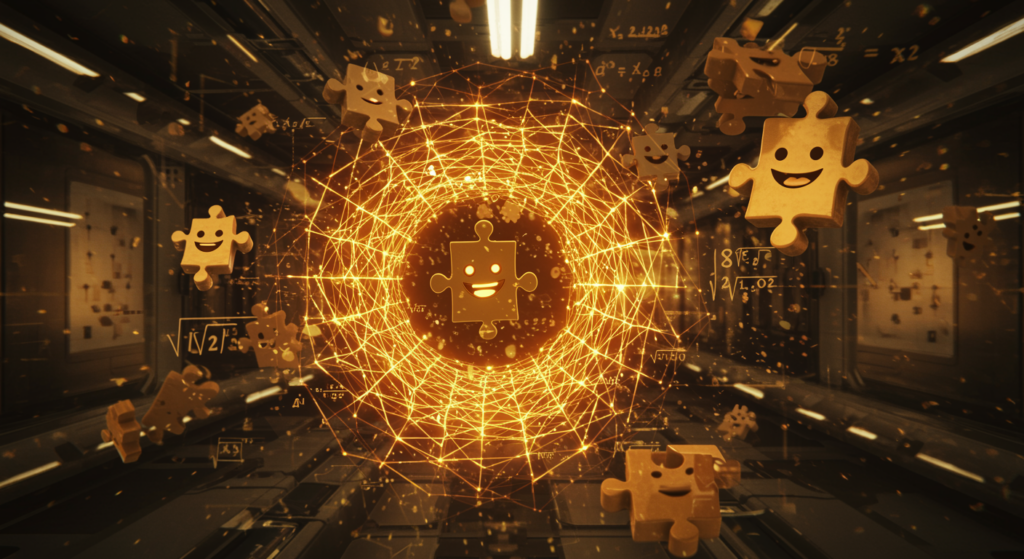
Quantum-Neural Hybrid Breakthrough Solves Impossible Math Problems
Revolutionizing Impossible Problem Solving
Have you ever wondered how quantum-neural hybrid technology could turn today’s toughest math challenges into tomorrow’s routine solutions? This innovative fusion of quantum mechanics and artificial intelligence is making waves, thanks to researchers at Washington University in St. Louis. The cutting-edge system, NeuroSA, harnesses quantum tunneling to deliver optimal results in complex optimization tasks, far surpassing the constraints of traditional AI or quantum methods alone[1]. By integrating these worlds, we’re not just solving problems—we’re redefining what’s possible in fields like logistics and scientific research.
Imagine trying to navigate a maze where classical paths lead to dead ends, but quantum-neural hybrid systems can quantum-leap through barriers. This approach guarantees better outcomes by exploring vast solution spaces more efficiently, offering a glimpse into a future where “impossible” problems become manageable.
The Quantum-Neural Hybrid Approach: NeuroSA Explained
Traditional neural networks often hit roadblocks, like getting stuck in local minima, which means they settle for good-but-not-great solutions. Enter NeuroSA, a quantum-neural hybrid marvel that uses Fowler-Nordheim (FN) annealers to break free from these traps through quantum tunneling[1]. What makes this hybrid so exciting is its ability to systematically scan the entire landscape of possibilities, ensuring the system doesn’t give up too soon.
- Quantum tunneling acts like a secret passage, allowing the system to bypass energy barriers and uncover hidden paths for optimal exploration.
- FN annealers keep the process dynamic, preventing premature conclusions and pushing toward true excellence.
- This quantum-neural hybrid setup even provides mathematical assurances of finding the best answer, as long as we give it the time it needs—not just a quick fix, but a guaranteed win.
Immediate Applications of Quantum-Neural Hybrids
So, how does this quantum-neural hybrid innovation play out in real life? In logistics, it could streamline routing and scheduling, cutting down delays and costs for global supply chains. Drug development benefits hugely too, as modeling molecular interactions at scale speeds up discoveries that save lives. And for scientific research, it’s like having a key to unlock age-old mathematical puzzles that have stumped experts for years.
- Logistics: Picture optimizing delivery routes in real-time, avoiding traffic jams and reducing emissions—now that’s efficiency in action.
- Drug development: Researchers can simulate complex protein folding, accelerating the hunt for new treatments and making healthcare breakthroughs more attainable.
- Scientific research: Tackle problems in physics or engineering that demand precision, turning theoretical ideas into practical realities.
What if we applied this quantum-neural hybrid tech to your daily challenges? It might sound futuristic, but it’s already here, offering tools that could transform how we approach optimization.
Quantum-Classical Synergy: Why Quantum-Neural Hybrids Matter
The magic of quantum-neural hybrids lies in their blend of quantum and classical strengths, creating a powerhouse for handling intricate tasks with speed and accuracy. Quantum elements tackle the heavy lifting of complex calculations, while classical systems handle the nuts and bolts of data management, resulting in a seamless partnership that boosts overall efficiency[4][2][3].
- Quantum computers shine in simulating quantum systems and scaling up optimization efforts.
- Classical computers excel at organizing data and making sense of results, ensuring the hybrid runs smoothly.
- Together, they overcome the limitations of going solo, opening doors to innovations in areas where traditional methods fall short.
Generalization in Quantum-Neural Hybrid Models
One key advantage of quantum-neural hybrids is their strong generalization ability—how well they adapt to new, unfamiliar data. Recent studies have developed frameworks to measure this reliability, which is crucial for using these systems in unpredictable real-world scenarios[2]. Think of it as training a model that not only learns from today’s data but thrives on tomorrow’s surprises, making quantum-neural hybrid tech more dependable than ever.
