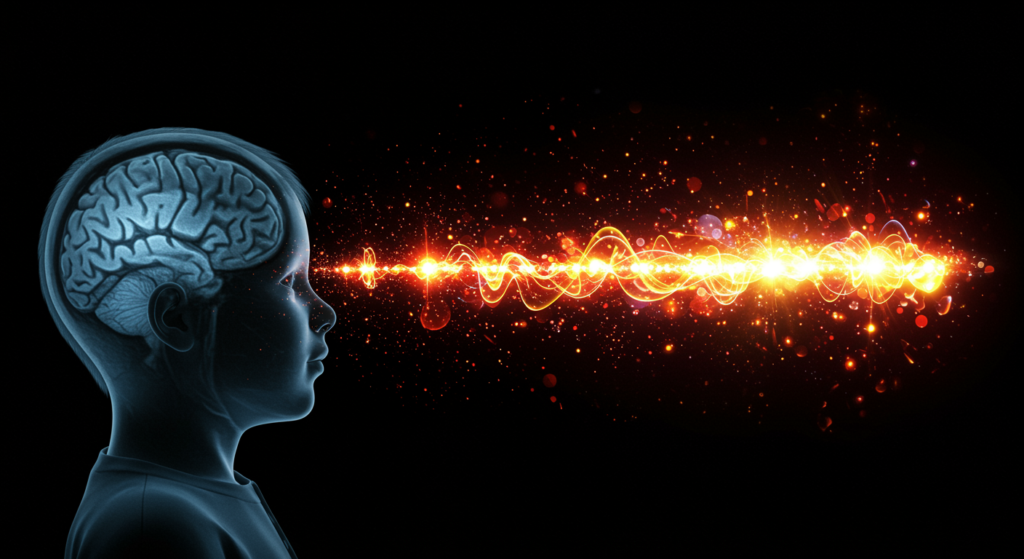
Predicting Pediatric Glioma Recurrence Using Temporal Learning AI
Breaking New Ground in Pediatric Glioma Recurrence Prediction
Imagine a world where predicting pediatric glioma recurrence could spare children from endless rounds of stressful MRI scans. That’s exactly what a team of researchers from Mass General Brigham, Boston Children’s Hospital, and Dana-Farber/Boston Children’s Cancer and Blood Disorders Center has achieved. Their innovative AI model, published in The New England Journal of Medicine AI, harnesses temporal learning to analyze sequences of brain MRI scans, spotting subtle signs of glioma recurrence that might otherwise go unnoticed[1].
Pediatric glioma recurrence is a major worry for families, as these tumors are among the most common in children. While surgery often cures low-grade gliomas, the fear of relapse leads to routine imaging that can feel overwhelming. This new approach offers hope by providing more accurate predictions without relying on imprecise markers[3].
Have you ever wondered how AI could make a real difference in cancer care? This model does just that, using patterns from multiple scans to forecast outcomes, potentially transforming how we handle pediatric glioma recurrence.
The Burden of Uncertainty in Managing Pediatric Glioma Recurrence
Senior author Dr. Benjamin Kann from the Artificial Intelligence in Medicine program at Mass General Brigham puts it plainly: “Many pediatric gliomas are curable with surgery alone, but when relapses occur, they can be devastating.” The challenge with pediatric glioma recurrence lies in the lack of reliable predictors, forcing doctors to schedule frequent MRIs for years. This not only adds stress for kids and parents but also raises questions about long-term effects[1].
For families, the emotional toll is immense—think of the anxiety before each scan or the disruption to daily life. In up to half of cases, recurrence can lead to serious complications, making early detection crucial yet hard to achieve. What if we could ease this burden while keeping kids safe?
This uncertainty highlights why advancing pediatric glioma recurrence prediction is so vital, as it could lead to tailored care plans that minimize unnecessary procedures.
How Temporal Learning AI is Revolutionizing Pediatric Glioma Recurrence Prediction
Temporal learning AI stands out because it doesn’t just look at one MRI scan; it examines a series over time. This method uncovers hidden patterns in brain images that signal potential glioma recurrence, offering a more dynamic view than traditional techniques[4].
The Technical Approach Behind Temporal Learning for Glioma Recurrence
Led by Dr. Benjamin Kann and first author Divyanshu Tak, the team trained their AI model on chronologically ordered MRI scans. By focusing on changes in tissue and the tumor environment, the system learns to link these shifts to actual recurrence events. It’s like turning a static photo album into a predictive movie[7].
This differs from older methods that analyze images in isolation. Instead, temporal learning builds a timeline, helping predict pediatric glioma recurrence with greater precision. For instance, in a hypothetical scenario, if a child’s scans show gradual changes post-surgery, the AI could flag rising risks early, allowing for proactive steps.
