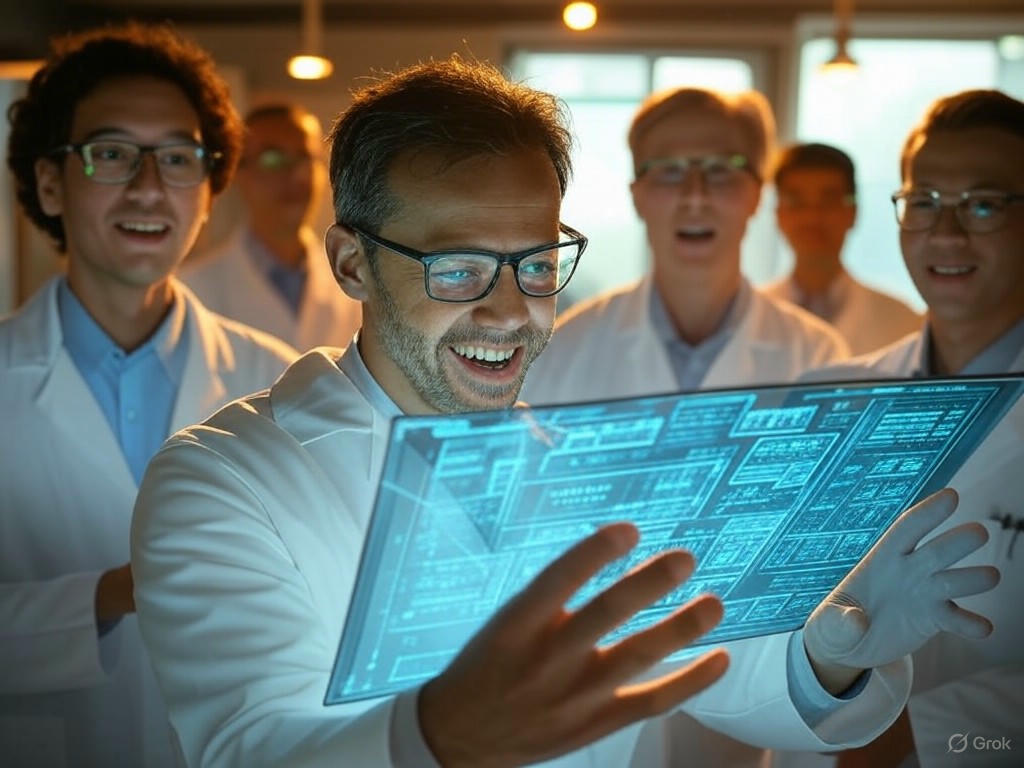
AI-Powered Science Acceleration at Berkeley Lab
The AI Revolution Transforming Scientific Research at Berkeley Lab
AI for science is reshaping the landscape of discovery, and Berkeley Lab is leading the charge with innovative tools that blend artificial intelligence, automation, and advanced data processing. Have you ever imagined how quickly we could solve energy crises if research moved at lightning speed? By harnessing these technologies, researchers are processing vast datasets faster than ever, enabling breakthroughs in fields from materials science to particle physics.
This approach isn’t just about speed—it’s about opening doors to innovations that traditional methods couldn’t touch. For instance, AI-driven systems at Berkeley Lab are helping scientists analyze complex data patterns, ensuring the U.S. stays ahead in global scientific leadership. As a result, what once took months of manual work can now happen in hours, making AI for science a game-changer for the entire research community.
Building the Automated Laboratory of the Future
At the heart of Berkeley Lab’s efforts is the A-Lab, an automated system that’s a prime example of AI for science in action. This robotic setup tackles one of research’s biggest hurdles: testing new materials at a pace that keeps up with theoretical predictions. Picture this—a lab that works non-stop, synthesizing and evaluating hundreds of samples daily without human oversight.
Traditionally, verifying a predicted material for applications like better batteries has been slow and labor-intensive. But A-Lab flips that script, handling 50 to 100 times more samples than a human could in the same timeframe. By drawing from resources like Berkeley Lab’s Materials Project and Google DeepMind’s GNoME, it creates chemical recipes that evolve through continuous learning.
How AI for Science Accelerates Discovery in A-Lab
AI for science shines in A-Lab’s closed-loop design, where robots make decisions based on real-time data, freeing scientists to focus on big-picture ideas. For example, if a recipe doesn’t work, the system adjusts instantly, learning from failures to refine the next attempt. This not only boosts efficiency but also minimizes errors that could derail projects.
Researchers at Berkeley Lab emphasize that this automation lets them innovate without getting bogged down in routine tasks. What if every lab could operate like this? It’s a step toward a future where material discoveries happen exponentially faster, potentially leading to cleaner energy solutions sooner than we thought.
Cross-Disciplinary AI Applications Advancing Science
Beyond materials, AI for science is making waves across disciplines at Berkeley Lab, from electronics to environmental research. Teams are using advanced AI tools to model complex systems, like particle interactions, which were once too cumbersome for traditional computing. This cross-pollination of ideas ensures that U.S. science remains a global frontrunner.
Have you considered how AI could enhance your own field? At Berkeley Lab, it’s enabling new investigations that blend physics, biology, and computing in unexpected ways. By optimizing experiments and data analysis, researchers are uncovering insights that drive real-world applications, such as more efficient solar cells or safer medical technologies.
