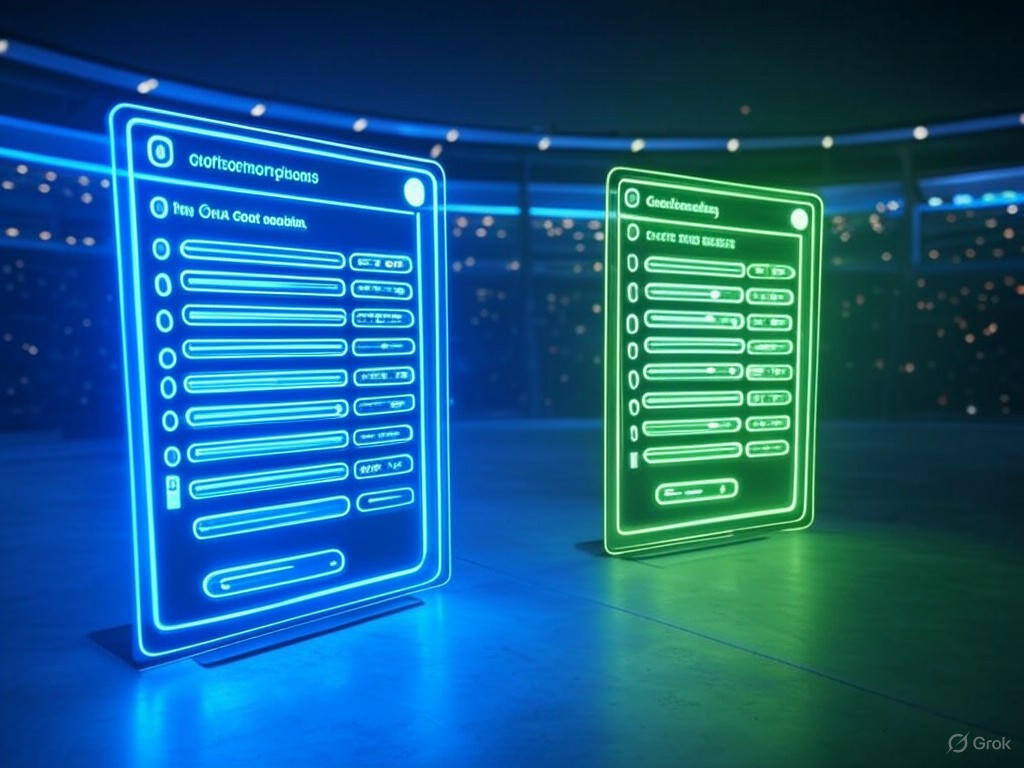
Chatbot Arena Shenanigans Exposed: Investigating the Controversy
Unveiling the AI Benchmarking Controversy
Have you ever wondered if the AI models dominating the headlines are truly the best, or if something sketchier is at play? The AI benchmarking controversy has erupted around Chatbot Arena, a popular platform for testing large language models through user votes. Run by LM Arena in collaboration with UC Berkeley, this system promised fair, crowdsourced comparisons, but recent accusations are making people question its backbone of integrity.
At its core, the AI benchmarking controversy stems from claims that major players like Meta, Google, and OpenAI got an unfair edge. This issue hits hard because benchmarks like these shape how we perceive AI progress, influencing everything from investments to everyday tech adoption.
The Inside Scoop on Chatbot Arena and AI Benchmarking
Picture this: you’re comparing two AI chatbots in a virtual showdown, voting on which one nails a response better. That’s the essence of Chatbot Arena, a tool designed to democratize AI benchmarking by letting everyday users weigh in. But as this AI benchmarking controversy unfolds, we’re seeing how such crowdsourced setups can inadvertently favor the big names.
These battles aren’t just fun—they’re influential. AI companies use Arena results to tout their supremacy, but critics argue the process might be rigged, giving certain firms more visibility and tweaks based on user feedback. It’s a classic case of how AI benchmarking can amplify successes while hiding flaws.
Key Players and Their Stakes in the AI Benchmarking Debate
Who are the main characters in this AI benchmarking controversy? Think tech giants like OpenAI and Google, alongside researchers from places like Stanford and MIT. A group from Cohere and other institutions dropped a bombshell study, pointing fingers at preferential treatment that let these companies polish their models in secret.
For instance, allegations include private testing slots where labs could refine AI without public eyes. This isn’t just nitpicking—it’s about ensuring that AI benchmarking reflects real-world reliability, not just who’s got the inside track.
Deep Dive into Allegations of Manipulation in AI Benchmarking
The drama really heats up with specific charges in the AI benchmarking controversy. According to the study, elite AI labs enjoyed perks like selective score sharing and more frequent matchups, potentially skewing results. Sara Hooker from Cohere called it a “gamification” tactic that’s anything but fair.
