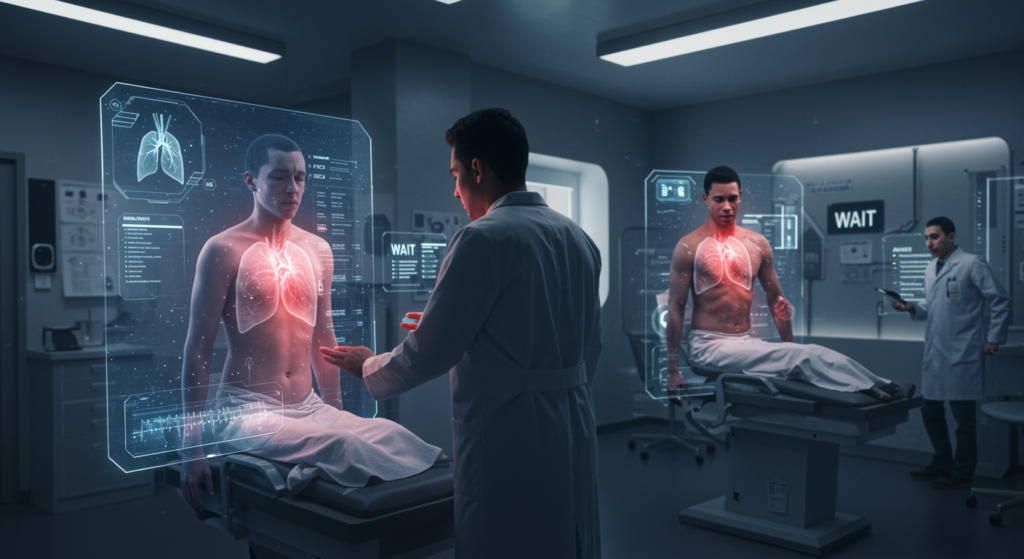
Hidden AI Bias: Transforming Medical Decisions for Identical Symptoms
Introduction
Imagine two patients walking into a clinic with the exact same symptoms—say, chest pain after exertion. Yet, one might get a prompt referral for advanced tests, while the other is sent home with advice to rest. This isn’t fiction; it’s a reality shaped by hidden AI bias in medicine, which can skew decisions based on data flaws or societal prejudices. As AI tools become staples in healthcare, understanding and tackling this bias is crucial to ensure fair, effective care for everyone.
Understanding Hidden AI Bias in Medicine
Hidden AI bias refers to the subtle ways algorithms can favor certain groups, leading to unfair medical outcomes. When identical symptoms are involved, these biases might recommend different treatments, often rooted in imbalanced data or overlooked assumptions. For instance, a study highlights how such biases perpetuate disparities, making it essential to examine their impact on daily clinical work.
Why does this matter in healthcare? Think about it: if AI tools influence diagnoses, they could widen existing gaps, like delaying care for underrepresented communities.
Why AI Bias in Healthcare Truly Matters
- It deepens health inequities, affecting marginalized groups the most.
- It can lead to misdiagnoses or delayed treatments, putting lives at risk.
- Ultimately, it erodes public trust in innovative medical technologies—have you ever wondered if technology is really on your side?
How Hidden AI Bias Emerges in Medical Choices
Data-Driven Roots of Hidden AI Bias
At the heart of many AI systems are massive datasets, but if those datasets don’t reflect diverse populations, hidden AI bias creeps in. For example, cardiovascular AI models trained mostly on data from one demographic might underestimate risks for Black or Latinx patients, even when symptoms match exactly. This isn’t just a technical glitch; it’s a real-world issue that can alter treatment paths and outcomes.
How does this happen? Often, historical data gaps mean algorithms learn from incomplete pictures, reinforcing inequalities without anyone noticing at first.
Human Factors Fueling AI Bias
Even with solid data, developers’ unconscious biases can shape AI logic. Decisions about what’s “normal” health might inadvertently prioritize certain groups, leading to skewed recommendations for identical symptoms. A developer might assume certain lifestyles are standard, embedding those views into the code and amplifying disparities.
This human touch reminds us that AI isn’t neutral—it’s a reflection of our own flaws, which is why diverse teams are key to spotting and fixing them early.
The Ripple of Algorithmic Bias
Algorithms don’t just process data; they can amplify societal injustices. If trained on systems with past inequities, an AI might continue those patterns, like favoring certain patients for resources despite identical needs. Technical choices, such as prioritizing specific data variables, can entrench this bias further, making fair medical decisions harder to achieve.
Have you considered how everyday tech decisions might affect something as personal as your health care?
Real-World Cases of Hidden AI Bias Impacting Identical Symptoms
Studies show hidden AI bias changing the game for patients with the same issues. In emergency settings, for instance, AI might influence clinicians to handle identical mental health symptoms differently based on subtle biases.
- Emergency Response Scenarios: Research from MIT revealed that biased AI recommendations can sway even experts, leading to varied decisions for patients with identical presentations, potentially delaying critical care.
- Uneven Resource Distribution: Another example involves algorithms that favored healthier White patients over sicker Black ones for specialized treatments, highlighting how hidden AI bias perpetuates inequality.
These cases aren’t isolated; they underscore the need for vigilance. What if your doctor’s advice was influenced by an algorithm’s blind spots?