The 1,000 Scientist AI Jam: Fueling Collaborative AI for Science Innovations
In early 2025, Berkeley Lab hosted the groundbreaking 1,000 Scientist AI Jam, a collaborative event that highlighted the potential of AI for science on a national scale. Bringing together 1,500 experts from various labs, participants tested models from OpenAI and Anthropic on real scientific problems, fostering hands-on problem-solving. Ana Kupressanin, Director of Berkeley Lab’s Scientific Data Division, likened AI to a telescope for research, saying, “It allows us to see beyond what the naked eye can grasp, transforming how we interpret data.”
This event wasn’t just about testing tech; it was about building a community. Attendees worked in teams to apply AI to challenges in their domains, revealing how tools like large language models can predict outcomes in climate modeling or drug discovery. If you’re in research, events like this show how AI for science can spark collective creativity and accelerate progress.
NERSC: Supercomputing Power Boosting AI for Science
The National Energy Research Scientific Computing Center (NERSC) at Berkeley Lab is a powerhouse for AI for science, providing supercomputing resources that turn ambitious ideas into reality. Its Perlmutter supercomputer is key to running complex AI simulations that handle massive datasets, from climate patterns to quantum mechanics. In 2025, NERSC opened calls for proposals to use this system for cutting-edge projects.
Selected initiatives get substantial computing time, up to 10,000 GPU node hours, which can process data at speeds that human analysis can’t match. This support is crucial for maintaining U.S. leadership in AI-driven research, as it allows scientists to experiment without resource constraints.
Generating AI-Ready Datasets for Scientific Advancement
Beyond raw power, NERSC focuses on creating AI-ready datasets, another facet of AI for science that’s often overlooked. Researchers can apply for CPU node hours to develop datasets that become publicly available, benefiting the wider community. For instance, a project might generate data on energy materials that others can use to train new models.
This strategy ensures high-quality inputs for AI systems, making them more accurate and reliable. Imagine accessing a shared library of datasets—it’s like giving every scientist a head start on their work.
Training the Next Generation of AI Scientists
Berkeley Lab understands that AI for science needs skilled people behind it, so they’re offering programs like the 2025 Deep Learning for Science Summer School. Running from June 23-27, this event covers everything from transformers to generative AI, with hands-on sessions on high-performance computing. It’s designed for researchers and engineers eager to apply these tools to real problems.
If you’re looking to upskill, this could be your opportunity. The curriculum includes interactive labs led by experts, teaching how to build and deploy AI models for scientific tasks. What tips would you take away? Attendees learn best practices, like scaling models efficiently, which can transform how you approach data-intensive projects.
Competitive Advantage in the Global AI Landscape
By integrating AI for science, Berkeley Lab is helping the U.S. stay competitive amid global AI investments. This synergy of human expertise and machine intelligence creates faster, more precise research outcomes. For example, in a world racing to develop sustainable tech, Berkeley’s methods could mean the difference in bringing innovations to market first.
It’s not just about technology—it’s about blending creativity with computation. A hypothetical scenario: What if AI helped design a new vaccine in record time during a health crisis? That’s the kind of edge Berkeley Lab is building.
Future Directions: Expanding the AI Ecosystem
Looking ahead, Berkeley Lab plans to broaden its AI for science ecosystem by incorporating models from more commercial labs, enhancing collaboration and tool diversity. Insights from events like the AI Jam will guide these expansions, ensuring researchers have access to the best options for their needs. This adaptive approach means AI systems can evolve to tackle increasingly complex challenges.
Future sessions might include even more AI variants, allowing scientists to match tools to specific problems, like using diffusion models for material simulations. How could this evolution impact your work in science?
Integrating Theory, Experimentation, and AI for Science Computation
The real power of AI for science lies in its ability to connect theory, experiments, and computation seamlessly. At Berkeley Lab, this integration speeds up the cycle from idea to application, reducing timelines for new technologies. For instance, AI can simulate experiments virtually before physical testing, saving time and resources.
This holistic method promises breakthroughs in areas like energy efficiency, where rapid prototyping is key. It’s a reminder that AI isn’t replacing scientists—it’s empowering them to achieve more.
Conclusion: Transforming the Scientific Enterprise
AI for science at Berkeley Lab is more than a trend; it’s a fundamental shift toward faster, smarter research. From automated labs to superpowered computing, these advancements are paving the way for an energy-abundant future. As we wrap up, consider how this could inspire your own explorations in innovation.
If you’re passionate about science, why not dive deeper? Share your thoughts in the comments, explore related topics on our site, or check out more resources to see how AI is changing the game. Let’s keep the conversation going—what excites you most about AI’s role in science?
References
- Accelerating Science with AI and Automation at Berkeley Lab, LBL News Center.
- Harnessing Artificial Intelligence for High-Impact Science, LBL News Center.
- Harnessing AI for Biosciences, Berkeley Lab Biosciences.
- NERSC AI for Science Call for Proposals 2025, NERSC.
- Deep Learning for Science Summer School 2025, NERSC Training.
- Related AI in Science Video, YouTube (external resource for deeper insights).
- AI Jam Session Details, Berkeley Lab Computing Sciences.
- Additional Research Context, CGA Connecticut.
AI for science, Berkeley Lab AI, scientific automation, material discovery, robotic laboratories, scientific breakthroughs, AI-powered research, automated labs, NERSC supercomputing, deep learning for science