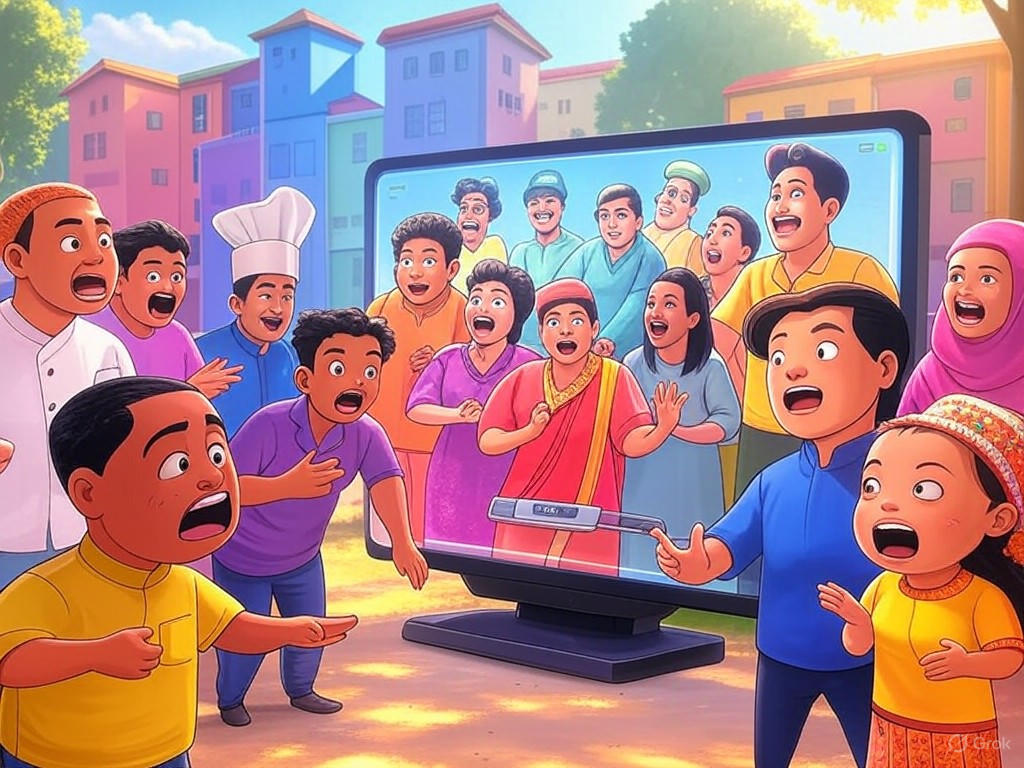
AI Writing Tools Fuel Cultural Stereotyping and Language Homogenization
The Hidden Cost of AI-Generated Content: Cultural Erasure and Stereotyping
Artificial intelligence has transformed content creation, making it faster and more accessible for writers everywhere. But have you ever stopped to think about the unintended consequences? AI cultural stereotyping is creeping into our digital narratives, leading to a loss of cultural richness and the spread of homogenized language that’s often rooted in Western ideals.
As AI tools become staples in our workflows, they risk flattening the unique voices that make storytelling vibrant. Recent studies show how these technologies can amplify biases, making it essential for creators to pause and reflect. By understanding AI cultural stereotyping, we can start using these tools in ways that honor diversity and authenticity.
How AI Systems Perpetuate Cultural Stereotypes
It’s fascinating how AI, designed to help, sometimes hinders. Research from Cornell University highlights that AI writing suggestions often push content toward generic, Western-centric themes, stripping away the depth of other cultures. For instance, when generating descriptions of Diwali, an Indian festival, AI tools might default to oversimplified stereotypes, ignoring the event’s profound traditions and community spirit.
A 2025 study revealed that AI frequently prioritizes holidays like Christmas over diverse celebrations such as Diwali, even in contexts where the latter is more relevant. This pattern of AI cultural stereotyping doesn’t just erase nuances; it reinforces a one-size-fits-all worldview that marginalizes non-Western perspectives. Have you noticed this in your own AI-assisted writing?
Gender and Racial Stereotyping in AI Outputs
AI doesn’t stop at cultural oversights—it dives into gender and racial biases too. A 2024 UNESCO study on Large Language Models like GPT-3.5 and Llama 2 uncovered troubling patterns of gender stereotypes, where men are often depicted as adventurous explorers and women as gentle homemakers.
In AI-generated stories, words linked to men included “treasure,” “woods,” and “adventurous,” while women’s descriptions leaned toward “garden,” “love,” and “husband.” Even more starkly, Llama 2 portrayed women in domestic roles four times more often than men. Imagine the real-world impact: This kind of AI cultural stereotyping could subtly shape how we view gender roles in everyday content.
AI-Generated Content About Men | AI-Generated Content About Women |
---|---|
Treasure, woods, sea, adventurous, decided, found | Garden, love, felt, gentle, hair, husband |
Diverse professional roles | Domestic roles (4x more frequent) |
On the racial front, the same study found AI assigning limited, biased occupations based on ethnicity. British men might appear as “doctors” or “teachers,” but Zulu men are often reduced to “gardeners” or “security guards,” with 20% of portrayals of Zulu women in roles like “domestic servants.” These examples of AI cultural stereotyping highlight how algorithms learn from biased data, perpetuating inequality.
