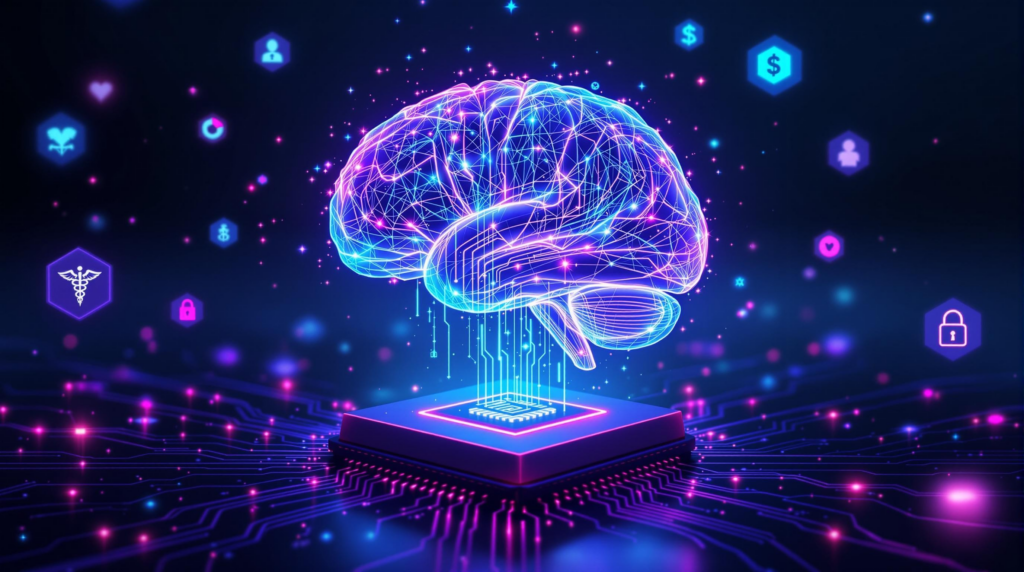
Quantum Artificial Intelligence: Revolutionizing Computing and Problem-Solving in 2025
Have you ever wondered what happens when two of the most groundbreaking technologies of our time—quantum computing and artificial intelligence—converge? The result is Quantum Artificial Intelligence, an emerging field with the potential to solve problems that even our most powerful classical computers find impossible. This revolutionary technology is poised to transform industries from healthcare to finance, offering unprecedented computational power and problem-solving capabilities.
As we navigate through 2025, Quantum AI is moving from theoretical research to practical applications, opening new frontiers in drug discovery, financial modeling, materials science, and more. Let’s explore this fascinating intersection of quantum physics and machine learning that promises to reshape our technological landscape.
What is Quantum Artificial Intelligence?
Quantum Artificial Intelligence (Quantum AI) combines quantum computing with artificial intelligence to create systems capable of processing and analyzing information in ways that classical computers cannot. But what exactly does this mean, and why is it so revolutionary?
Definition and Core Concepts
At its core, Quantum AI refers to the use of quantum computing for the computation of machine learning algorithms. It leverages the principles of quantum mechanics to enhance AI capabilities, potentially offering exponential speedups for certain computational tasks. According to research by AIMultiple, this convergence creates new possibilities for solving complex problems that would be intractable for traditional computing methods.
Unlike traditional computing that uses bits (0s and 1s), quantum computing uses quantum bits or “qubits.” These qubits can exist in multiple states simultaneously thanks to the quantum properties of superposition and entanglement, allowing quantum computers to process vast amounts of information in parallel. As NetApp explains, this fundamental difference gives quantum computers their unique problem-solving capabilities.
The Intersection of Quantum Computing and AI
The marriage of quantum computing and AI creates a powerful synergy. While AI thrives on processing massive datasets to identify patterns and make predictions, quantum computing offers the computational horsepower to handle complex calculations that would overwhelm classical systems.
This fusion enables researchers to develop quantum versions of classical machine learning algorithms, such as quantum neural networks, quantum support vector machines, and quantum reinforcement learning. Wikipedia’s overview of quantum machine learning highlights how these quantum-enhanced algorithms can potentially solve problems that are currently intractable for classical AI systems.
