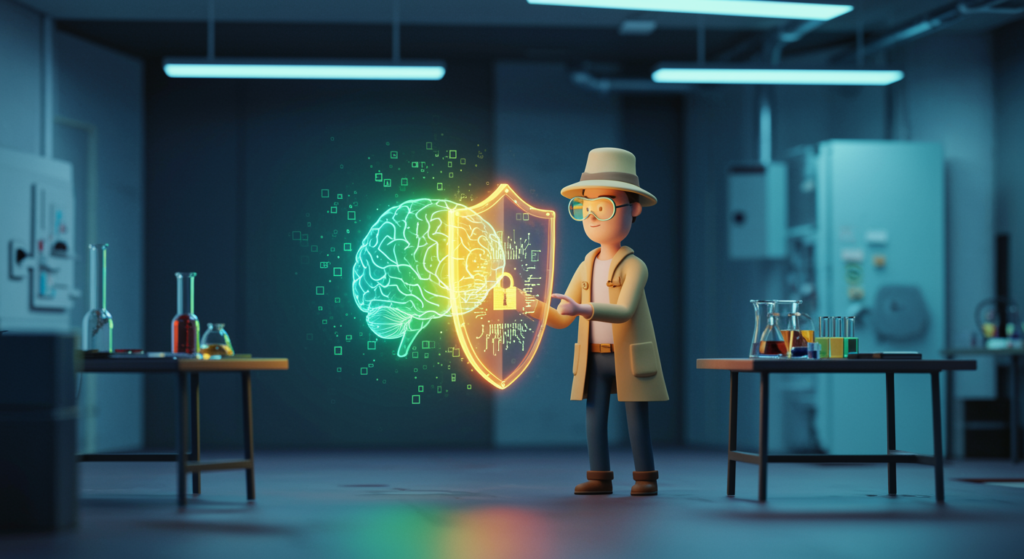
AI Security Specifications Unveiled for Benchmarking AI
A New Era for AI Security Benchmarking
Artificial Intelligence (AI) is reshaping industries at an unprecedented pace, but this progress comes with significant cybersecurity risks that demand specialized attention. The European Telecommunications Standards Institute (ETSI) has stepped up with its new technical specification, ETSI TS 104 223, establishing a global benchmark for AI security benchmarking that covers the full AI lifecycle [1]. This framework helps stakeholders protect AI systems from evolving threats, ensuring that security is built in from the ground up.
Have you ever wondered how AI models, which learn from vast datasets, could be manipulated by bad actors? AI security benchmarking addresses this by providing measurable standards to evaluate and strengthen defenses, making it easier for developers and organizations to innovate responsibly.
Why AI Demands Specialized Security Benchmarking
Traditional cybersecurity tools often fall short when it comes to AI, where threats like data poisoning or model obfuscation can undermine entire systems. AI security benchmarking introduces tailored guidelines to assess and mitigate these risks, ensuring that AI deployments are both effective and secure.
For instance, imagine a healthcare AI system that relies on patient data; if attackers poison the dataset, it could lead to faulty diagnoses. That’s why ETSI TS 104 223 emphasizes proactive measures, drawing from sources like Infosecurity Magazine to highlight vulnerabilities specific to AI [3]. By adopting these benchmarks, businesses can avoid costly breaches and build trust with users.
This approach not only tackles immediate threats but also sets a foundation for long-term resilience, making AI security benchmarking an essential tool in today’s digital landscape.
Overview of the ETSI TS 104 223 Specification
ETSI’s specification, “Securing Artificial Intelligence (SAI); Baseline Cyber Security Requirements for AI Models and Systems,” emerged from collaborative efforts involving global experts and cybersecurity leaders [1]. It focuses on creating a structured benchmark for AI security that spans the entire lifecycle, from initial design to decommissioning.
Key Focus Areas in AI Security Benchmarking
- 13 Core Principles: These form the backbone, outlining essential requirements like data integrity and threat detection to guide AI security benchmarking.
- 72 Trackable Principles: These provide detailed, actionable steps that organizations can measure and implement, turning abstract ideas into practical benchmarks.
- Five AI Lifecycle Phases: Covering secure design, development, deployment, maintenance, and end-of-life processes, this ensures comprehensive protection throughout.
By embedding security-by-design principles, ETSI TS 104 223 helps prevent issues before they arise, much like how architects plan for earthquakes in building designs. If you’re in AI development, consider how these benchmarks could streamline your workflow and reduce vulnerabilities.
Who Stands to Gain from AI Security Benchmarking?
- Developers: They get clear, measurable guidelines to innovate while meeting AI security benchmarking standards.
- Vendors: This allows them to market products with verified security, boosting credibility in a competitive market.
- Integrators: They can deploy AI systems confidently, using benchmarks to ensure seamless and safe integration.
- Operators: Ongoing maintenance becomes easier with tools to monitor and adapt to new threats based on established benchmarks.
What if your organization could use these benchmarks to avoid regulatory fines? It’s a real possibility, as AI security benchmarking promotes compliance and risk reduction.
Key Security Challenges Tackled by AI Security Benchmarking
AI systems are prime targets for sophisticated attacks, and ETSI TS 104 223 directly confronts them through rigorous benchmarking. For example, data poisoning—where attackers corrupt training data—can skew AI outputs, but these standards offer ways to detect and neutralize such risks.