Could this mean more robust AI for everything from weather forecasting to financial modeling? Absolutely, and it’s a game-changer for industries relying on accurate predictions.
Breaking the ‘Impossible’ Barrier with Quantum-Neural Hybrids
Quantum-neural hybrids are proving their worth by cracking problems long considered unsolvable, such as Euler’s officer problem, which baffled mathematicians for centuries. By leveraging quantum entanglement, these systems explore multiple solutions simultaneously, shattering classical constraints and revealing answers that were once out of reach[6].
Case Study: Solving Euler’s Officer Problem with Quantum-Neural Tech
Take Euler’s officer problem, for example—a classic puzzle where arranging officers under specific rules seemed impossible using traditional methods. With quantum-neural hybrids, entanglement lets elements coexist in various states at once, effectively bypassing the roadblocks that frustrated experts for over 200 years. This isn’t just a win for math; it’s a testament to how quantum-neural approaches can redefine problem-solving in fields like cryptography and beyond.
Ever faced a puzzle that felt unbeatable? Imagine having a tool that could untangle it effortlessly—what would that change for you?
Technical Innovations Driving Quantum-Neural Hybrid Breakthroughs
Behind the scenes, several advancements fuel the success of quantum-neural hybrids. Quantum annealing minimizes complex functions by exploiting quantum effects, while equivariant quantum neural architectures incorporate problem symmetries for better efficiency and generalization[8]. Then there’s geometric deep learning, which applies spatial insights to quantum data, proving invaluable for tasks like 3D protein modeling in quantum chemistry[5][7].
- Quantum annealing: It fine-tunes objectives with quantum precision, speeding up processes that classical systems struggle with.
- Equivariant quantum neural architectures: These designs ensure models adapt intelligently, enhancing performance in symmetric problems.
- Geometric deep learning: By integrating spatial data, it opens new avenues in areas like material science and drug design.
Feature | Classical Neural Networks | Quantum-Neural Hybrids |
---|---|---|
Optimization Capacity | Can get stuck in local minima | Escapes local minima using quantum tunneling |
Generalization | Limited by classical statistics | Enhanced by quantum-enabled exploration |
Guaranteed Solutions | No mathematical guarantee | Provable optimality (with time) |
Computational Speed | Limited by Moore’s Law | Potential for exponential speedup |
Problem Domains | General ML/AI, specific tasks | Intractable optimization, quantum simulation, combinatorics |
This comparison highlights why quantum-neural hybrids are stepping up where classical methods lag, offering a fresh toolkit for innovators.
Impact on Computing and Research
The rise of quantum-neural hybrids is rippling through industries, enhancing optimization in finance, logistics, and pharmaceuticals. These systems deliver solutions where classical approaches stall, boosting AI’s ability to handle complex patterns with greater accuracy[4]. Plus, they’re pushing the envelope in mathematics, making once-elusive proofs achievable and expanding our knowledge base.
- Better optimization: From stock trading algorithms to supply chain logistics, quantum-neural hybrids cut through complexity with ease.
- Advances in AI: Expect smarter predictions in healthcare, where early disease detection could become the norm.
- New mathematics frontiers: Researchers are now exploring uncharted territories, like advanced cryptography, with tools that were unimaginable just years ago.
Future Prospects: The Dawn of Quantum-Enhanced AI
As quantum-neural hybrid technologies evolve, we’re on the cusp of scalable systems that tackle real-world issues in logistics, healthcare, and security. Wider adoption of hybrid algorithms is on the horizon, promising faster discoveries in science, from unbreakable encryption to innovative materials[2]. This could mean shorter development cycles, giving us solutions to global challenges sooner than expected.
- Scalable quantum AI: Imagine hospitals using these systems for personalized medicine, tailoring treatments based on intricate patient data.
- Broader use of hybrid algorithms in enterprises, driving efficiency and innovation.
- Accelerated scientific progress, where fields like quantum chemistry lead to sustainable energy breakthroughs.
Conclusion: A New Era of Mathematical Discovery
The quantum-neural hybrid breakthrough is more than a technological leap—it’s a gateway to solving impossible math problems and beyond. By merging quantum tunneling with sophisticated neural designs, we’re unlocking potentials that could reshape industries and spark new scientific adventures. As this field grows, consider how it might influence your world, and let’s keep the conversation going.
What are your thoughts on quantum-neural hybrids? Share your ideas in the comments, explore more on our site, or spread the word—together, we can dive deeper into this exciting frontier.
References
- [1] ScienceBlog. “Quantum-Neural Hybrid Solves Impossible Math.” Link
- [2] Quantum Zeitgeist. “Novel Bounds for Generalization in Hybrid Quantum-Classical Machine Learning.” Link
- [3] MDPI. “Applied Sciences Journal.” Link
- [4] Quantinuum. “Quantum Computers Will Make AI Better.” Link
- [5] Quantum Zeitgeist. “Extending K-P Quantum Control with Cartan Decompositions.” Link
- [6] LiveScience. “Math Puzzle Gets Quantum Solution.” Link
- [7] KDD Conference. “2022 Table of Contents.” Link
- [8] APS Physics. “PRX Quantum Article.” Link
quantum-neural hybrid, optimization, quantum tunneling, NeuroSA, impossible math problems, quantum mechanics, artificial intelligence, Fowler-Nordheim annealers, quantum entanglement, hybrid quantum-classical models