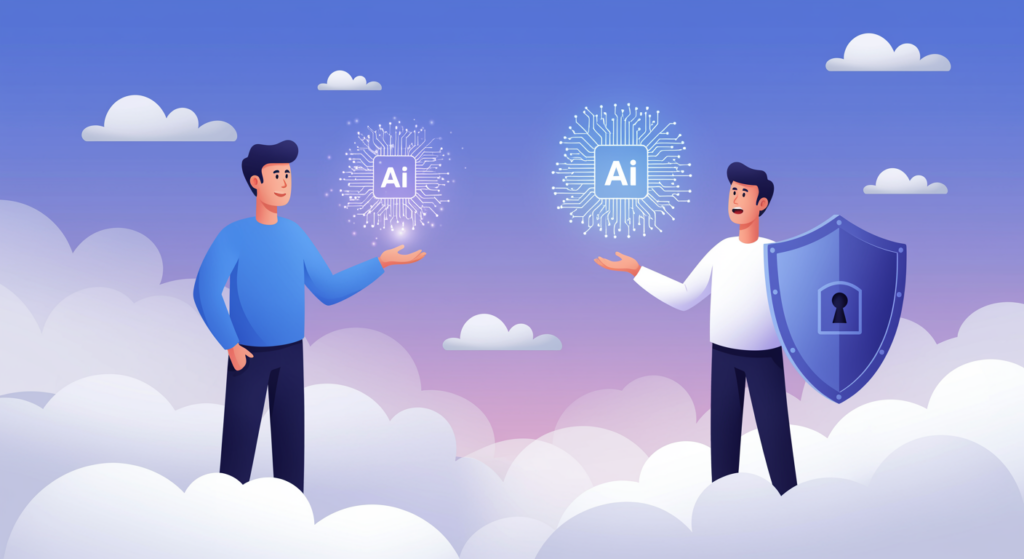
AI Security Paradox in Cloud Native Development
The AI Security Paradox: A Double-Edged Sword
In today’s fast-paced tech world, the AI security paradox looms large as organizations dive into cloud native development. AI tools promise to supercharge productivity, but they also introduce risks that can undermine everything you’ve built. Have you ever wondered how something so innovative could expose your systems to new threats?
Picture this: a developer uses an AI code generator to speed up a project, only to inadvertently introduce a vulnerability that hackers exploit. This is the essence of the AI security paradox—where the same technology that accelerates innovation creates unforeseen security gaps. As we explore this topic, we’ll uncover strategies to harness AI’s benefits while keeping your cloud environments safe.
The Rise of AI in Cloud Native Development
AI is no longer a futuristic concept; it’s reshaping how we approach cloud native development every day. Major platforms like AWS, Azure, and Google Cloud have woven AI into their core services, helping teams automate tasks and innovate faster.
For instance, AI-driven tools can handle repetitive coding, optimize resource use, and even predict potential issues before they arise. A recent survey from Palo Alto Networks shows that 100% of organizations are integrating AI into their development pipelines, highlighting its widespread adoption.
But as AI becomes ubiquitous, the AI security paradox emerges, where efficiency gains clash with heightened vulnerabilities. Think about how AI can analyze vast datasets to improve app performance, yet if not secured properly, it could leak sensitive information.
Understanding the AI Security Paradox
At its heart, the AI security paradox stems from AI’s core strengths: speed, autonomy, and adaptability. These features drive progress but also open doors to sophisticated threats that traditional security can’t always handle.
The Duality of Innovation and Risk in AI Security
On one side, AI boosts efficiency by automating code generation and deployment, letting teams focus on creative problem-solving. Yet, this speed can mask hidden flaws, like bugs in AI-generated code that slip through initial checks.
Attackers are getting smarter too, using AI to craft attacks that evade standard defenses. According to the Palo Alto Networks 2024 report, 44% of organizations worry that the AI security paradox introduces new vulnerabilities, while 43% note that AI-powered threats are dodging conventional tools.
This balance isn’t just theoretical—it’s a real challenge. For example, if an AI system misinterprets data in a cloud native app, it could lead to breaches that cost businesses millions. How can we mitigate these risks without slowing down innovation?
