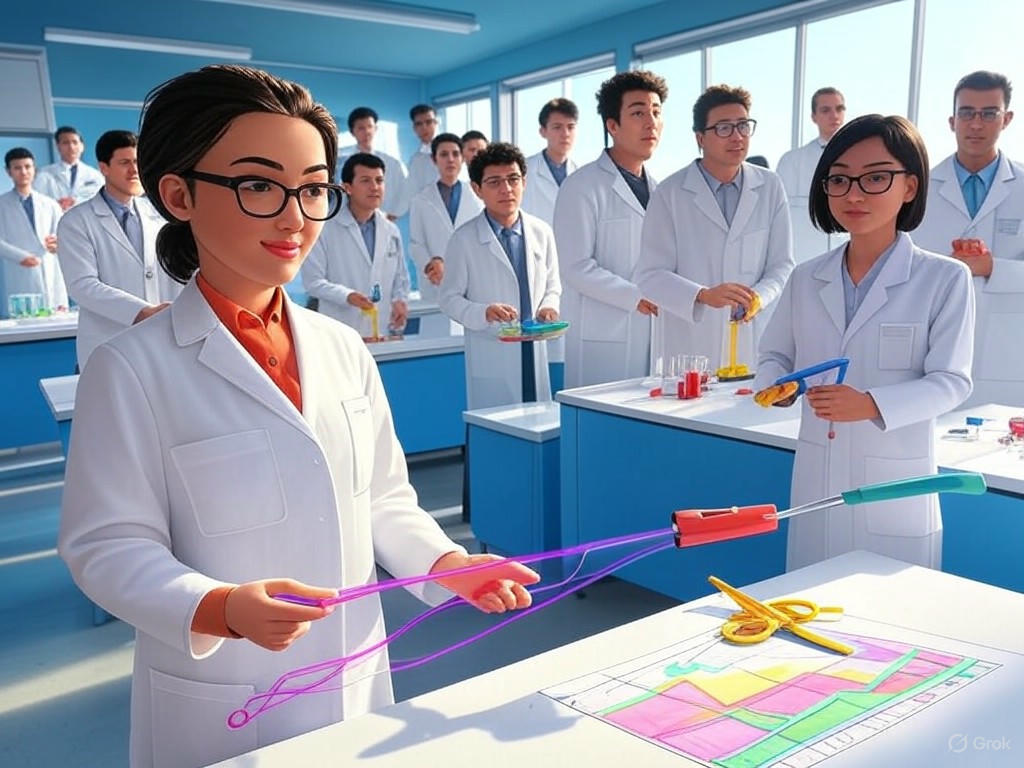
Detecting AI Bias: New Tool Empowers Researchers Globally
Revolutionizing Fairness in AI: Why AI Bias Detection Matters
Artificial intelligence is reshaping industries at an unprecedented rate, yet it’s not immune to the flaws in the data it’s trained on. AI bias detection has emerged as a crucial process, allowing researchers to spot and address unfair prejudices embedded in machine learning models. Imagine a job recommendation system that inadvertently favors certain demographics—tools like these ensure such issues don’t escalate, fostering trust and equitable decisions in hiring, healthcare, and everyday applications.
This focus on AI bias detection isn’t just technical; it’s about building AI that serves everyone fairly. As AI systems influence critical areas like medical diagnoses or news curation, detecting bias early prevents real-world harm and promotes inclusivity. Have you ever wondered how a simple algorithm could perpetuate societal inequalities? Let’s dive into why this matters more than ever.
The Roots of AI Bias: How Unseen Prejudices Shape Machine Learning
Machine learning models, such as popular generative AI tools like ChatGPT, learn from massive internet datasets that often mirror human biases. This means AI bias detection becomes essential to unearth these hidden prejudices before they lead to discriminatory outcomes, like biased language translations or unfair hiring suggestions. For instance, if a dataset underrepresents certain groups, the AI might amplify stereotypes without anyone noticing.
These biases can creep in subtly, affecting everything from facial recognition to content recommendations. According to a study from TechXplore, early AI bias detection can prevent legal and ethical pitfalls. By understanding these roots, researchers can take proactive steps to create more balanced systems.
Introducing LangBiTe: A New Frontier in AI Bias Detection
A game-changer in the field, LangBiTe is an open-source tool developed by experts at the Universitat Oberta de Catalunya and the University of Luxembourg, designed specifically for AI bias detection. What sets it apart is its accessibility, allowing anyone from developers to everyday users to evaluate generative AI for potential discrimination and ensure legal compliance. This tool doesn’t just identify problems; it promotes a culture of transparency and ethical AI development.
With goals like assessing model fairness and encouraging responsible practices, LangBiTe is a beacon for global collaboration. Picture a researcher in a small lab using this free resource to test AI applications—it’s that empowering. By making AI bias detection tools like LangBiTe widely available, we’re moving toward a more accountable tech landscape.
How AI Bias Detection Tools Work: Core Mechanisms Explained
At their heart, AI bias detection tools use sophisticated analytics to scrutinize datasets, predictions, and behaviors for signs of unfairness. These systems often blend natural language processing with statistical methods to flag issues, making AI bias detection a precise science. For example, they can detect if an AI model treats different demographic groups unequally in its outputs.
Techniques like fairness-aware classifiers help in this process, ensuring algorithms don’t favor one group over another. Tools such as Insight7 apply these methods to research contexts, highlighting how AI bias detection can improve data integrity. If you’re working on AI projects, incorporating these tools early can save time and prevent costly errors.