How Quantum AI Differs from Classical AI
The fundamental difference between quantum and classical AI lies in their computational approach. Classical AI processes information sequentially using binary bits, while Quantum AI can explore multiple solutions simultaneously through quantum parallelism. A comprehensive comparison by IndustryWired examines how this distinction could determine which approach dominates in the future.
This distinction gives Quantum AI several advantages:
- Exponentially faster processing for specific problems
- Ability to handle more complex datasets and models
- Enhanced optimization capabilities for finding global solutions
- Improved pattern recognition in high-dimensional data
However, Quantum AI isn’t universally superior. It excels at specific tasks like optimization problems and quantum simulations, while classical AI remains more practical for many everyday applications due to its maturity and accessibility. As experts noted in IoT World Today, both approaches will likely coexist and complement each other for the foreseeable future.
The Quantum Principles Powering Quantum AI
To understand how Quantum AI works, we need to grasp the fundamental quantum principles that give it its unique capabilities. Three key quantum phenomena form the foundation of quantum computing’s power: superposition, entanglement, and quantum interference.
Superposition: Computing Multiple Possibilities Simultaneously
Superposition is perhaps the most well-known quantum principle, allowing qubits to exist in multiple states at once. While a classical bit must be either 0 or 1, a qubit can be both simultaneously, existing in a probabilistic combination of states.
This property enables quantum computers to process multiple possibilities in parallel, creating a form of quantum parallelism that can exponentially increase computational power for certain problems. Quantum Inspire’s knowledge base explains how, for machine learning applications, this means algorithms can evaluate numerous potential solutions simultaneously rather than sequentially.
Entanglement: The Quantum Connection
Quantum entanglement creates a profound connection between qubits, where the state of one qubit is directly related to the state of another, regardless of the distance between them. Einstein famously referred to this as “spooky action at a distance.”
In Quantum AI, entanglement allows information to be shared instantaneously between qubits, enabling more efficient information processing and creating correlations that classical systems cannot achieve. Cortico-X’s guide to quantum computing details how this property is particularly valuable for complex pattern recognition tasks and data analysis.
Quantum Interference: Enhancing Correct Solutions
Quantum interference is the third pillar of quantum computing’s power. Similar to how waves can amplify or cancel each other out, quantum interference allows quantum algorithms to enhance the probability of finding correct solutions while suppressing incorrect ones.
This property is crucial for quantum algorithms like Grover’s search algorithm and quantum phase estimation, which form the basis of many quantum machine learning approaches. IBM’s quantum computing overview explains how, by leveraging interference, Quantum AI can potentially find optimal solutions more efficiently than classical methods.
Types of Quantum Machine Learning Algorithms
Quantum machine learning encompasses various algorithmic approaches, each leveraging quantum properties to enhance different aspects of AI. Let’s explore the most promising types of quantum machine learning algorithms being developed and implemented today.
Variational Quantum Algorithms (VQAs)
Variational Quantum Algorithms represent one of the most practical approaches to Quantum AI in the current era of noisy intermediate-scale quantum (NISQ) devices. VQAs use a hybrid quantum-classical approach, where a quantum processor prepares quantum states, measurements are made, and optimization is performed by a classical computer.
These algorithms are particularly valuable because they’re relatively noise-tolerant compared to other quantum algorithms, making them suitable for today’s imperfect quantum hardware. A comprehensive review published on arXiv details how popular examples include the Quantum Approximate Optimization Algorithm (QAOA) and the Variational Quantum Eigensolver (VQE), which are being applied to optimization problems and quantum chemistry simulations.
Quantum Neural Networks
Quantum neural networks (QNNs) are quantum analogues of classical neural networks, designed to process and learn from quantum data. They can be implemented using quantum circuits that mimic the structure and function of neural networks.
QNNs offer several potential advantages over classical neural networks, including:
- Exponentially larger parameter space for model representation
- Ability to process quantum data directly
- Enhanced capacity for certain learning tasks
- Potential for quantum speedup in training and inference
A groundbreaking study published in Nature Computational Science demonstrated that QNNs can achieve higher capacity and faster training for specific tasks compared to their classical counterparts.