Let’s break it down: some companies allegedly tested multiple AI versions privately, only unveiling the winners. This raises a big question—how can we trust AI benchmarking if not everyone’s playing by the same rules? It’s like a race where one runner gets a head start without telling anyone.
Detailed Claims in the AI Benchmarking Controversy
- Private Access Perks: Top labs reportedly got early, exclusive testing, allowing them to iron out kinks before going public in AI benchmarking contests.
- Picking Winners Only: By publishing only their best scores, these firms might have masked weaker performances, muddying the waters of honest AI benchmarking.
- More Spotlight Time: Models from big players showed up in more battles, giving them extra chances to learn and improve through user votes.
These tactics, if true, could mean the AI benchmarking controversy is more than hype—it’s a wake-up call for the entire field. Imagine if your favorite app’s ratings were boosted this way; you’d want answers, right?
Flaws in Crowdsourced AI Benchmarking Systems
Is crowdsourced voting the best way to judge AI? Experts like Emily Bender from the University of Washington think not, highlighting issues with construct validity in AI benchmarking. Simply voting on responses doesn’t always capture what matters most, like ethical implications or real utility.
As Asmelash Teka Hadgu notes, some labs have even tweaked models specifically for Chatbot Arena, only to release inferior versions elsewhere. This kind of gaming underscores why AI benchmarking needs a overhaul to prevent such manipulations.
Think about it: if AI benchmarking relies on quick user polls, how do we account for biases in those votes? It’s a human element that can make or break the credibility of the whole system.
How Industry Giants Are Responding to the AI Benchmarking Controversy
LM Arena has pushed back hard against these claims, insisting their AI benchmarking processes are transparent and open. They’ve argued that any advantages were just from public participation, not secret deals.
Yet, not everyone’s buying it. Some stakeholders are pushing for independent audits to clean up AI benchmarking for good. It’s a mixed bag—while companies like Meta defend their positions, others see this as a chance to rebuild trust.
For example, a TechCrunch article dives deeper into expert critiques, showing how this controversy could lead to better standards across the board.
The Ripple Effects on AI Rankings and Trust
This AI benchmarking controversy isn’t just internal—it’s shaking public confidence in AI as a whole. Leaderboard scores drive hype, funding, and even regulations, so any whiff of bias can send shockwaves.
Compare that to expert-driven methods: they’re less flashy but often more reliable. Here’s a quick look at how they stack up:
Benchmarking Method | Strengths | Weaknesses |
---|---|---|
Crowdsourced Voting |
|
|
Expert Evaluations |
|
|
So, what’s your take—do you prefer the crowd’s voice or expert analysis in AI benchmarking?
Pushing for Fixes in the AI Benchmarking Landscape
In light of this mess, there’s a growing push for reforms to make AI benchmarking more trustworthy. Ideas include setting up independent watchdogs and requiring full disclosure of all model tests.
A hybrid approach could blend user input with expert reviews, creating a more balanced system. If you’re in AI development, consider adopting these strategies to stay ahead and ethical.
For actionable tips, start by auditing your own evaluations: ensure transparency in testing and seek diverse feedback to avoid the pitfalls we’ve seen in this AI benchmarking controversy.
Wrapping Up: Building a Brighter Future for AI Benchmarking
The Chatbot Arena saga highlights the urgent need for honest AI benchmarking practices. As we move forward, collaboration between researchers, companies, and users will be key to restoring faith.
If this topic sparks your interest, why not share your thoughts in the comments below? Explore our other posts on AI ethics, or sign up for updates to stay in the loop. Let’s keep the conversation going—your input could shape the next big change in AI benchmarking.
References
- Simon Willison. “Criticism of the Chatbot Arena.” https://simonwillison.net/2025/Apr/30/criticism-of-the-chatbot-arena/
- OpenTools AI. “LM Arena Under Fire: Allegations of Benchmark Bias Stir AI Industry.” https://opentools.ai/news/lm-arena-under-fire-allegations-of-benchmark-bias-stir-ai-industry
- NextBigWhat. “AI Leaderboard Scandal: Chatbot Testing, Meta, and Google.” https://nextbigwhat.com/ai-leaderboard-scandal-chatbot-testing-meta-and-google-artificial-intelligence-advancements-lm-arena-controversy/
- Bitcoin World. “AI Benchmark Gaming Study.” https://bitcoinworld.co.in/ai-benchmark-gaming-study/
- TechCrunch. “Crowdsourced AI Benchmarks Have Serious Flaws, Some Experts Say.” https://techcrunch.com/2025/04/22/crowdsourced-ai-benchmarks-have-serious-flaws-some-experts-say/
AI benchmarking controversy,Chatbot Arena,AI leaderboard bias,LM Arena controversy,AI evaluation bias,crowdsourced AI flaws,AI model manipulation,tech ethics in AI,AI ranking integrity,fair AI testing