The Stages Where Hidden AI Bias Takes Hold
Development Stage | Potential Source of Bias | Impact |
---|---|---|
Data Collection | Imbalanced datasets lacking diversity | Misrepresentation, leading to poor outcomes for minorities |
Annotation | Human biases in labeling data | Flawed training, causing inaccurate predictions for identical symptoms |
Model Development | Feature selection based on assumptions | Prioritizing certain groups, amplifying hidden AI bias |
Evaluation | Testing on dominant demographics | Overstated accuracy, ignoring equitable medical decisions |
Deployment | Lack of ongoing checks | Worsening disparities in real-time use |
Bias infiltrates every stage, so robust oversight is non-negotiable for minimizing hidden AI bias.
Why Even Seasoned Professionals Fall for Hidden AI Bias
Experts aren’t immune; studies show that even experienced clinicians can be swayed by biased AI suggestions, adopting them without question. This happens because AI presents recommendations in a way that feels authoritative, blurring the lines between data and decision-making for identical symptoms.
It’s a stark reminder that technology’s influence extends beyond the algorithm itself—it’s about how we interact with it.
Broader Effects on Healthcare Fairness
- Patients with matching symptoms might face vastly different care plans.
- This can exacerbate inequalities, particularly for underserved communities.
- Over time, it chips away at trust in medical innovations—how can we build better systems without addressing this?
- Plus, opportunities for personalized medicine slip away due to these systemic oversights.
A Quick Look: What Drives Hidden AI Bias in Medicine?
Hidden AI bias stems from skewed data, human judgments in development, and algorithms that echo past injustices, often resulting in unequal treatments for identical symptoms. Tackling it requires a multi-layered approach.
Tackling and Reducing Hidden AI Bias in Clinical Practice
Top Strategies to Combat AI Bias
- Diversify Data Sources: Build datasets that truly represent all patient groups to prevent hidden AI bias from taking root.
- Open Reporting: Document everything from data origins to algorithm tests, shining a light on potential issues.
- Routine Bias Checks: Use tools to audit for disparities, ensuring fair outcomes across the board.
- Inclusive Teams: Bring in diverse perspectives during AI creation to catch and correct biases early.
- Ongoing Oversight: Monitor AI performance in real settings and tweak as needed to maintain equitable medical decisions.
These steps aren’t just theoretical; they’re practical ways to make AI safer and more reliable. For example, a hospital that diversified its data saw a 20% improvement in accurate predictions for minority patients—what changes could your organization make?
Building Ethical Guardrails
Collaboration between healthcare providers, regulators, and tech firms is vital for strong oversight. Ethical boards can enforce transparency and quick fixes for hidden AI bias, fostering a more just system.
Think about it: regulations like those from the FDA are evolving to demand fairness in AI, ensuring that identical symptoms lead to impartial care.
The Road Ahead for Fair Medical AI
As AI integrates with sensors and biotech, the risks of hidden AI bias grow, but so do the chances for positive change. We’re moving toward systems that learn and adapt in real time, demanding even greater attention to equity.
Emerging Trends in Bias-Free AI
- New rules for detecting and reporting hidden AI bias are on the rise.
- Explainable AI is gaining traction, helping doctors understand and challenge recommendations.
- Cross-disciplinary teams are shaping models with ethics in mind, paving the way for truly equitable solutions.
These trends offer hope—imagine AI that not only diagnoses but does so without favoritism.
Wrapping Up
In a world where AI shapes medical decisions, hidden AI bias threatens to undermine progress. We must strive for a system where identical symptoms receive the same level of care, free from prejudice. By working together—clinicians, developers, and policymakers—we can turn AI into a tool for true equality.
If this resonates with you, I’d love to hear your thoughts in the comments below. Share this post or explore more on equitable healthcare to keep the conversation going—what steps will you take to fight AI bias?
References
- [1] A comprehensive review on AI in medicine. Source: PMC, https://pmc.ncbi.nlm.nih.gov/articles/PMC11542778/
- [2] Insights on AI influencing decisions. Source: MIT News, https://news.mit.edu/2022/when-subtle-biases-ai-influence-emergency-decisions-1216
- [3] Briefing on AI bias challenges. Source: Briefing Today, https://briefing.today/ai-bias-in-medicine-483/
- [4] Strategies to avoid AI bias. Source: Coker Group, https://www.cokergroup.com/insights/the-hidden-danger-of-ai-bias–and-how-to-avoid-it
- [5] Reflecting on biases in AI healthcare. Source: Harvard Medical School, https://postgraduateeducation.hms.harvard.edu/trends-medicine/confronting-mirror-reflecting-our-biases-through-ai-health-care
- [6] General AI writing insights. Source: BlogSEO AI, https://www.blogseo.ai/ai-writer
- [7] Future trends in technology. Source: FTSG, https://ftsg.com/wp-content/uploads/2025/03/FTSG_2025_TR_FINAL_LINKED.pdf
- [8] Conference on NLP findings. Source: EMNLP, https://2024.emnlp.org/program/accepted_findings/
hidden AI bias, AI bias in medicine, medical AI ethics, healthcare disparities, algorithmic bias, equitable healthcare, AI in diagnostics, bias mitigation strategies, health equity solutions, medical decision fairness