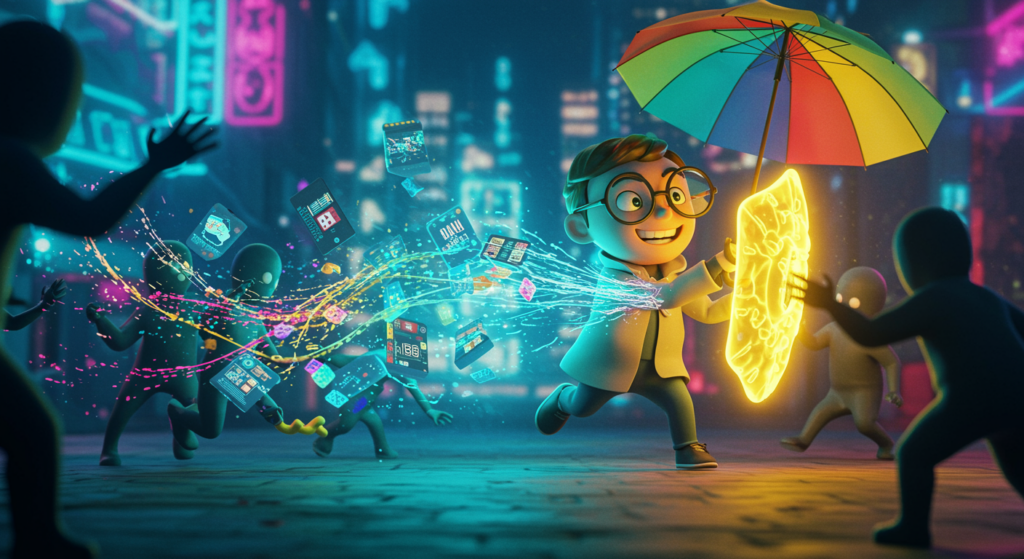
Strengthen AI Cybersecurity: 13 Core Principles for Protection
Why AI Cybersecurity Matters in Today’s Digital Landscape
As artificial intelligence weaves into the fabric of everyday business and personal life, AI cybersecurity has become a critical shield against increasingly clever cyber threats. Think about it: from healthcare diagnostics to financial predictions, AI systems handle sensitive data that attackers are eager to exploit. In this article, we’ll dive into 13 core principles drawn from global standards, helping you build a fortress around your AI assets to ensure reliability and trust.
The Evolving Challenges of AI Cybersecurity
Securing AI isn’t like locking down a standard app; it involves unique hurdles such as data poisoning, where attackers corrupt training data, or adversarial attacks that trick models into wrong decisions. These issues stem from AI’s reliance on vast datasets and complex algorithms, making a comprehensive, lifecycle-based approach essential. Have you ever wondered how a small tweak in input data could derail an entire AI system? That’s why experts emphasize ongoing vigilance from design to deployment.
What Sets AI Cybersecurity Apart from Traditional Methods
- AI systems deal with dynamic data flows, expanding the attack surface far beyond static networks.
- They depend on opaque algorithms that can be manipulated, unlike conventional software.
- Ultimately, breaches in AI cybersecurity can erode customer trust and disrupt operations in real time.
Overview of the 13 Core Principles for AI Cybersecurity
Based on the ETSI TS 104 223 specification, these 13 principles offer a practical blueprint for enhancing AI cybersecurity. They’re not just theoretical—they’re actionable steps that address everything from data handling to ongoing maintenance. Whether you’re developing AI or managing it, these guidelines can help you create more resilient systems. Let’s break them down to see how they fit into your workflow.
Essential Principles to Bolster Your AI Cybersecurity
-
Data Governance in AI Cybersecurity
Start with solid data governance to manage the information feeding your AI models. This means verifying data quality, tracking its origins, and ensuring privacy to ward off risks like data poisoning. For instance, imagine a retail AI system trained on tampered customer data—suddenly, recommendations go haywire. By implementing strict controls early, you can prevent such scenarios and maintain the integrity of your AI cybersecurity framework.
-
Building Robustness and Resilience for AI Security
Make your AI systems tough against attacks by incorporating adversarial training and regular stress tests. This principle focuses on keeping models reliable even when faced with unexpected inputs. A real-world example: In autonomous vehicles, robustness ensures that slight alterations in road signs don’t lead to accidents, directly tying into effective AI cybersecurity.
-
Ensuring Transparency and Explainability in AI Cybersecurity
Transparency allows you to audit AI decisions, turning black-box models into accountable tools. This not only aids in compliance but also helps in quickly spotting issues during investigations. Picture a loan approval AI—being able to explain its decisions builds user trust and strengthens overall AI cybersecurity.
-
Access Control and Identity Management for Secure AI
Limit who can interact with your AI systems through rigorous identity and access management. Assign granular permissions to reduce unauthorized access risks. In a corporate setting, this could mean only select team members can modify models, a key layer in your AI cybersecurity strategy.
-
Adopting Secure Development Practices in AI
Incorporate security from the ground up by including testing and code reviews in every development phase. Addressing vulnerabilities early prevents them from snowballing into major threats. For developers, this might involve automated scans that catch issues before deployment, enhancing AI cybersecurity.
-
Threat Modeling and Risk Assessment for AI Protection
Proactively identify potential threats by conducting regular risk assessments tailored to your AI systems. As AI evolves, so should your defenses—update these models to stay ahead. This principle is like a security checklist that adapts, ensuring your AI cybersecurity remains dynamic.
