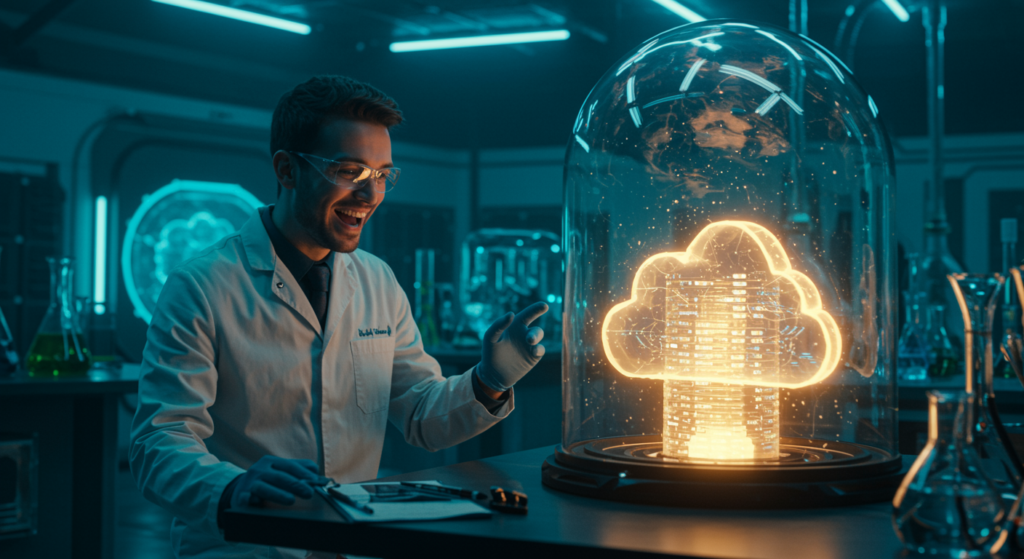
AI Scaling Limitations: Expert Warns It Won’t Boost Intelligence
Introduction: Is the Era of Easy AI Progress Fading?
Have you ever wondered if simply making AI models bigger and more powerful will keep driving breakthroughs? AI scaling limitations are becoming a critical concern, as experts argue that throwing more data and compute at the problem won’t lead to smarter machines. Over the last decade, the strategy of scaling AI has dominated, with massive investments fueling tools like ChatGPT and transforming industries. But now, mounting evidence suggests these AI scaling limitations are hitting hard, forcing a rethink in how we approach artificial intelligence development.
This shift isn’t just theoretical—it’s reshaping the field. As AI scaling limitations tighten, we’re seeing a move toward innovative solutions that could unlock true reasoning capabilities. Let’s dive into why bigger doesn’t always mean better and what the future holds.
The Foundation of AI Scaling Limitations: Cracks in the System
At its core, AI scaling relies on the idea that performance improves with larger models, more data, and greater computational power. Yet, AI scaling limitations are revealing themselves as these gains slow down, challenging the once-reliable “scaling laws.” For years, this approach delivered impressive results, but recent research shows it’s not a magic bullet for achieving human-like intelligence.
Think about it: what if we’ve been chasing size over substance? AI scaling limitations mean that even with exponential growth in resources, fundamental issues in AI reasoning persist, leaving experts like those at OpenAI and Google to question the path forward.
Recent Setbacks: Are We Hitting a Wall in AI Scaling Limitations?
- Projects such as xAI’s Grok 3 have faced significant delays, not due to funding, but because of inherent AI scaling limitations that hinder progress beyond a certain point [5].
- Industry giants like Anthropic and Google are openly acknowledging these AI scaling limitations, shifting focus from sheer size to more efficient strategies [1].
- Experts such as Margaret Mitchell from Hugging Face and Noah Giansiracusa from Bentley University are calling for alternatives, emphasizing that AI scaling limitations demand new approaches to overcome [5].
These examples highlight how AI scaling limitations aren’t just theoretical—they’re impacting real-world innovation. If you’re in AI development, this might make you pause and ask: how can we break through these barriers?
Main Barriers Exacerbating AI Scaling Limitations
1. The Data Dilemma in AI Scaling Limitations
One of the biggest AI scaling limitations stems from data availability. Even the most advanced models are only as good as the data they’re trained on, and as we approach the limits of internet-scale datasets, the quality and diversity start to suffer.
Imagine trying to learn from a library that’s running out of new books—eventually, you’re just rereading the same pages. This data wall exemplifies AI scaling limitations, where diminishing returns make it harder to achieve meaningful improvements in machine intelligence.
2. Compute and Power: Overlooked AI Scaling Limitations
Training cutting-edge AI models demands enormous computational resources, but physical and economic constraints are emerging as major AI scaling limitations. Electricity consumption and chip availability are growing faster than we can sustain, with training compute increasing by about 4x per year.
For context, this outpaces even the rapid growth of past tech revolutions. These AI scaling limitations could force developers to get creative, perhaps by optimizing existing systems rather than scaling up endlessly.
3. Beyond Prediction: Core Flaws in AI Scaling Limitations
The transformer architecture, great for next-token prediction, falls short when it comes to true reasoning—a key aspect of AI scaling limitations. While larger models can handle more complex tasks, they don’t replicate human-like logic, leading to incremental gains at best.
Experts warn that these AI scaling limitations mean we’re stuck in a loop of predictions without deeper understanding. It’s like teaching a child to memorize facts without explaining why they matter—progress stalls eventually.