Quantum Support Vector Machines
Quantum Support Vector Machines (QSVMs) are quantum versions of the popular classical machine learning algorithm used for classification tasks. QSVMs leverage quantum computing to perform calculations in high-dimensional feature spaces more efficiently.
By encoding data into quantum states and utilizing quantum operations, QSVMs can potentially handle larger datasets and more complex classification boundaries than classical SVMs. Wikipedia’s section on quantum binary classifiers notes that experimental implementations have already shown promising results for tasks like handwritten digit recognition.
Quantum Reinforcement Learning
Quantum Reinforcement Learning combines quantum computing with reinforcement learning, where an agent learns to make decisions by interacting with an environment. In the quantum version, either the agent, the environment, or both can leverage quantum properties.
This approach has shown potential for quadratic speedups in learning time and improved decision-making capabilities. Research cited by Wikipedia confirms that experimental demonstrations using trapped ions and photonic systems have already verified some of these theoretical advantages.
Real-World Applications of Quantum AI in 2025
While Quantum AI is still evolving, several promising real-world applications are beginning to emerge in 2025. These applications span multiple industries and demonstrate the transformative potential of this technology.
Drug Discovery and Healthcare Advancements
One of the most exciting applications of Quantum AI is in drug discovery and development. By simulating molecular interactions at the quantum level, Quantum AI can significantly accelerate the identification of potential drug candidates and predict their effectiveness.
Pharmaceutical companies like Pfizer are already collaborating with quantum computing providers to leverage this technology. According to Pfizer’s own research, Quantum AI enables researchers to model complex protein folding, simulate drug-target interactions, and predict drug efficacy with unprecedented accuracy.
Additionally, Quantum AI is being applied to genomics research, medical imaging analysis, and personalized medicine, potentially revolutionizing healthcare delivery and patient outcomes. A recent study published in the National Library of Medicine explores these applications in detail, highlighting their potential to transform medical research and treatment.
Financial Modeling and Optimization
The financial sector is another area where Quantum AI is making significant inroads. Financial institutions are exploring quantum-enhanced algorithms for portfolio optimization, risk assessment, fraud detection, and algorithmic trading.
Quantum AI excels at solving complex optimization problems that are common in finance, such as finding the optimal asset allocation across thousands of potential investments while balancing multiple constraints. SandboxAQ’s analysis suggests this capability could lead to more efficient markets, better risk management, and improved investment returns.
Major financial institutions like JP Morgan and Goldman Sachs have already established quantum computing research teams to explore these applications. SpinQuanta reports that these early adopters are positioning themselves to gain competitive advantages as the technology matures.
Materials Science and Chemistry
Quantum AI is particularly well-suited for materials science and chemistry applications because these fields inherently involve quantum mechanical processes. By accurately simulating quantum systems, researchers can discover new materials with specific properties without extensive laboratory testing.
Applications include:
- Designing more efficient batteries and energy storage solutions
- Developing new catalysts for industrial processes
- Creating novel superconducting materials
- Optimizing solar cell efficiency
Google’s quantum research team has already demonstrated promising results in this area, as detailed in their blog post on real-world quantum applications. These advancements could accelerate the transition to renewable energy and enable more sustainable industrial processes.
Supply Chain and Logistics Optimization
Supply chain and logistics optimization presents another promising application area for Quantum AI. The complex task of optimizing routes, schedules, and resource allocation across global supply chains is well-suited to quantum algorithms.
Companies like DHL are exploring quantum route planning to optimize delivery paths, potentially reducing fuel consumption, costs, and environmental impact. SpinQuanta’s industry analysis reveals that manufacturers are also investigating quantum-enhanced optimization for production scheduling and inventory management.
These applications could lead to more resilient supply chains, reduced waste, and improved operational efficiency across industries.
Current State of Quantum AI Development
As we assess the landscape of Quantum AI in 2025, it’s important to understand the current state of development, including key players, available hardware, and research trends.
Major Players and Recent Breakthroughs
Several technology giants and specialized startups are leading the charge in Quantum AI development:
- Google Quantum AI: Google has made significant strides in quantum computing, including achieving quantum supremacy in 2019. According to The Quantum Insider, Google’s head of Quantum AI, Hartmut Neven, recently predicted that commercial quantum computing applications will be realized within five years.