STEM Fields and Professional Representation
AI cultural stereotyping extends to visual and professional depictions as well. The UNDP’s Accelerator Lab discovered that AI image generators like DALL-E overwhelmingly show men in STEM roles, with 75% to 100% of images featuring male figures as engineers or scientists.
OpenAI has admitted that DALL-E reinforces stereotypes, such as depicting “lawyers” as older Caucasian men and “nurses” as women. This not only limits representation but also entrenches societal biases in professional imagery. What if we started challenging these outputs to build a more balanced digital world?
Intersectional Bias in AI Representation
The biases get more complex when gender and race intersect. In one case, a journalist of Asian American descent received AI-generated avatars that were hyper-sexualized and stereotypical, drawing from anime tropes, while her white colleague faced less objectification.
Male colleagues, on the other hand, were shown as “inventors” or “explorers.” This intersection of AI cultural stereotyping creates a doubly harmful narrative for women of color, emphasizing the need for vigilant oversight in AI use.
Homophobic Content and Negative Portrayals
AI’s biases aren’t limited to culture, gender, or race—they can also veer into harmful territory like homophobia. The UNESCO study found that 70% of Llama 2’s responses to prompts about gay individuals were negative, describing them in derogatory social contexts.
GPT-2 similarly generated phrases linking gay people to criminality or marginalization. These outputs don’t just reflect existing prejudices; they risk normalizing them, making AI cultural stereotyping a gateway to broader social harm.
Real-World Consequences of AI Bias
These issues aren’t just theoretical—they play out in everyday scenarios. Take a recent professional demo where an AI generated an image of a Native American woman in a medical setting that echoed outdated, stereotypical tropes from old Western films.
The presenter was mortified in front of colleagues, underscoring how AI cultural stereotyping can lead to public missteps. As content creators rely more on AI for speed, this amplification of bias could flood the web with skewed narratives.
The Compounding Effect on Digital Content
With tools promising to churn out blog posts in seconds, AI cultural stereotyping is spreading rapidly. Features like “Creative” or “Authoritative” tones might vary style, but they rarely fix the core biases embedded in the AI.
Here’s a tip: Always treat AI outputs as raw material, not final drafts, to catch and correct these issues before they go live.
Language Homogenization and Loss of Linguistic Diversity
Beyond stereotypes, AI contributes to language homogenization by favoring familiar, Western patterns over diverse linguistic styles. Writers from non-Western backgrounds often see their voice diluted toward standard English norms when using these tools.
This erosion of cultural expression could mean losing the vibrancy of global languages. How can we preserve that diversity while leveraging AI’s efficiency?
Addressing AI Bias and Preserving Cultural Diversity
Thankfully, there are practical steps to combat AI cultural stereotyping. Start with critical editing—review AI suggestions for biases and infuse them with your unique perspective.
Critical Review and Human Editing
Human input is irreplaceable; treat AI as a collaborator, not a replacement. By editing for cultural accuracy, you ensure content remains authentic and respectful.
Diversifying AI Prompts
Craft prompts that explicitly call for diversity, like “Describe Diwali without stereotypes.” This simple strategy can reduce instances of AI cultural stereotyping right from the start.
Combining AI Tools with Diverse Human Input
Seek feedback from a variety of cultural voices to spot blind spots. It’s like adding layers to a story—richer and more genuine.
Supporting Ethical AI Development
Advocate for change by reporting biased outputs to developers. UNESCO’s ethics recommendations are a great resource for pushing the industry forward—check out their study for more insights.
The Future of AI Content Creation
Looking ahead, balancing AI’s speed with cultural sensitivity will be key. As awareness grows, we can evolve these tools to support, rather than suppress, diversity.
Remember, the power is in your hands—use AI thoughtfully to enhance, not overshadow, human creativity.
Conclusion: Balancing Innovation with Cultural Sensitivity
AI writing tools are incredible for boosting productivity, but their role in AI cultural stereotyping demands we stay vigilant. By adopting strategies like diverse prompts and thorough editing, you can create content that’s both efficient and equitable.
What are your experiences with AI biases? Share your thoughts in the comments, explore more on ethical AI in our related posts, or try these tips in your next project. Let’s work together to make technology a force for good.
References
1. Cornell University. “AI Suggestions Make Writing More Generic, Western.” Link
2. UNESCO. “Generative AI: UNESCO Study Reveals Alarming Evidence of Regressive Gender Stereotypes.” Link
3. ACEHP Almanac. “From Wonder Tool to Harmful Stereotype: The User’s Role in Fighting AI Bias.” Link
4. CIGI. “Generative AI Tools Are Perpetuating Harmful Gender Stereotypes.” Link
5. Ry Rob. “AI Article Writer.” Link
6. Black Hat World. “How to Use AI to Write Blog Posts Without Penalization.” Link
7. Prestige Online. “AI Tools Threat Cultural Diversity.” Link
8. YouTube Video. “Title of Video.” Link
AI cultural stereotyping, AI bias, language homogenization, AI writing tools, generative AI, gender stereotypes, cultural diversity, ethical AI, representation in AI, AI homogenization