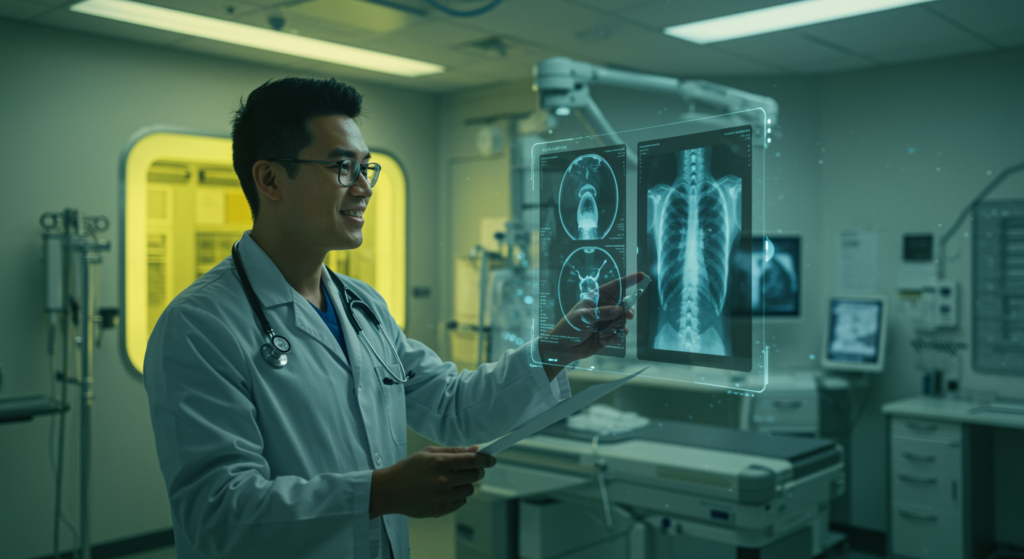
AI and Radiologist Shortage: Pros, Cons, and Solutions
AI in Radiology is transforming how we tackle the global radiologist shortage, offering tools to boost efficiency and accuracy while presenting unique challenges. As healthcare demands grow, this technology could be a game-changer, but it’s essential to understand its full impact. Let’s dive into the key aspects step by step.
Understanding the Radiologist Shortage
The radiologist shortage is a pressing issue in healthcare, with many regions struggling to meet the rising need for imaging services. Factors like an aging population and increasing chronic diseases mean more scans are required, yet there’s a limited pool of trained professionals. AI in Radiology steps in as a potential ally, helping to bridge this gap by automating routine tasks and supporting human experts.
Imagine a busy hospital where delays in reading X-rays lead to longer wait times for patients—it’s a reality in many places. A study from the American College of Radiology highlights that the shortage could worsen without innovative solutions, making AI an intriguing option to explore. Have you ever wondered how technology could ease these bottlenecks?
Pros of AI in Radiology
One of the biggest advantages of AI in Radiology is its ability to handle repetitive tasks with precision, freeing up radiologists for more critical work.
Improved Accuracy and Efficiency in AI in Radiology
AI algorithms excel at scanning medical images quickly, often spotting anomalies that human eyes might overlook. For instance, in analyzing CT scans, AI can process data in seconds, compared to the minutes or hours a radiologist might need, reducing errors and speeding up diagnoses. This not only enhances workflow but also allows professionals to focus on complex cases that require nuanced judgment.
Consider a scenario where a patient needs urgent brain imaging; AI in Radiology could flag potential issues instantly, potentially saving lives. According to research from PMC, AI systems have shown up to 90% accuracy in detecting certain conditions, making them a reliable partner in daily operations.
Enhanced Patient Care through AI in Radiology
By enabling early detection of abnormalities, AI in Radiology plays a vital role in improving patient outcomes. It automates the identification of issues like tumors in mammograms, allowing for timely interventions that could prevent more serious health problems. Radiologists can then dedicate more time to patient interactions, building trust and providing personalized care.
This shift isn’t just about speed—it’s about quality. For example, if AI flags a suspicious spot on a lung scan early, doctors can act quickly, which might mean the difference between a simple treatment and a prolonged battle. What if we could make healthcare this proactive for everyone?
Triage and Workload Management in Radiology with AI
AI in Radiology revolutionizes triage by prioritizing images based on urgency, such as sorting high-risk cases first. This approach can cut down the number of exams needing immediate human review by over 50%, as noted in a Radiology Business report. As a result, radiologists handle less overload, reducing burnout and maintaining high standards.