-
Continuous Monitoring and Incident Detection in AI Cybersecurity
Set up real-time monitoring to catch anomalies or breaches as they happen, with protocols for swift responses. This ongoing watchfulness is crucial for maintaining operational integrity. Ask yourself: How quickly can your team react to an alert? That’s the essence of robust AI cybersecurity.
-
Prioritizing Privacy and Confidentiality in AI
Protect sensitive data using encryption and techniques like differential privacy, while conducting audits to meet regulations. This not only safeguards information but also bolsters your overall AI cybersecurity posture. In healthcare AI, for example, ensuring patient data confidentiality is non-negotiable.
-
Securing the Supply Chain for AI Cybersecurity
Vet all external components, from APIs to pre-trained models, to avoid introducing hidden vulnerabilities. A single weak link in the chain can compromise everything, so thorough checks are vital. This principle reminds us that AI cybersecurity extends beyond in-house systems.
-
Lifecycle Management in AI Security
Maintain security at every stage, from initial deployment to eventual decommissioning. Proper management ensures that updates don’t create new risks and that data is securely archived. It’s about treating AI cybersecurity as a continuous process, not a one-time fix.
-
Accountability and Auditability for AI Systems
Keep detailed logs of all AI activities to enable traceability and quick incident resolution. This level of accountability is essential for regulatory compliance and internal reviews. In practice, it means having a clear paper trail that supports your AI cybersecurity efforts.
-
Fostering Agility and Adaptability in AI Cybersecurity
Design your security measures to evolve with emerging threats and AI advancements. Encourage a culture of learning and iteration to keep your defenses sharp. This adaptability ensures that your AI cybersecurity strategy doesn’t become outdated.
-
Promoting Awareness and Training for AI Protection
Regular training sessions for your team can highlight new attack vectors and reinforce best practices. Everyone from developers to end-users plays a role in AI cybersecurity. Consider this: A well-informed team is your first line of defense against potential breaches.
Putting These AI Cybersecurity Principles into Practice
To make these principles work, apply them across the entire AI lifecycle: from design to decommissioning. In the design phase, weave in security requirements to avoid future headaches. During development, test rigorously against common threats, and in operations, use monitoring tools to keep everything in check. This holistic approach not only strengthens AI cybersecurity but also makes your systems more reliable overall.
- Design: Build security into the blueprint from day one.
- Development: Test defenses thoroughly to catch vulnerabilities early.
- Deployment: Harden infrastructure before going live.
- Operation and Maintenance: Monitor continuously and update as needed.
- Decommissioning: Safely retire systems and erase sensitive data.
AI Cybersecurity vs. Traditional Cybersecurity: Key Differences
Aspect | Traditional Cybersecurity | AI Cybersecurity |
---|---|---|
Attack Surface | Networks and endpoints | Data sets and algorithms |
Key Threats | Phishing and malware | Adversarial inputs and model theft |
Defensive Focus | Perimeter protection | Model integrity and explainability |
Lifecycle Coverage | Primarily operations | Full lifecycle integration |
Top Strategies for Deploying Secure AI Systems
- Form a team that blends AI expertise with security know-how for comprehensive coverage.
- Adopt flexible frameworks that adapt to AI’s fast pace, ensuring your AI cybersecurity stays effective.
- Regularly reassess risks as new AI applications emerge to keep threats at bay.
- Use established standards like ETSI TS 104 223 for measurable results in AI cybersecurity.
- Focus on clear documentation to build trust with users and regulators alike.
Aligning AI Cybersecurity with Your Business Goals
Effective AI cybersecurity isn’t just about tech—it’s about protecting your brand and growth. Identify your key assets, like customer data, and align security investments to support them. By doing so, you not only comply with regulations but also foster long-term trust. For example, a fintech company enhancing its AI cybersecurity can prevent costly breaches while maintaining client confidence.
The Future of AI Cybersecurity: Standards and Collaboration
Standards like ETSI TS 104 223 are paving the way for safer AI, emphasizing the need for industry-wide cooperation. As threats evolve, working with experts and regulators will help refine these principles. This collaborative spirit ensures that AI cybersecurity advances alongside technology, making systems more secure for everyone.
Wrapping Up: Make AI Cybersecurity Your Foundation
By embracing these 13 principles, you’re not just defending against threats—you’re building a trustworthy AI ecosystem. Remember, strong AI cybersecurity means innovation can thrive without fear. What steps will you take next to protect your systems? Share your thoughts in the comments, explore our related posts on secure tech practices, or connect with us for personalized advice.
References
- ETSI TS 104 223 on Securing AI. (2025). Help Net Security. Source
- AI Cybersecurity Reinvented: 13 Essential Principles. Cyber Experts. Source
- The Fundamentals of Cybersecurity in the Age of AI. Security Magazine. Source
- ITI AI Security Principles. ITI. Source
- AI Security Best Practices. Wiz. Source
- Top 8 AI Security Best Practices. Sysdig. Source
- AI and SEO Insights. Marketing Sherpa. Source
- AI Cybersecurity Blog. CDG. Source
AI cybersecurity, AI security principles, protecting AI systems, secure AI lifecycle, AI threat prevention, AI data protection, robust AI defenses, AI risk management, AI privacy strategies, AI compliance standards