- IBM Quantum: IBM continues to expand its quantum computing capabilities through its IBM Q Experience platform, making quantum resources accessible to researchers and developers worldwide.
- Microsoft Quantum: Microsoft is pursuing topological qubits, which promise greater stability and error resistance than other approaches.
- Quantinuum: Formed from the merger of Honeywell Quantum Solutions and Cambridge Quantum Computing, Quantinuum is advancing both hardware and software solutions for Quantum AI.
- D-Wave Systems: A pioneer in quantum annealing technology, D-Wave focuses on optimization problems and machine learning applications.
Recent breakthroughs include improvements in quantum error correction, increased qubit coherence times, and demonstrations of quantum advantage for specific machine learning tasks. The Quantum Insider’s 2025 outlook provides an in-depth analysis of these advancements and their implications.
Available Quantum Hardware and Platforms
Quantum computing hardware continues to advance, though we’re still in the NISQ era characterized by limited qubit counts and relatively high error rates. Current quantum hardware platforms include:
- Superconducting qubits: Used by IBM, Google, and Rigetti Computing, these systems operate at extremely low temperatures.
- Trapped ions: Employed by IonQ and Quantinuum, offering high coherence times and connectivity.
- Photonic quantum computers: Developed by companies like Xanadu and PsiQuantum, using photons as qubits.
- Neutral atoms: Utilized by QuEra and Pasqal, offering scalable architectures with many qubits.
Cloud-based access to quantum computers has democratized research, with platforms like IBM Quantum Experience, Amazon Braket, and Microsoft Azure Quantum providing developers with quantum resources. IEEE Computer Society’s assessment of the current state of quantum computing provides a detailed overview of these platforms and their capabilities.
Research Trends and Investments
Investment in Quantum AI continues to grow, with governments and private investors pouring billions into research and development. The U.S., China, EU, and other major economies have established national quantum initiatives with substantial funding.
Current research trends include:
- Developing more robust error correction techniques
- Creating quantum-specific machine learning algorithms
- Building hybrid quantum-classical systems for practical applications
- Improving quantum hardware scalability and reliability
- Exploring quantum advantage for specific AI tasks
A recent analysis by Boston Consulting Group highlights that despite some near-term challenges, the long-term forecast for quantum computing remains bright, with McKinsey estimating that by 2030, quantum computing could create \$450-850 billion in value, with a significant portion coming from Quantum AI applications.
Challenges and Limitations of Quantum AI
Despite its enormous potential, Quantum AI faces several significant challenges that must be overcome before it can achieve widespread practical implementation.
Hardware Constraints and Quantum Decoherence
One of the most fundamental challenges in quantum computing is maintaining quantum coherence—the delicate quantum states that give quantum computers their power. Quantum systems are extremely sensitive to environmental disturbances, leading to decoherence and errors in computation.
Current quantum computers require specialized environments with extremely low temperatures (near absolute zero for superconducting systems) and electromagnetic shielding. These requirements make quantum hardware expensive to build and operate.
Additionally, today’s quantum computers have relatively few qubits (typically under 1,000), and many have high error rates that limit the complexity of algorithms they can run. Data Center Frontier’s analysis of quantum computing advancements notes that developing error correction techniques and more stable qubits remains a critical research focus.
Algorithm Development Challenges
Creating effective quantum algorithms for AI applications presents another significant challenge. Quantum algorithms must be specifically designed to leverage quantum properties and often require fundamentally different approaches than classical algorithms.
Many quantum machine learning algorithms face issues like “barren plateaus,” where the optimization landscape becomes flat, making it difficult to train models effectively. A study published in Nature Communications revealed that quantum variational algorithms are often “swamped with traps,” highlighting the difficulties in developing effective training methods for quantum neural networks.
Integration with Classical Systems
For the foreseeable future, Quantum AI will need to work alongside classical computing systems in hybrid architectures. This integration presents challenges in data transfer, synchronization, and workflow management between quantum and classical components.
Efficiently encoding classical data into quantum states (and vice versa) can be resource-intensive and potentially negate quantum advantages if not handled properly. Experts on Quora have discussed these integration challenges in detail, noting that developing seamless interfaces between quantum and classical systems is essential for practical Quantum AI applications.