Picture a radiology department during peak hours: AI steps in to organize the queue, ensuring critical cases don’t slip through the cracks. It’s like having an extra team member who never tires, making the process smoother for everyone involved.
Cons of AI in Radiology
While AI in Radiology offers exciting benefits, it’s not without drawbacks that need careful consideration to ensure safe implementation.
Lack of Human Judgment in AI-Driven Radiology
AI lacks the intuitive understanding that comes from years of clinical experience, which can lead to errors in ambiguous situations. For instance, a system might misinterpret an unusual image due to its reliance on programmed data, potentially missing subtle details that a seasoned radiologist would catch. This highlights why AI should complement, not replace, human expertise.
In a real-world example, during a complex case involving rare conditions, over-reliance on AI could delay accurate diagnosis. How do we balance machine efficiency with human insight to avoid these pitfalls?
Data Quality and Bias Issues in AI in Radiology
The effectiveness of AI in Radiology depends heavily on diverse, high-quality training data. If datasets are biased—say, underrepresented in certain demographics—outcomes could perpetuate inequalities in healthcare. A study from NCBI warns that such biases might lead to inaccurate results for underrepresented groups, emphasizing the need for inclusive data practices.
Think about how this could affect rural or diverse communities: If AI tools aren’t trained on a broad range of cases, they might not perform as well, widening health disparities. Addressing this requires ongoing efforts to refine algorithms and ensure fairness.
Ethical and Regulatory Challenges in AI for Radiology
Implementing AI in Radiology brings up ethical questions, including accountability for errors and compliance with regulations like HIPAA. Who is responsible if an AI misdiagnosis occurs? These concerns can slow adoption, as healthcare providers navigate legal hurdles to protect patients.
For example, a hospital might hesitate to use AI due to potential lawsuits, even if it could improve efficiency. By fostering transparent systems, we can build trust and pave the way for broader use.
Potential Solutions for AI in Radiology
To harness the full potential of AI in Radiology, we need strategies that integrate it thoughtfully into healthcare systems.
Seamless Integration with Workflows in AI in Radiology
Tools like Aidoc’s AI platforms can blend into existing radiology workflows, prioritizing critical images and lightening the load on staff. This not only boosts efficiency but also ensures AI supports radiologists without disrupting routines. By starting with pilot programs, hospitals can test and refine these integrations for optimal results.
Actionable tip: Train your team on AI tools early to maximize benefits. In one case, a clinic reported a 40% reduction in turnaround times after adoption—what could this mean for your practice?
Education and Training Enhancements via AI in Radiology
AI can revolutionize radiology education by providing simulated cases and instant feedback, helping new professionals build skills faster. Programs that use AI for training could shorten the learning curve, addressing shortages by producing competent radiologists more quickly. Universities are already incorporating these technologies to standardize education.
Here’s a relatable scenario: A medical student practices interpreting scans with AI guidance, gaining confidence through repeated, safe practice. This approach not only improves expertise but also makes the field more appealing to newcomers.
Addressing Ethical Concerns in AI for Radiology
Tackling biases and ensuring data privacy are key to making AI in Radiology trustworthy. Developers should implement regular audits and use diverse datasets to minimize errors. Regulatory bodies can set guidelines that promote accountability, turning potential risks into strengths.
One strategy: Partner with ethicists during AI development to anticipate issues. By doing so, we create systems that are both innovative and responsible, fostering a healthier future for all.
Conclusion
Ultimately, AI in Radiology isn’t about replacing radiologists but empowering them to deliver better care amid the shortage. By embracing its pros, mitigating cons, and implementing smart solutions, we can create a more efficient healthcare landscape. As this technology evolves, staying informed and adaptable will be crucial.
What are your thoughts on AI’s role in radiology? Share your experiences in the comments below, or explore more on how it’s shaping healthcare. If you’re interested, check out this resource from Aidoc for deeper insights.
References
- Rayscape AI Article. (n.d.). Rayscape. Link
- AI and the Radiologist Shortage. (2023). Radiology Business. Link
- PMC Article on AI in Radiology. (2023). PMC. Link
- Can AI Replace Radiologists? (2023). Med School Insiders. Link
- Pros and Cons of AI in Radiology. (2023). Statim Healthcare. Link
- Radiology and AI: Pros and Cons. (2023). Microbioz Health. Link
- Additional PMC Study. (2024). PMC. Link
- Radiologist Shortage Blog. (2023). Aidoc. Link
AI in Radiology, Radiologist Shortage, AI Solutions, Pros of AI in Radiology, Cons of AI, Radiology Shortage Solutions, AI in Healthcare, Medical Imaging AI, Ethical AI in Radiology, AI Workload Management