Key Features of Leading AI Bias Detection Platforms
- Automated data scanning: Quickly spots anomalies that signal potential biases, streamlining the AI bias detection process.
- Sentiment and linguistic analysis: Uncovers subtle prejudices in text, helping maintain ethical standards.
- Demographic disparity measurement: Evaluates if models disadvantage specific groups, a core aspect of effective AI bias detection.
- Collaborative reporting: Provides insights that teams can act on immediately.
Case Studies: Real-World Impact of AI Bias Detection
From journalism to healthcare, AI bias detection is making a tangible difference. In media, the University of Pennsylvania’s Media Bias Detector helps journalists analyze their reporting for unintended biases, ensuring balanced news coverage. This not only builds reader trust but also demonstrates how AI bias detection can combat misinformation.
In healthcare, adaptive frameworks identify disparities in AI-driven diagnostics, leading to fairer treatments across racial and socioeconomic lines. Consider a scenario where an AI tool unfairly predicts outcomes for certain patients—AI bias detection tools correct this, potentially saving lives. These examples show why prioritizing AI bias detection is non-negotiable for ethical AI deployment.
Corporate settings benefit too, with tools monitoring AI for compliance and fairness. What if your company used these strategies to avoid lawsuits? It’s a practical step toward responsible innovation.
Comparison Table: Top Approaches to AI Bias Detection
Tool/Approach | Core Method | Best For | Unique Strength |
---|---|---|---|
LangBiTe (Open Source) | Multi-model assessment, legal compliance checks | Academic, non-technical users | Transparency and open access for robust AI bias detection |
Insight7 | NLP, sentiment analysis | Research bias detection | In-depth analysis of methodologies |
Responsible AI Platforms | Integrated fairness checks | Corporations, MLOps teams | Lifecycle-wide monitoring, enhancing AI bias detection |
Media Bias Detector | Event and tone analysis | Newsrooms, media analysts | Real-time analysis for effective AI bias detection |
Adaptive Bias Detection Frameworks (Healthcare) | Statistical algorithms, classifiers | Healthcare AI | Focus on equity and debiasing techniques |
Beyond Detection: Strategies for AI Bias Mitigation
Once AI bias detection uncovers issues, the real work begins with mitigation techniques like re-weighting data or adversarial debiasing. These methods ensure models produce equitable results, turning detection into actionable insights. For organizations, integrating these strategies means building AI that stands up to scrutiny.
Fairness constraint optimization is another powerful tool, enforcing balanced outcomes across applications. A quick tip: Start with continuous monitoring post-deployment to catch any emerging biases early. By combining AI bias detection with these steps, you’re not just fixing problems—you’re preventing them.
Empowering Researchers: The Global Reach of AI Bias Detection Tools
Open-source tools are democratizing AI bias detection, giving researchers around the world the tools they need, regardless of their resources. This accessibility fosters collaboration and innovation, making fairness a global priority. Ever thought about how a simple tool could change the way we approach AI ethics? That’s the power at play here.
When choosing the right AI bias detection solution, consider factors like data type support and ease of integration. This ensures it fits seamlessly into your workflow, boosting efficiency and ethical standards.
Tips for Selecting Effective AI Bias Detection Tools
- Evaluate support for various data types to cover all aspects of AI bias detection.
- Prioritize user-friendly options for non-experts.
- Ensure scalability for long-term use.
The Future of AI: Advancing Fairness Through Bias Detection
As technology evolves, so does the landscape of AI bias detection, with new advancements promising even greater accuracy and usability. This progress is key to creating a digital world where AI serves all communities equitably. Researchers and developers are at the forefront, driving these changes forward.
Looking ahead, expect tools that integrate seamlessly into everyday workflows, making AI bias detection second nature. How might this shape your own projects? It’s an exciting time for ethical innovation.
Wrapping Up: A Call for Fair AI Practices
In summary, AI bias detection tools like LangBiTe are making it easier than ever to build trustworthy AI. By adopting these resources, we can ensure technology benefits everyone fairly. What’s your take on tackling AI biases—share your thoughts in the comments, explore our related posts on algorithmic fairness, or try out an open-source tool today to see the difference.
References
- TechXplore. (2024). AI bias detection tool to tackle discrimination. https://techxplore.com/news/2024-12-ai-bias-tool-tackle-discrimination.html
- DARPA. (n.d.). MAD Bias Program. https://www.darpa.mil/research/programs/mad-bias
- AIMultiple. (2023). AI Bias Research. https://research.aimultiple.com/ai-bias/
- Envisioning. (2022). Algorithmic Bias Detection Tool Signals. https://www.envisioning.io/signals/algorithmic-bias-detection-tool
- University of Pennsylvania ASC. (2024). AI-Powered Bias Detector Transforms News Analysis. https://www.asc.upenn.edu/ai-powered-bias-detector-transforms-news-analysis
- Insight7. (2023). AI Tools for Research Bias Detection. https://insight7.io/ai-tools-for-research-bias-detection/
- Ignite Visibility. (2024). AI Generated Content. https://ignitevisibility.com/ai-generated-content/
- Gavin Publishers. (2023). AI for Equity: Developing Adaptive Bias Detection Frameworks for Healthcare Algorithms. https://www.gavinpublishers.com/article/view/ai-for-equity-developing-adaptive-bias-detection-frameworks-for-healthcare-algorithms-
AI bias detection, algorithmic fairness, bias mitigation, research tools, generative AI ethics, AI tools, fairness in AI, ethical AI development, open-source AI, global AI research