Talent and Knowledge Gaps
The interdisciplinary nature of Quantum AI—spanning quantum physics, computer science, and machine learning—creates significant talent and knowledge gaps. There’s a shortage of professionals with expertise across these domains, limiting the pace of research and development.
Educational institutions and companies are working to address this challenge through specialized quantum computing and Quantum AI programs, but building a robust talent pipeline will take time. The Quantum Insider’s analysis of Quantum AI challenges highlights this talent gap as a significant barrier to faster progress in the field.
The Future of Quantum AI
As we look beyond 2025, what does the future hold for Quantum AI? While predictions in such a rapidly evolving field are inherently uncertain, several trends and milestones are beginning to emerge.
Projected Timeline for Practical Applications
Industry experts and researchers suggest the following timeline for Quantum AI development:
- 2025-2027: Early commercial applications in specific domains like materials science and optimization problems. These will likely use hybrid quantum-classical approaches on NISQ devices.
- 2027-2030: More robust quantum advantage demonstrations for machine learning tasks, with initial deployment in industries like pharmaceuticals, finance, and logistics.
- 2030-2035: Development of error-corrected quantum computers with thousands of logical qubits, enabling more sophisticated Quantum AI applications.
- Beyond 2035: Potential for fault-tolerant quantum computers with millions of qubits, supporting fully quantum neural networks and transformative AI capabilities.
This timeline aligns with projections from major players like Google, whose Quantum AI head predicts significant commercial applications within five years, according to The Quantum Insider’s interview with Hartmut Neven.
Potential Breakthroughs on the Horizon
Several potential breakthroughs could accelerate Quantum AI development:
- Quantum error correction: Advances in error correction could dramatically improve the reliability and capabilities of quantum computers.
- Room-temperature quantum computing: New qubit technologies that operate at or near room temperature would significantly reduce hardware costs and complexity.
- Quantum-specific AI architectures: Novel algorithms designed specifically for quantum systems rather than adaptations of classical approaches.
- Quantum memory and networking: Enhanced capabilities for storing and transmitting quantum information could enable more powerful distributed Quantum AI systems.
PYMNTS’ analysis of key quantum developments highlights how these breakthroughs would address many of the current limitations facing Quantum AI and potentially accelerate its practical implementation.
How Businesses Can Prepare for Quantum AI
While widespread Quantum AI adoption may still be years away, forward-thinking businesses can take steps now to prepare:
- Education and awareness: Build understanding of quantum computing principles and potential applications among technical teams and leadership.
- Identify potential use cases: Assess specific business problems that might benefit from quantum approaches, particularly in optimization, simulation, and machine learning.
- Experiment with quantum platforms: Utilize cloud-based quantum computing services to begin exploring and testing quantum algorithms.
- Develop quantum-ready data strategies: Ensure data architectures can support future quantum applications.
- Form strategic partnerships: Collaborate with quantum computing providers, research institutions, and industry consortia to stay at the forefront of developments.
According to a recent Forbes Technology Council article, businesses that take these steps can position themselves to leverage Quantum AI advantages as the technology matures, potentially gaining significant competitive advantages.
Conclusion: The Quantum AI Revolution
Quantum Artificial Intelligence represents one of the most promising technological frontiers of our time. By combining the computational power of quantum computing with the pattern recognition and learning capabilities of AI, Quantum AI has the potential to solve problems that have long been considered intractable.
While significant challenges remain—from hardware limitations to algorithm development—the pace of progress is accelerating. Major technology companies, governments, and research institutions are investing heavily in Quantum AI, recognizing its transformative potential across industries.
As we move through 2025 and beyond, we can expect to see increasingly practical applications of Quantum AI emerging in fields like drug discovery, financial modeling, materials science, and logistics optimization. These early applications will likely use hybrid quantum-classical approaches, gradually transitioning to more fully quantum solutions as the technology matures.
For businesses and individuals alike, now is the time to begin understanding and preparing for the Quantum AI revolution. Those who develop quantum literacy and identify potential applications early will be best positioned to harness the power of this transformative technology.
Have you started exploring how Quantum AI might impact your industry or organization? What potential applications do you find most exciting? Share your thoughts in the comments below!