- Data Poisoning: This involves malicious alterations to datasets, which AI security benchmarking addresses with integrity checks and validation techniques.
- Model Obfuscation: Attackers hide flaws in AI models; benchmarks provide tools for transparency and regular audits.
- Indirect Prompt Injection: Subtle manipulations of inputs can lead to unintended results, so benchmarking includes testing protocols to catch these early.
- Complex Data Management: Handling massive datasets securely is crucial, and these guidelines emphasize encryption and access controls.
Think about a financial AI that processes transactions; without proper benchmarking, a prompt injection could cause errors. By following ETSI’s framework, you can implement defenses that make your AI more robust.
Global Impact of AI Security Benchmarking Standards
ETSI TS 104 223 represents a landmark in AI security benchmarking, offering the first global baseline for evaluating and enhancing AI defenses [3]. Scott Cadzow, chair of ETSI’s Technical Committee, notes that this standard brings clarity amid rising cyber threats, helping organizations worldwide align their practices.
Advantages of Embracing AI Security Benchmarks
- Consistent Compliance: It aligns teams with international standards, simplifying audits and regulatory adherence.
- Supply Chain Confidence: Vendors and partners can verify security levels, fostering trust in collaborative projects.
- Risk Mitigation: Proactive benchmarking reduces the likelihood of breaches, saving resources in the long run.
- Innovation Boost: With solid security foundations, developers can focus on creativity without compromising safety.
A hypothetical scenario: A tech startup adopts these benchmarks and avoids a major data breach, turning potential disaster into a success story. How might AI security benchmarking transform your projects?
Comparison: Traditional Cybersecurity vs. AI Security Benchmarking
Aspect | Traditional Cybersecurity | AI Security Benchmarking (ETSI TS 104 223) |
---|---|---|
Focus | Software, hardware, and networks | AI models, data pipelines, and lifecycle management |
Key Threats | Malware, unauthorized access, DDoS | Data poisoning, prompt injection, model theft |
Mitigation Techniques | Firewalls, encryption, access control | Adversarial testing, data integrity checks, benchmark evaluations |
Lifecycle Integration | Primarily development and deployment | Full lifecycle, including design, maintenance, and end-of-life |
This comparison shows how AI security benchmarking goes beyond traditional methods, offering a more holistic approach tailored to AI’s unique needs.
Insights from Recent Studies on AI Security Benchmarking
Beyond ETSI, studies like the RAND report provide additional layers to AI security benchmarking, with five security levels to gauge threats from amateur hackers to state actors [5]. These complement ETSI’s work by offering scalable strategies for organizations.
Breaking Down Security Level Benchmarks
- Basic: Simple protections against novice threats, ideal for small-scale AI deployments.
- Intermediate: Enhanced defenses for experienced attackers, incorporating benchmarking for regular assessments.
- Advanced: Robust measures against persistent threats, with ongoing AI security benchmarking to adapt.
- Network Isolation: Segregates AI systems for added security, a key benchmark for high-risk environments.
- Frontier Threat Protection: Prepares for future risks, using benchmarking to anticipate and test emerging vulnerabilities.
These guidelines aren’t just theoretical; a company could use them to prioritize investments, ensuring their AI security benchmarking aligns with real-world risks.
Practical Applications of AI Benchmarking Frameworks
Frameworks like the CLASSic model expand on AI security benchmarking by evaluating systems on cost, latency, accuracy, stability, and security [7]. This balanced approach helps businesses weigh security against performance, making decisions that drive success.
Essential Metrics in AI Security Benchmarking
- Cost efficiency in operations.
- Latency for real-time responses.
- Accuracy in outputs and processes.
- Stability across varying conditions.
- Security resilience against attacks, a core element of effective benchmarking.
For actionable advice, start by auditing your AI systems using these metrics. It could reveal gaps in security that, once addressed, enhance overall performance.
Securing the Future Through AI Security Benchmarking
With tools like ETSI TS 104 223 and supporting research, AI security benchmarking is empowering organizations to build safer, more reliable systems. As threats evolve, staying ahead means regularly updating your strategies and embracing these standards.
We’d love to hear your thoughts—how is your organization approaching AI security? Share in the comments, explore more on our site, or check out related posts for deeper insights.
References
- ETSI Press Release: “ETSI Technical Specification Sets International Benchmark for Securing Artificial Intelligence.” https://www.etsi.org/newsroom/press-releases/2521-etsi-technical-specification-sets-international-benchmark-for-securing-artificial-intelligence
- SC Magazine: “Benchmarking Standard for Securing AI Systems Released.” https://insight.scmagazineuk.com/benchmarking-standard-for-securing-ai-systems-released
- Infosecurity Magazine: “ETSI Baseline Requirements.” https://www.infosecurity-magazine.com/news/etsi-baseline-requirements/
- Hyperproof: “AI in Cybersecurity 2024 Benchmark Report.” https://hyperproof.io/resource/ai-in-cybersecurity-2024-benchmark-report/
- RAND: “Securing AI Model Weights.” https://www.rand.org/news/press/2024/05/30.html
- Aisera: “Enterprise AI Benchmark.” https://aisera.com/blog/enterprise-ai-benchmark/
AI security benchmarking, AI security, ETSI TS 104 223, AI model protection, AI lifecycle security, global AI benchmarks, AI threat protection, cybersecurity for AI, AI standards, AI risk mitigation