Key Security Risks in AI-Driven Cloud Native Environments
Diving deeper into the AI security paradox, we see risks amplified across the software development lifecycle. AI’s complexity means even small oversights can escalate into major issues.
Take the unpredictability of AI algorithms: they might work flawlessly in tests but falter with real-world data, complicating security testing. Or consider data exposure, where AI processes sensitive information, making it a prime target for leaks or manipulation.
Pipeline attacks are another concern, where automated CI/CD processes could be hijacked to insert malicious code. In one hypothetical scenario, a weak supply chain link lets attackers alter AI-generated components, turning a helpful tool into a liability.
Strategies to Tackle the AI Security Paradox
Facing the AI security paradox head-on requires a proactive mindset. Organizations should adopt layered defenses that integrate security from the start, ensuring AI’s advantages don’t come at the cost of safety.
Shifting Security Left in AI-Enhanced Workflows
One effective approach is to “shift security left,” embedding checks early in the development process. This means using tools for static code analysis right in your IDE or CI/CD pipeline to catch problems before they reach production.
By doing so, you address the AI security paradox by making security a natural part of the workflow, not an afterthought. For teams, this translates to fewer surprises and more reliable deployments.
Continuous Monitoring and Threat Intelligence
In dynamic cloud native setups, real-time monitoring is essential. Advanced observability tools can detect anomalies in AI workloads, flagging potential threats as they emerge.
Imagine an AI system behaving oddly—perhaps due to an attempted breach. With the right setup, you can respond instantly, turning the tables on attackers and resolving the AI security paradox through vigilance.
Strengthening Data and Identity Management
Data security is a cornerstone of overcoming the AI security paradox. Start with granular access controls to limit who can interact with AI services and sensitive datasets.
- Encrypt data both at rest and in transit to prevent unauthorized access.
- Conduct regular audits of permissions and API keys to close potential gaps.
- These steps ensure that even if an attack occurs, the impact is minimized.
A practical tip: Use role-based access to align with the principle of least privilege, reducing the risk of privilege escalation in your cloud native stack.
Fostering a Security-First Culture
The AI security paradox isn’t just technical—it’s cultural. When security becomes everyone’s job, teams are better equipped to handle challenges.
Train developers on AI-specific risks, like how generated code might introduce vulnerabilities, and encourage security champions within groups. This shared responsibility helps blend security with business goals, making it a driver of success rather than a barrier.
Best Practices for Managing AI Security Risks
Risk Area | Best Practices |
---|---|
AI Code Generation | Always review AI-generated code with automated tools and peer reviews to catch issues early. |
Data Storage and Access | Implement real-time threat analysis and data segmentation for better protection against the AI security paradox. |
Model Training and Inference | Apply least privilege principles and use endpoint protection to secure AI infrastructure. |
CI/CD Pipelines | Automate security scans and monitor dependencies to prevent supply chain attacks. |
These practices aren’t just checklists; they’re actionable steps to navigate the AI security paradox effectively.
The Future of AI Security in Cloud Native Development
Looking ahead, the AI security paradox will evolve, but so will our defenses. Organizations that prioritize security alongside innovation will lead the way.
Building a Secure, AI-Ready Tomorrow
The key is to secure your velocity, not just your code, by adapting to emerging threats. What if your team could leverage AI without the constant worry of breaches?
By fostering a culture of continuous improvement, you’ll turn the AI security paradox into an opportunity for growth. Remember, the future belongs to those who innovate securely.
Conclusion
The AI security paradox challenges us to rethink how we build in cloud native environments, but it’s not insurmountable. With the right strategies, you can embrace AI’s potential while safeguarding your organization.
What steps will you take to address this paradox in your own work? Share your thoughts in the comments, explore our related articles on cloud-native security, or dive deeper into resources like the OWASP AI Security guide.
References
- Palo Alto Networks. (2024). State of Cloud-Native Security Report. Link
- Daily.dev. The Urgent Security Paradox of AI in Cloud-Native Development. Link
- Semgrep. (2025). The Future of SaaS Security: AI-Driven, Fast, and Secure. Link
- Other sources consulted include discussions from Resourcive and Black Hat World for broader insights on AI and security trends.
AI security paradox, AI security, cloud native development, AI-powered code, cloud-native security, security risks, AI innovation, cloud security strategies, DevSecOps, threat intelligence