Expert Insights: Why AI Scaling Limitations Mean Bigger Isn’t Smarter
Ilya Sutskever, former chief scientist at OpenAI, once championed scaling but now stresses that addressing AI scaling limitations requires scaling the right elements, not just more of everything [1].
Margaret Mitchell adds that overcoming AI scaling limitations demands entirely new paradigms for human-like intelligence. And Noah Giansiracusa points out the unsustainability of current trends, urging a shift before these limitations derail the field entirely.
This consensus among experts paints a clear picture: AI scaling limitations aren’t going away on their own. What do you think—could this be the wake-up call AI needs?
Comparing Strategies Amid AI Scaling Limitations
Approach | Strengths | Key AI Scaling Limitations | Current Trend |
---|---|---|---|
Traditional Scaling (Larger Models, More Data/Compute) | Rapid initial progress and better generalization | Resource constraints and lack of true reasoning due to AI scaling limitations | Showing signs of plateauing |
Prompt Engineering and Synthetic Data | Enhances performance without massive scaling | Limited to specific domains, highlighting AI scaling limitations | Increasing as a supplementary tactic |
Inference-Time Search Methods | More efficient resource use in some cases | Doesn’t overcome core AI scaling limitations for intelligence | Still experimental |
Alternative Architectures (e.g., Mixture of Experts) | Potential for flexible, human-like reasoning | Early stage and unproven at scale due to AI scaling limitations | Growing in research interest |
This comparison shows how AI scaling limitations are pushing the industry toward diverse strategies. For instance, hybrid models could be a game-changer, blending symbolic reasoning with deep learning to sidestep these constraints.
Moving Past AI Scaling Limitations: Innovative Paths Forward
To tackle AI scaling limitations, experts are eyeing architectures that prioritize planning and abstraction over raw size. Combining symbolic methods with data-driven AI could finally bridge the gap to advanced intelligence.
Consider using smaller, task-specific models like Mixture of Experts—these not only address AI scaling limitations but also offer practical efficiency. If you’re building AI projects, experimenting with synthetic data curation might be a smart first step to enhance quality without endless scaling.
- Design systems for better logic and abstraction to combat AI scaling limitations.
- Integrate hybrid approaches for more robust machine intelligence.
- Focus on specialized models to navigate these limitations effectively.
Industry Shifts Due to AI Scaling Limitations
AI scaling limitations are prompting a reevaluation of investments, with companies balancing between enhancing current models and pioneering new designs. Startups that innovate around these limitations are attracting more funding, while big players like Google are redirecting R&D toward quality over quantity.
This could mean more opportunities for ethical, efficient AI development. As an AI enthusiast, you might wonder: how can your organization adapt to these changes?
Lessons from AI Scaling Limitations for Content Creators
There’s a parallel in SEO and content creation: just as AI scaling limitations affect model performance, over-relying on AI for mass content production can harm your site’s rankings. Search engines penalize low-quality output, emphasizing the need for human oversight.
To avoid these pitfalls, focus on creating unique, valuable content. Tips include blending AI tools with manual edits and ensuring relevance—after all, overcoming AI scaling limitations in content means prioritizing substance over volume.
Wrapping Up: Embracing Change Beyond AI Scaling Limitations
The era of unchecked AI scaling is evolving, with limitations pushing us toward genuine innovation. As Ilya Sutskever notes, the future lies in creative discoveries, not just bigger models. By addressing AI scaling limitations head-on, we can pave the way for smarter, more sustainable AI.
What are your thoughts on these developments? Share in the comments, explore our related posts on AI innovation, or subscribe for more insights. Let’s keep the conversation going—your ideas could spark the next breakthrough!
References
- [1] “Has AI Scaling Hit a Limit?” Foundation Capital, https://foundationcapital.com/has-ai-scaling-hit-a-limit/
- [3] “Can AI Scaling Continue Through 2030?” Epoch AI, https://epoch.ai/blog/can-ai-scaling-continue-through-2030
- [5] “AI Industry Faces Major Setbacks: Are Scaling Laws Reaching Their Limits?” OpenTools.AI, https://opentools.ai/news/ai-industry-faces-major-setbacks-are-scaling-laws-reaching-their-limits
- [6] “SEO Techniques for AI-Generated Content,” OVRDRV, https://www.ovrdrv.com/blog/seo-techniques-for-ai-generated-content/
- [7] “Researchers Say They’ve Discovered a New Method of Scaling Up AI, But There’s Reason to Be Skeptical,” TechCrunch, https://techcrunch.com/2025/03/19/researchers-say-theyve-discovered-a-new-method-of-scaling-up-ai-but-theres-reason-to-be-skeptical/
- Scaling Laws and Likely Limits to AI, Effective Altruism Forum, https://forum.effectivealtruism.org/posts/LiHdypLRBGrkxWhAH/scaling-laws-and-likely-limits-to-ai
- The State of AI: Strategic Warning, CETAS, https://cetas.turing.ac.uk/publications/state-ai-strategic-warning
- AI-Generated Content and SEO, HubSpot Blog, https://blog.hubspot.com/marketing/ai-generated-content-seo
AI scaling limitations, AI limitations, artificial intelligence development, scaling laws, compute constraints, machine intelligence, AI reasoning, AI scaling laws, AI breakthroughs, AI innovation