The beauty of this for pediatric glioma recurrence prediction is its ability to catch trends that human eyes might miss, potentially saving lives through timely interventions.
Impressive Accuracy in Predicting Pediatric Glioma Recurrence
The results are eye-opening: this AI model boosts recurrence prediction accuracy by nearly 60% over standard methods[3]. When tested on sequential scans, it achieved up to 89% accuracy, proving that tracking changes over time is key[4].
Even better, the model only needs 4-6 scans to perform at its best, which could mean fewer visits for families. This advancement in pediatric glioma recurrence prediction might help doctors optimize monitoring without cutting corners on safety.
So, how does this change things? It could mean personalized plans where high-risk kids get more attention, and others enjoy a break from constant checks.
Potential Clinical Impact on Pediatric Glioma Recurrence Management
Beyond predictions, this AI tool paves the way for personalized care in pediatric glioma recurrence. It promises benefits like reduced imaging for low-risk patients and earlier interventions for those at higher risk[1].
Benefit | Impact on Pediatric Glioma Recurrence |
---|---|
Reduced Imaging Burden | Fewer scans for stable patients, easing family stress |
Earlier Intervention | Quick action for at-risk cases, improving survival rates |
Personalized Follow-Up | Custom schedules based on AI insights |
Improved Resource Allocation | Better use of hospital resources for all patients |
Enhanced Risk Stratification for Pediatric Glioma Recurrence
One standout feature is how this AI improves risk stratification, helping doctors pinpoint who needs frequent scans and who doesn’t. For pediatric glioma recurrence, this means less guesswork and more targeted care[3].
Dr. Kann and his team emphasize that tools like this are essential for guiding decisions. In practice, a parent might ask, “Can we skip this scan?” and the AI could provide data-driven answers, making follow-up more manageable.
This approach to pediatric glioma recurrence prediction could ultimately lead to better outcomes by focusing efforts where they’re needed most.
The Collaborative Effort Driving Pediatric Glioma Recurrence Innovations
This breakthrough stemmed from a partnership between top institutions, funded by the National Institutes of Health. By sharing data from hundreds of patients, they created a robust model for predicting pediatric glioma recurrence[3].
Such collaborations show how teamwork accelerates progress. For example, pooling expertise from different centers allowed for a more comprehensive dataset, which is crucial for refining AI in oncology.
If you’re interested in the details, check out the original research from Mass General Brigham, which highlights the potential of these joint efforts.
Future Directions in AI for Pediatric Glioma Recurrence and Beyond
While this model targets pediatric glioma recurrence, its temporal learning technique could apply to other cancers or conditions. It’s about turning imaging into a story of health over time[7].
Implementation Challenges in Pediatric Glioma Recurrence Prediction
Bringing this to clinics isn’t straightforward—it involves testing across diverse groups, integrating with existing systems, and training staff. Ensuring the AI’s reliability for pediatric glioma recurrence will require ongoing tweaks[4].
Questions around regulations and validation are also key, as we don’t want to rush something this important. Still, it’s an exciting step forward.
The Path to Everyday Use for Pediatric Glioma Recurrence
There’s no set timeline yet, but publication in a top journal is a big win. Future studies will fine-tune this for real-world application, potentially changing pediatric care for the better[1].
What do you think—could AI like this transform how we handle childhood cancers?
A New Era in Managing Pediatric Glioma Recurrence
This AI model represents a milestone, offering a smarter way to monitor and predict pediatric glioma recurrence. By analyzing trends in scans, it could reduce anxiety for families and empower doctors with better tools[7].
For children facing brain tumors, this means more normalcy in their lives. As a next step, consider discussing AI’s role in cancer care with your healthcare provider—it’s a conversation worth having.
We’d love to hear your thoughts on this technology. Share your experiences in the comments, explore more on our site, or spread the word to support advancements in pediatric health.
References
- [1] Mass General Brigham. “Artificial Intelligence Predicts Pediatric Brain Cancer Relapse.” Link
- [3] Inside Precision Medicine. “Pediatric Glioma Recurrence Predicted by Temporal Learning AI Model.” Link
- [4] Applied Radiation Oncology. “New AI Model Forecasts Pediatric Cancer Recurrence.” Link
- [5] Health Imaging. “AI Predicts Pediatric Cancer Recurrence With Impressive Accuracy.” Link
- [7] Bioengineer. “AI Tool Enhances Prediction of Relapse in Pediatric Brain Cancer.” Link
pediatric glioma recurrence, temporal learning AI, brain tumor recurrence, pediatric brain cancer, AI in oncology, MRI prediction, childhood glioma, cancer relapse prediction, glioma AI model, pediatric oncology advancements